Less is More: Reducing Overfitting in Deep Learning for EEG Classification.
2023 Computing in Cardiology (CinC)(2023)
摘要
Although most of the patients' recordings includes large scale long-term physiological time series, the patient-level quantity is relatively small, posing great challenges for machine learning methods. As part of the George B. Moody PhysioNet Challenge 2023, we aim to propose a series of Reducing Overfitting techniques in Deep learning for EEG (coined as RODE) in this scenario, for neurological recovery prognosis. RODE is a simple yet effective machine-learning method, which is mostly powered by generalizable deep-learning features. Specifically, we first pre-train a convolutional neural network on the segment level with a margin-based and mining-based loss, and extract deep features from it. Then we decrease the deep features' complexity using dimensionality reduction methods, which prove to be quite significant for reducing overfitting in deep learning. Finally, we combine the reduced features with static features in patient level, and put them into an ensemble model for classification. Our team, PKU_NIHDS, receives a Challenge score of 0.821 on the hidden validation set and 0.708 on the hidden test set.
更多查看译文
AI 理解论文
溯源树
样例
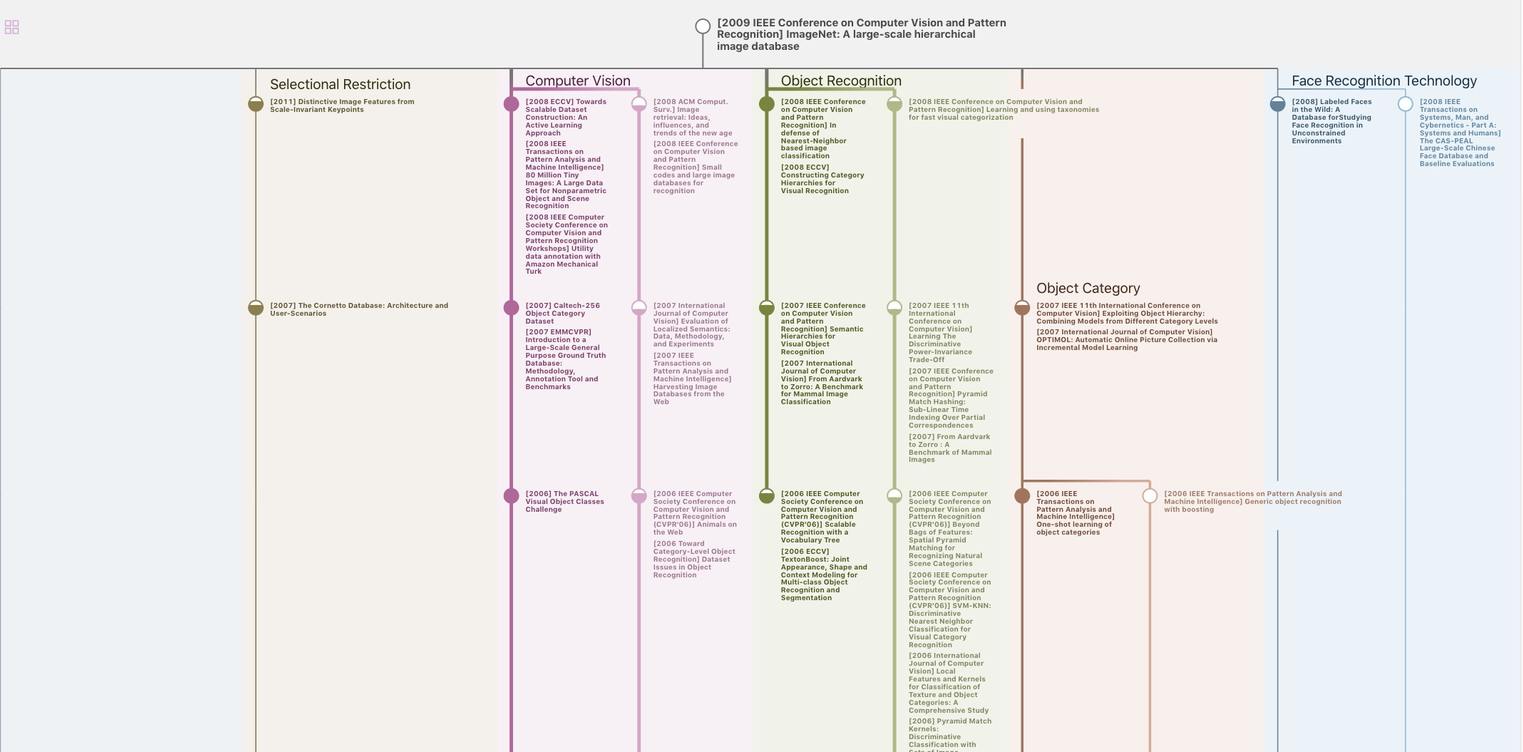
生成溯源树,研究论文发展脉络
Chat Paper
正在生成论文摘要