A Multitask Integrated Deep-Learning Probabilistic Prediction for Load Forecasting
IEEE TRANSACTIONS ON POWER SYSTEMS(2024)
摘要
Spinning reserve without accurate load forecasting can lead to automatic disconnection of critical loads by under-frequency load shedding devices. Such a predicament poses a grave threat to the economic and social welfare of the affected region, and in extreme scenarios, can result in a debilitating grid collapse. However, existing models lack the deep feature extraction capability and cannot accurately predict the uncertainty of electricity demand. Thus, a multitask integrated deep-learning probabilistic prediction with multidimensional feature extraction based on granularity information and quantile regression is explored in this study to solve the insufficient feature extraction problem in probabilistic prediction. In the proposed scheme, we designed a multi-layer feature extraction framework, which information granularity based on fuzzy rough sets to extract time-domain features, multilayer Laplace operators to extract features globally, and quantile regression recurrent neural network variants to extract time context information. In addition, the Pareto integration method is extended to capture better individuals. The experimental results demonstrate that the proposed system can effectively improve deterministic forecasting and uncertainty analysis of electricity demand and manage daily fluctuations.
更多查看译文
关键词
Feature extraction,Load modeling,Biological system modeling,Predictive models,Data mining,Spinning,Probabilistic logic,Spinning reserve,load forecasting,quantile regression,granular computing,feature extraction,multitask integration
AI 理解论文
溯源树
样例
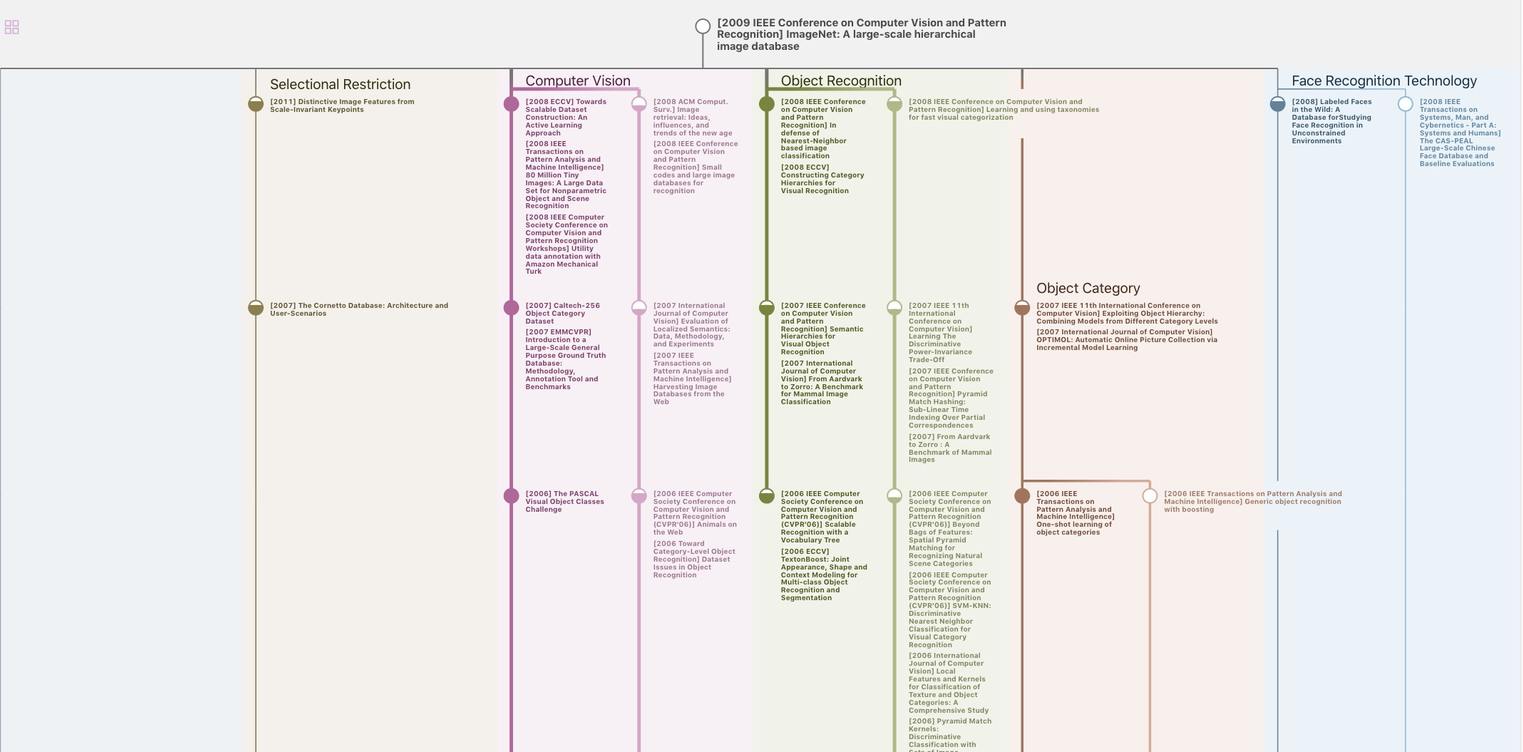
生成溯源树,研究论文发展脉络
Chat Paper
正在生成论文摘要