Recovery from Coma after Cardiac Arrest: Which Time-Window Counts the Most for Deep Learning Predictions?
2023 Computing in Cardiology (CinC)(2023)
摘要
The George B. Moody PhysioNet Challenge 2023 was dedicated to the development of automated methods for predicting neurological recovery from coma after cardiac arrest. Models were requested to predict a good vs poor neurological outcome using electroencephalograms (EEGs), electrocardiograms (ECGs) and clinical information. Here, we proposed a deep learning model based on a residual network architecture. The model was designed to process only one 5-minute window for each hour up to the 72nd from spontaneous resuscitation, and aggregated the output probabilities of poor outcome using different weighted averages. A 5-fold cross validation technique was used to set the hyperparameters of the model and evaluate the performance on the public dataset. The model's input involved EEG data, heart rate variability (HRV) features extracted from the available ECGs and clinical information. The weighted averages showed improvement over uniform weighting on the hidden validation set (score increased up to 20%), but no improvement in cross-validation. Also, the addition of HRV features and clinical information did not show significant improvement over using only EEG data. The Challenge scores on the public training set, hidden validation set and hidden test set were 0.887, 0.627, and 0.708, respectively (team name: unimi_bisp_squad. ranking: 5).
更多查看译文
AI 理解论文
溯源树
样例
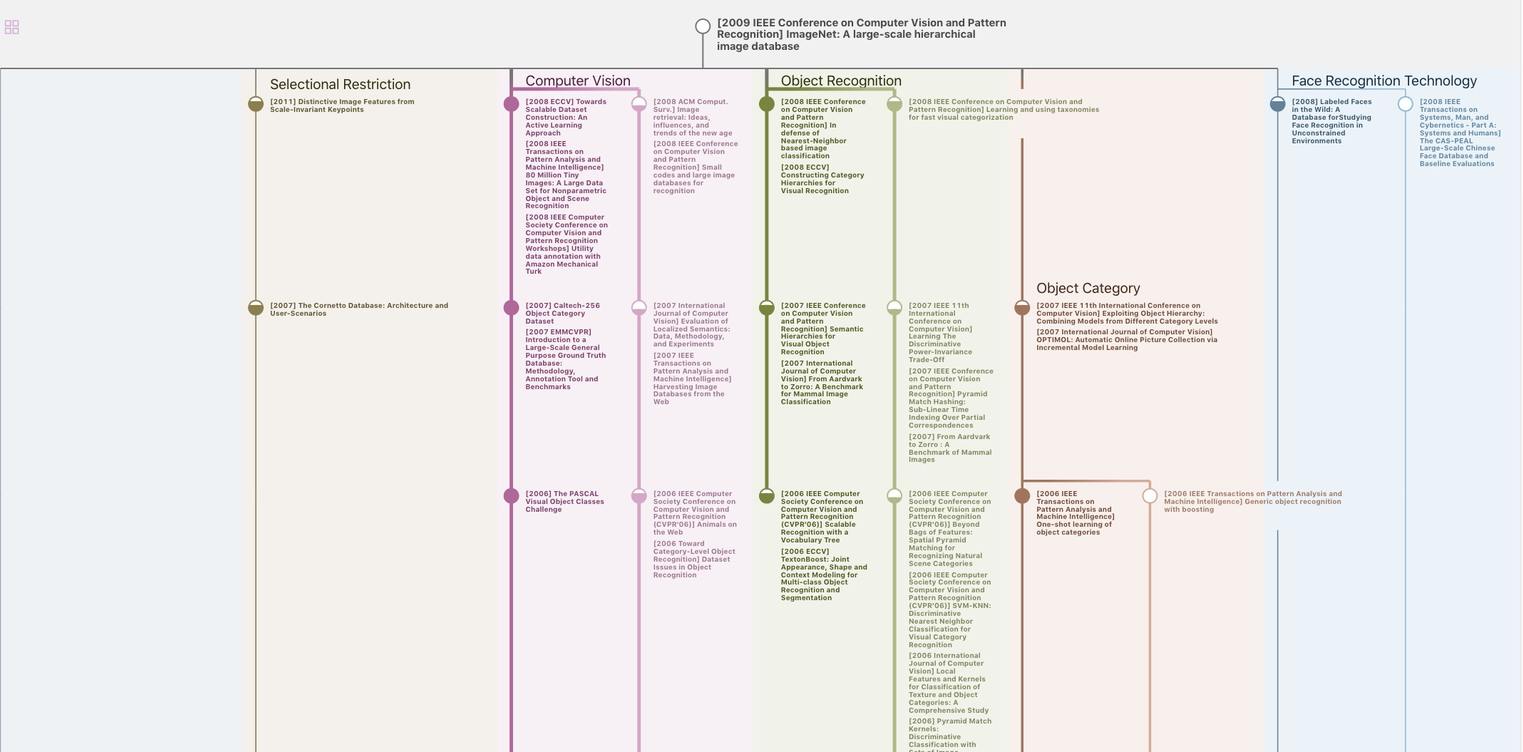
生成溯源树,研究论文发展脉络
Chat Paper
正在生成论文摘要