Assessment of Deep Learning Approaches for the Detection of Cardio-Respiratory Causal Interactions.
2023 Computing in Cardiology (CinC)(2023)
摘要
Granger causality (GC) and transfer entropy (TE) are commonly used methods for studying causality between physiological signals. Recently, neural networks (NN) approaches have been introduced for TE estimation and GC detection. This study compared traditional estimation of TE using adaptive partitioning (DVP), with two NN approaches: neural network GC (nnGC) and neural network TE (nnTE). The comparison was performed based on their ability to detect interactions and their computation times. The study used three simulation models (linear, nonlinear, and linear + nonlinear) and cardio-respiratory data from a polysomnography study of 26 subjects. In the simulations, DVP outperformed the NN-based methods (mean area under the receiving operating curve - AUC: 0.99, computation time: 0.07 s). nnGC performed well (mean AUC: 0.98) but was slower than DVP (167.07 s), while nnTE struggled with the linear and linear + nonlinear models (mean AUC: 0.75 and 0.49, respectively) and was the slowest (943.83 s). In the clinical data, nnGC and nnTE detected interactions on 9 and 10 subjects, respectively, which aligned with the results obtained by DVP (median of 12 subjects). These findings imply that these NN-based methods provide a suitable model-free alternative for studying cardio-respiratory interactions during sleep.
更多查看译文
AI 理解论文
溯源树
样例
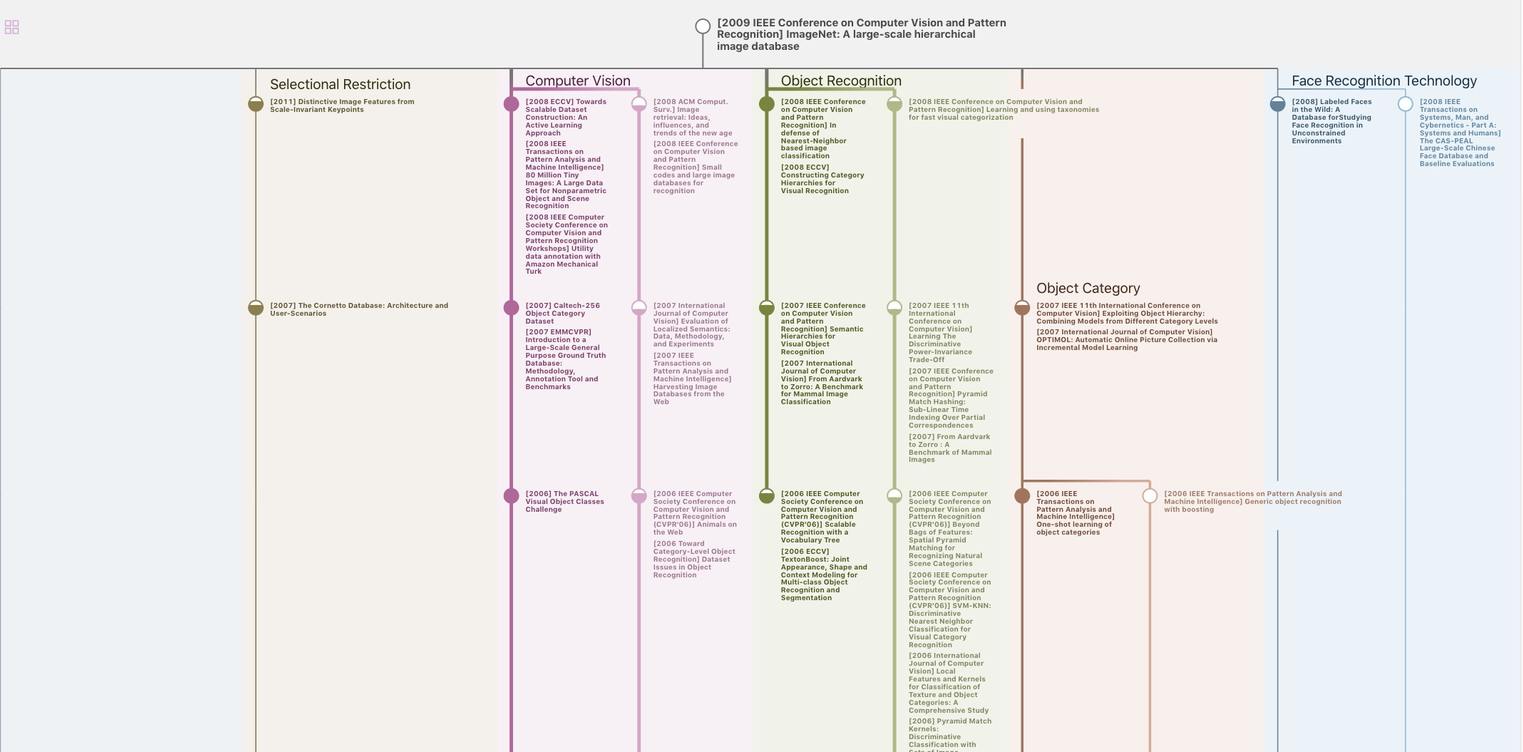
生成溯源树,研究论文发展脉络
Chat Paper
正在生成论文摘要