Time-series Imputation using Graph Neural Networks and Denoising Autoencoders
2023 IEEE 50TH PHOTOVOLTAIC SPECIALISTS CONFERENCE, PVSC(2023)
摘要
Robust system monitoring and prediction relies on having accurate and complete records of system performance. However, real world sensors and monitoring equipment will fail eventually leading to loss of data. This "missingness" effects the stability and accuracy of performance loss rate estimation. To this end, different missingness scenarios were developed to simulate the real world data-stream interruptions. Multiple state of the art and classical imputation methods are bench-marked with different missingness % and missingness type. Accuracy and the precision of the imputation methods was measured against the measured values of AC power from the uncorrupted dataset. It was found that the commonly used interpolation methods such as Linear Interpolation (LI) and Mean interpolation (MI) were significantly outperformed by cutting edge imputation methods such as K-Nearest-Neighbor Imputation (KNN) and a novel graph based neural network imputation (ST-GNN).
更多查看译文
关键词
Graph Neural Network,Imputation,Timeseries Decomposition,Linear Interpolation,Timeseries,Statistical Analysis,Deep Learning
AI 理解论文
溯源树
样例
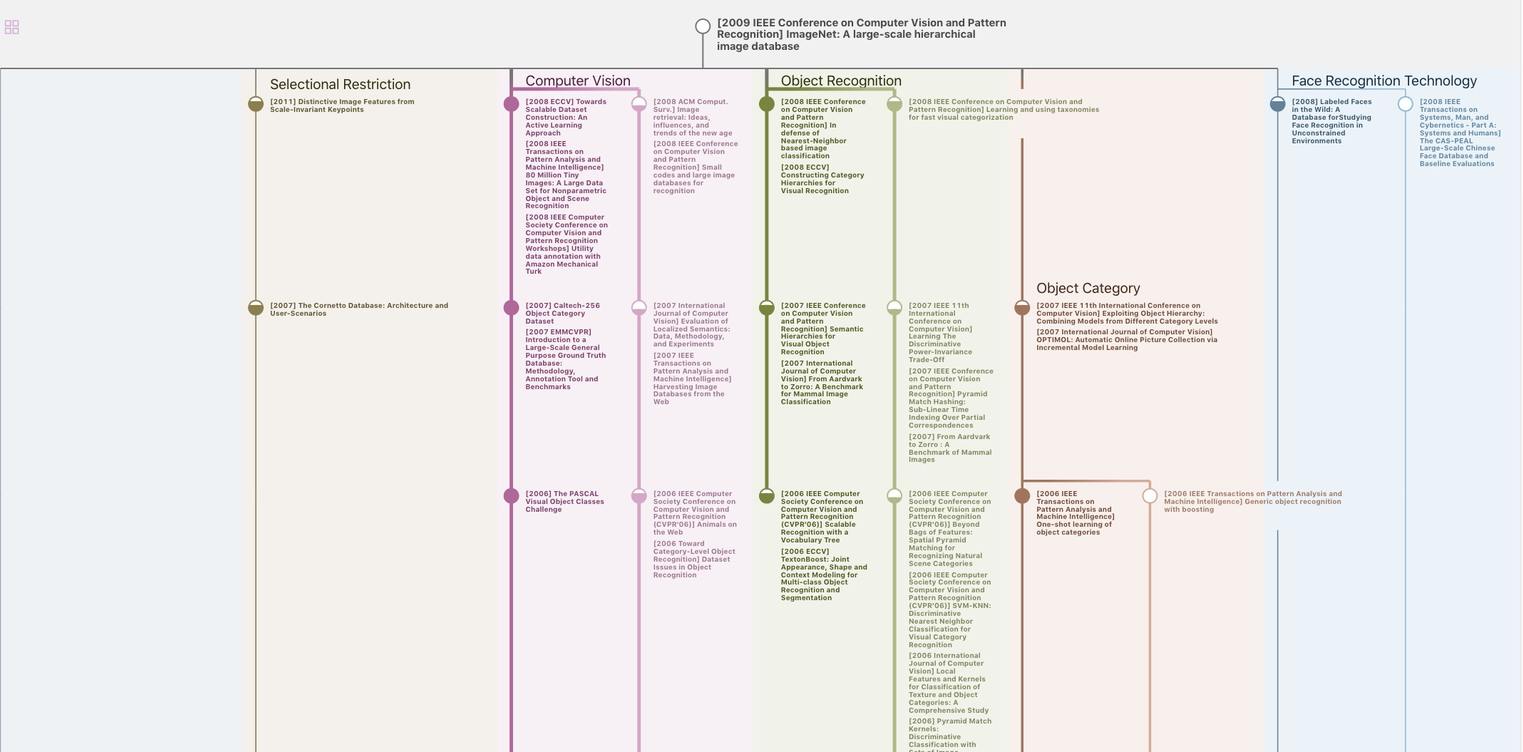
生成溯源树,研究论文发展脉络
Chat Paper
正在生成论文摘要