An Embedding Approach for Biomarker Identification in Hypertrophic Cardiomyopathy.
2023 Computing in Cardiology (CinC)(2023)
摘要
Hypertrophic Cardiomyopathy (HCM) consists of a thickening of the cardiac muscle, causing fatigue, changes in the cardioelectric system, arrhythmias, and even sudden deaths. Variants in gene MYBPC3 are a well-known cause of this illness. Our objective was to find variants in other genes that can cause this pathology. For that purpose, genetic data from a group of patients is analyzed using embedding methods, which allow a lower dimensional representation, which is very helpful for visualization, diagnosis, and personalized therapy. Our results, applying different methods –Principal Component Analyisis (PCA), t-distributed Stochastic Neighbor Embedding (t-SNE), Uniform Manifold Approximation and Projection (UMAP), Orthonormalized Partial Least Squares (OPLS) and Supervised Autoencoders– on genetic data showed a very good separability in the embedded space, allowing us to identify 10 variants that cause that separability. These results may be useful for identifying new HCM cases and implementing new Machine Learning models in those embedded spaces.
更多查看译文
AI 理解论文
溯源树
样例
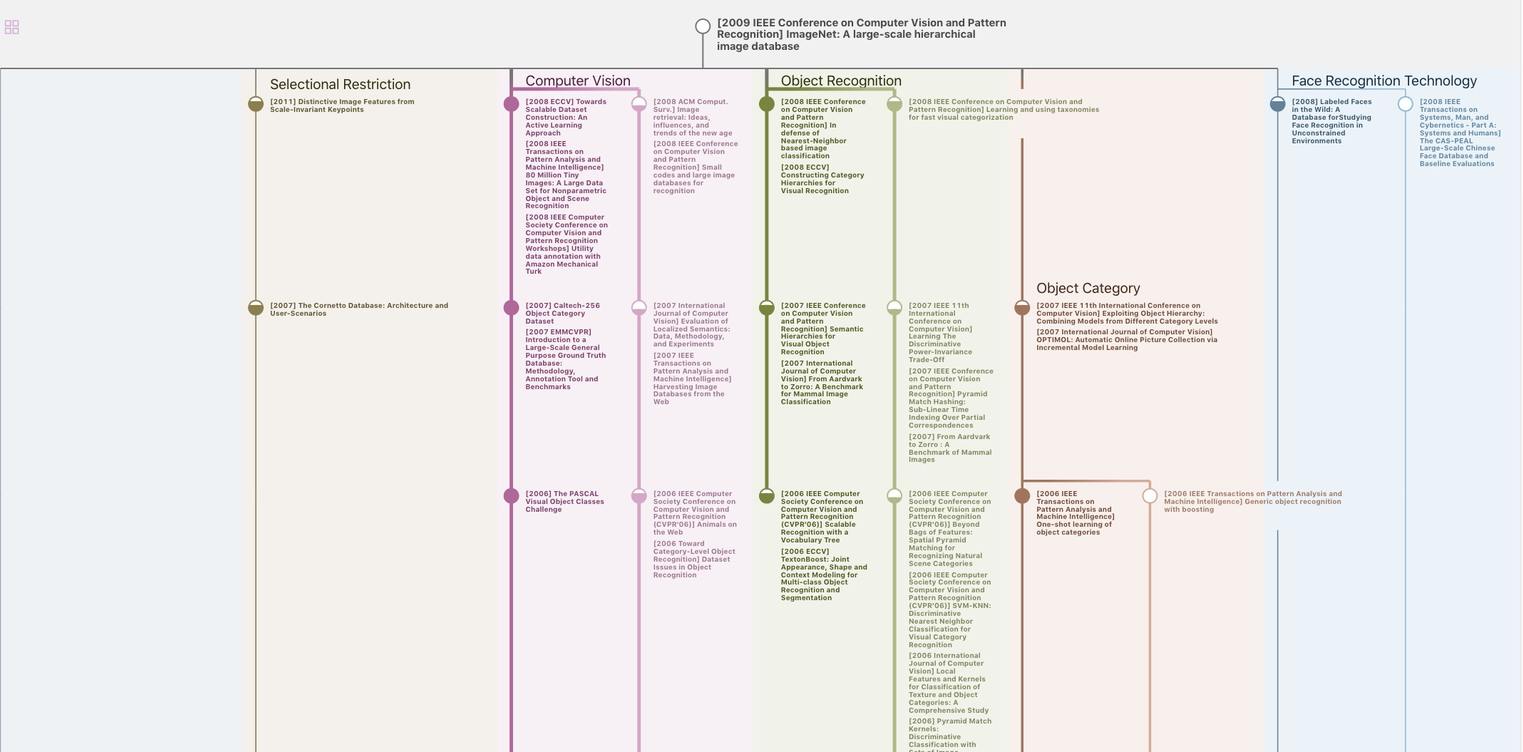
生成溯源树,研究论文发展脉络
Chat Paper
正在生成论文摘要