A Comparative Analysis of Data-Driven Modelling Techniques for 30-Day Heart Failure Readmission Prediction.
2023 Computing in Cardiology (CinC)(2023)
摘要
Unplanned readmissions due to heart failure are a major contributor to the overall annual healthcare costs associated with heart failure. It is anticipated that these costs will increase over 100% by the year 2030. Identifying patients who are at risk of readmission or mortality presents an opportunity to alleviate the burden on hospitals and improve patient outcomes. While there have been numerous studies exploring the use of machine-learning techniques on Electronic Health Records (EHR) data, only a subset of them have considered the temporal aspects of the data, and even fewer have conducted dedicated investigations. In this study, we utilize a dataset of EHR information obtained from a large-scale multi-center healthcare system to assess the effectiveness of extracting trends from time-series medical data using a range of machine-learning methods, spanning various model families. Our investigation incorporates statistical features, such as regression line coefficients and volatility metrics, and reveals notable enhancements in the predictive performance of all tested machine-learning models.
更多查看译文
AI 理解论文
溯源树
样例
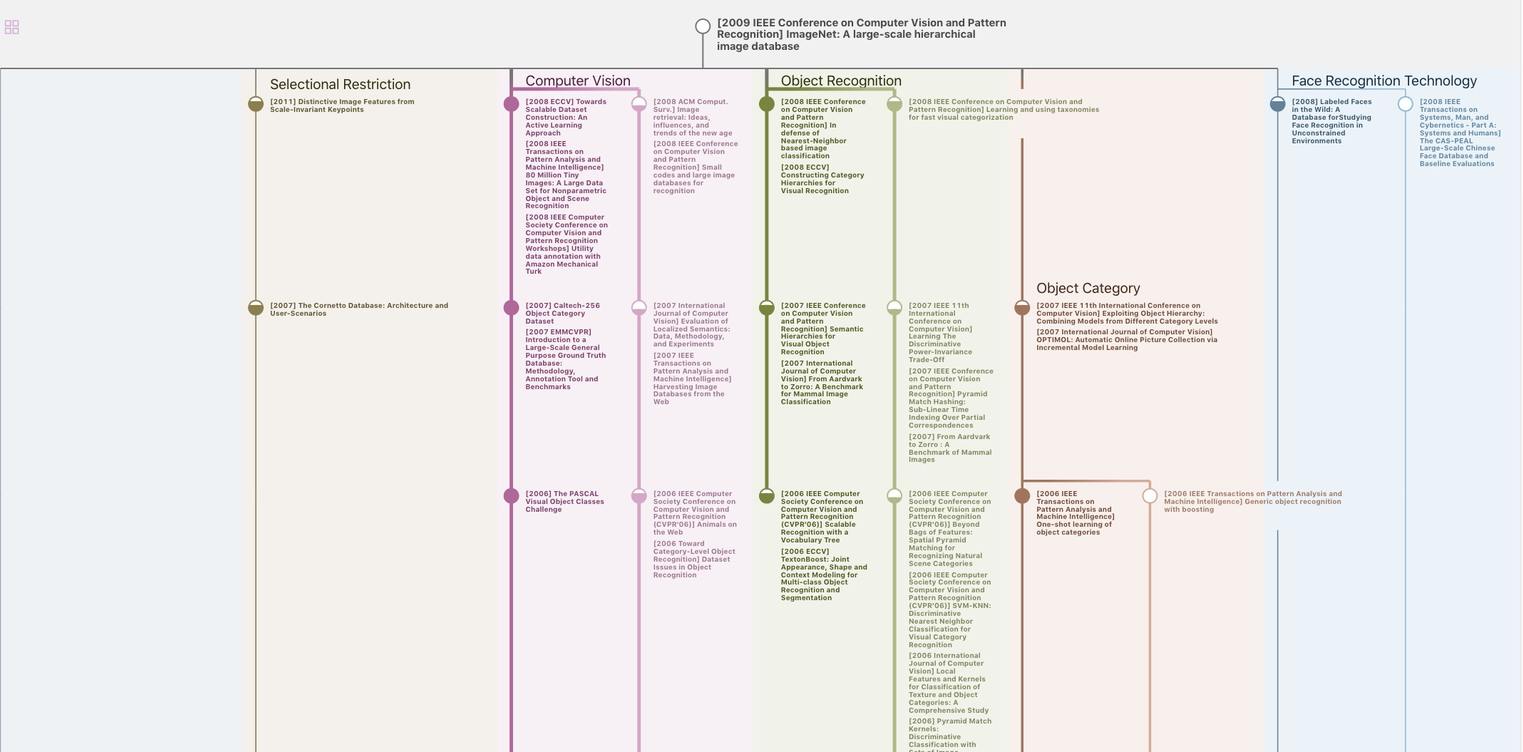
生成溯源树,研究论文发展脉络
Chat Paper
正在生成论文摘要