Online Data-Driven Battery Life Prediction and Quick Classification Based on Partial Charging Data Within 10 Min
Journal of power sources(2024)
摘要
Accurate online battery life prediction is critical for the health management of battery powered systems. This study develops a moving window-based method for in-situ battery life prediction and quick classification. Five features are extracted from the partial charging data within 10 min to indicate battery aging evolution. The machine learning techniques are used to connect the features and battery end of life (EOL), with the Gaussian process regression (GPR) and support vector machine (SVM) used to predict and classify battery life, respectively. The performance of the developed methods is validated based on experimental data of 121 battery cells. Results show that GPR predicts accurate battery EOL and knees with the root mean square errors and mean absolute percentage errors being within 100 cycles and 10 %, respectively. SVM classifies battery life quickly and accurately based on only one cycle's data, with the classification accuracy close to 92 %. In summary, the developed methods show comparable in-situ life prediction and classification accuracies to the benchmark that needs offline calibrations.
更多查看译文
关键词
Lithium -ion battery,Remaining useful life,Knee point prediction,Online life classification,Machine learning,Feature extraction
AI 理解论文
溯源树
样例
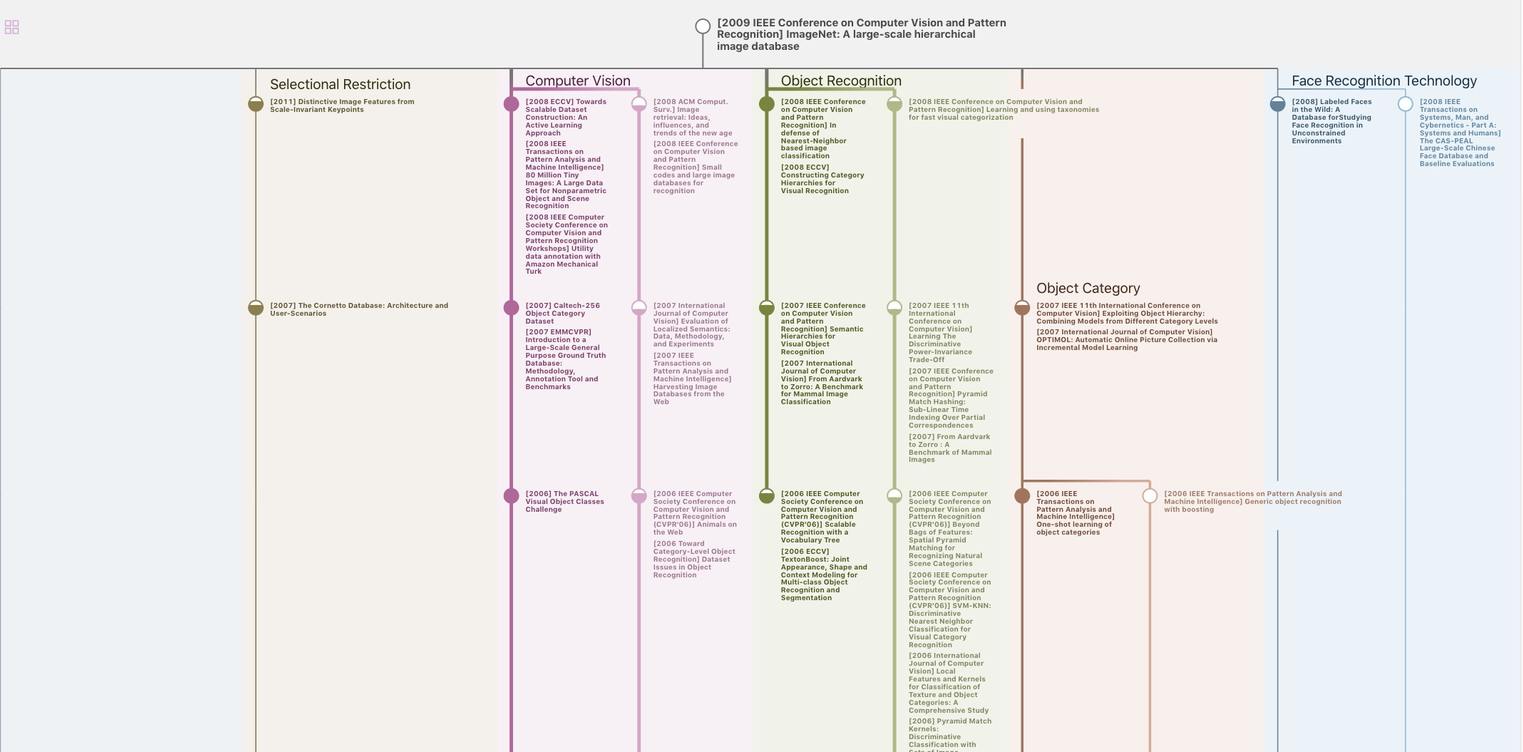
生成溯源树,研究论文发展脉络
Chat Paper
正在生成论文摘要