Action Behavior Learning Based on a New Multi-Scale Interactive Perception Network
IEEE ACCESS(2023)
摘要
Action recognition is a fundamental research topic in the field of video understanding, but classical action recognition relies on a large amount of manually annotated video data, which limits its development. Small sample action recognition is a promising topic that can overcome the dependence on large-scale annotated data. However, the current small sample action recognition has a series of shortcomings such as temporal singularity and a lack of perception of global information. Therefore, this work proposes an interactive perception network by designing a multi-scale temporal feature extraction module to capture global temporal dependencies between all frames and local temporal information between frames. Then the algorithm proposes a cross scale matching strategy to achieve robust matching between videos with different motion speeds, maximizing the consistency between local and global features of the same type of action. Finally, the experimental results on the SSV2 dataset and the HMDB51 dataset show that the proposed method outperforms the current mainstream methods. Compared with the backbone network, which is the most advanced method of ResNet, this method achieved performance improvements of 0.8% and 0.4% on HMDB51 and SSV2, respectively.
更多查看译文
关键词
Action recognition,small sample learning,video understanding,meta learning
AI 理解论文
溯源树
样例
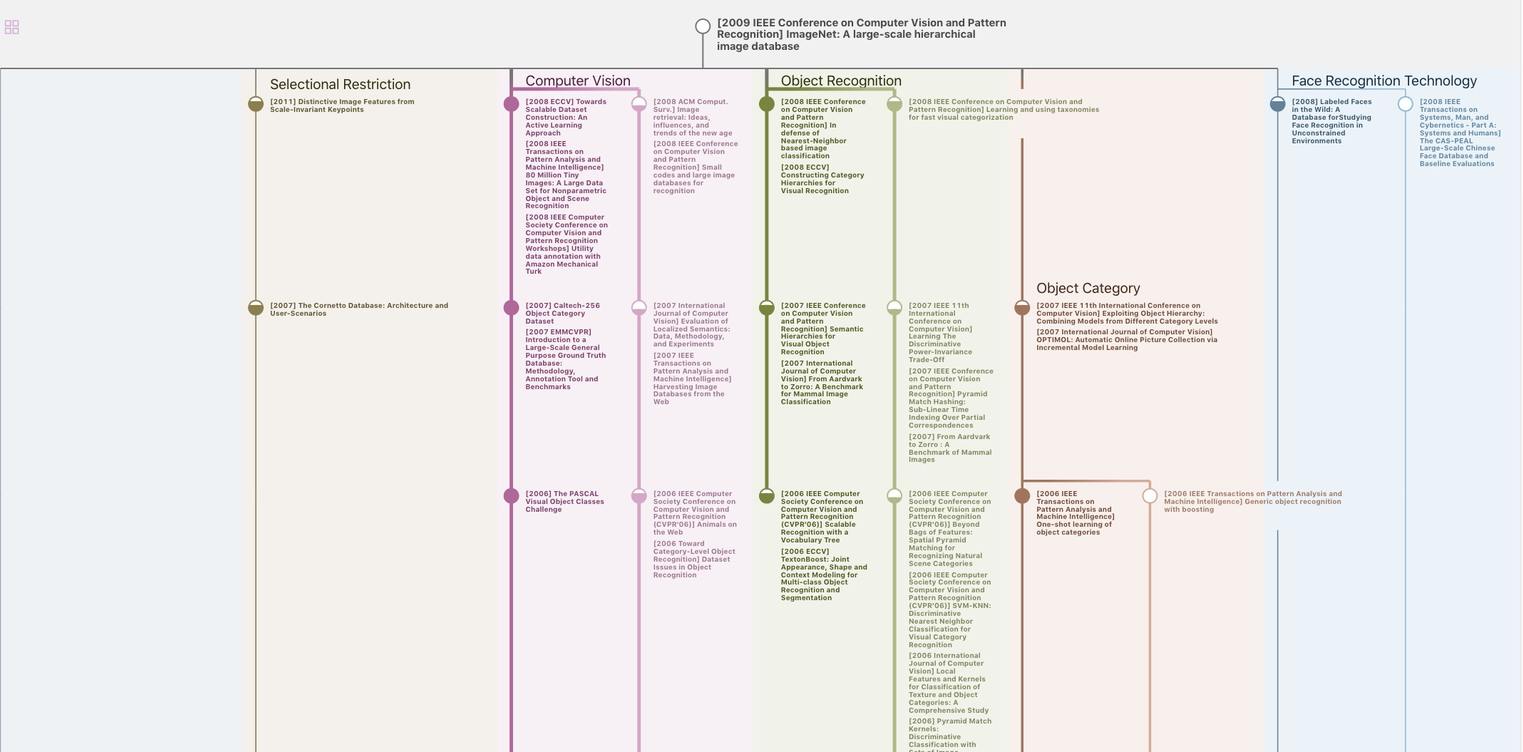
生成溯源树,研究论文发展脉络
Chat Paper
正在生成论文摘要