Combating Coronary Calcium Scoring Bias for Non-gated CT by Semantic Learning on Gated CT
2023 IEEE/CVF INTERNATIONAL CONFERENCE ON COMPUTER VISION WORKSHOPS, ICCVW(2023)
摘要
Coronary calcium scoring (CCS) can be quantified on non-gated or gated computed tomography (CT) for screening cardiovascular disease (CVD). And non-gated CT is used for routine coronary artery calcium (CAC) screening due to its affordability. However, artifacts of non-gated CT imaging, pose a significant challenge for automatic scoring. To combat the scoring bias caused by artifacts, we develop a novel semantic-prompt scoring siamese (SPSS) network for automatic CCS of non-gated CT. In SPSS, we establish a sharing network with regression supervised learning and semantic supervised learning. We train the SPSS by mixing non-gated CT without CAC mask and gated CT with CAC mask. In regression supervised learning, the network is trained to predict the CCS of non-gated CT. To combat the influence of motion artifacts, we introduce semantic supervised learning. We utilize gated CT to train the network to learn more accurate CAC semantic features. By integrating regression supervised learning and semantic supervised learning, the semantic information can prompt the regression supervised learning to accurately predict the CCS of non-gated CT. By conducting extensive experiments on publicly available dataset, we prove that the SPSS can alleviate the potential scoring bias introduced by pixel-wise artifact labels. Moreover, our experimental results show that the SPSS establishes state-of-the-art performance.
更多查看译文
AI 理解论文
溯源树
样例
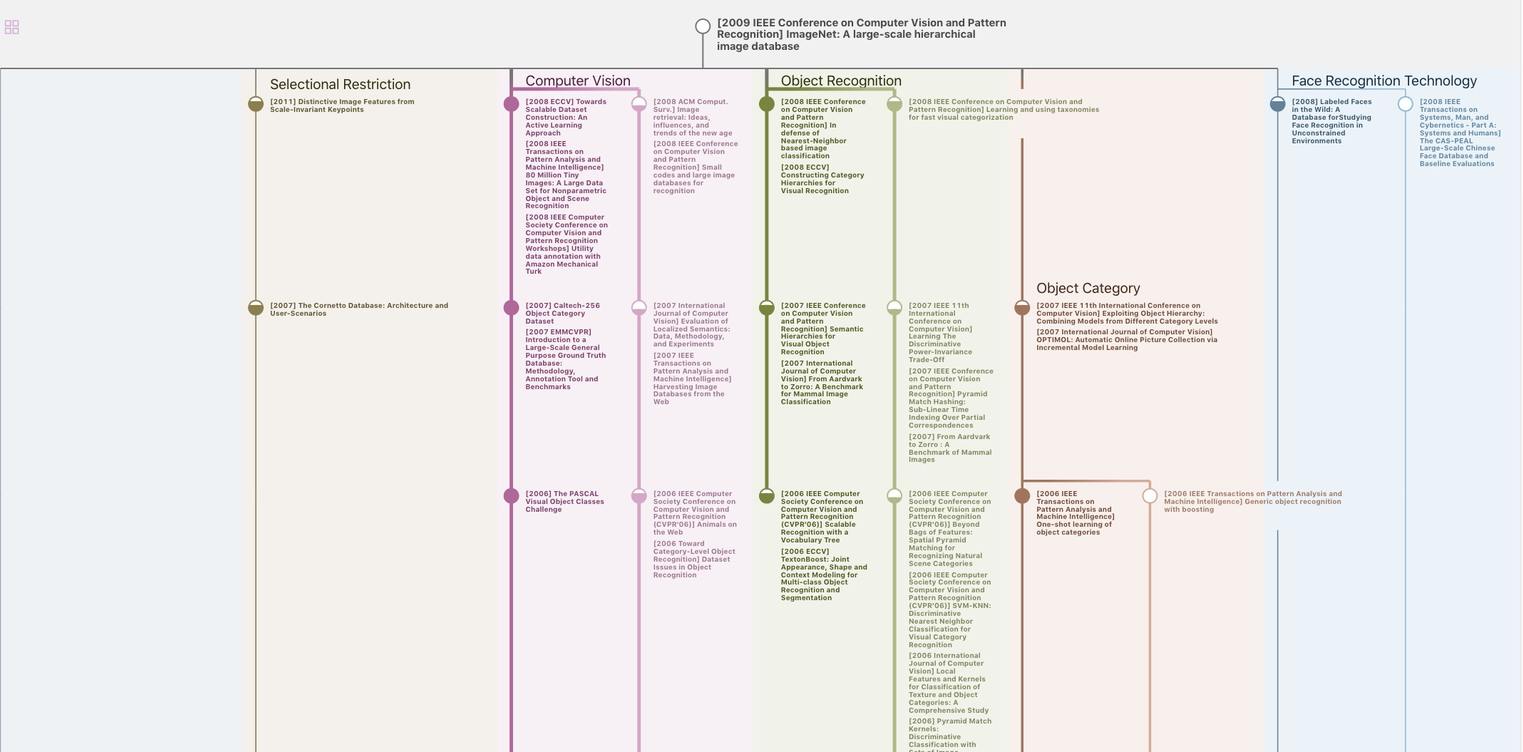
生成溯源树,研究论文发展脉络
Chat Paper
正在生成论文摘要