Threat Modeling for ML-based Topology Prediction in Vehicular Edge Computing Architecture.
2023 IEEE Intl Conf on Dependable, Autonomic and Secure Computing, Intl Conf on Pervasive Intelligence and Computing, Intl Conf on Cloud and Big Data Computing, Intl Conf on Cyber Science and Technology Congress (DASC/PiCom/CBDCom/CyberSciTech)(2023)
摘要
The Internet of Vehicles (IoV), a network that interlinks vehicles, infrastructure, and assorted entities, serves as a cornerstone for intelligent transportation systems and the emergence of smart cities. Within this context, edge computing has been identified as a critical solution for providing rapid and reliable data processing. Machine Learning (ML) techniques have become essential to IoV activities such as resource allocation and load balancing across mobile edge servers, typified by decentralized services that range from natural language processing to image recognition. The fusion of ML with edge computing within IoV architecture promises enhanced performance, efficiency, and safety. However, this amalgamation also creates challenges related to data privacy, cybersecurity, malfunctioning edge devices, inconsistent network connectivity, human errors, and malicious insiders. Consequently, this paper focuses on modeling security threats within an ML-based edge computing framework for the IoV. We analyze the system provided by the Linux Foundation Edge Akraino Project's Stable Topology Prediction blueprint by employing a hybrid threat modeling technique. Our strategy leverages STRIDE to elicit threats on distinct system elements like vehicle-to-vehicle communication networks, edge networks, and ML models. Subsequently, these threats are consolidated for a comprehensive view using an attack tree.
更多查看译文
关键词
Threat Modeling,Machine Learning,Internet of Vehicles,Network Topology,Mobile Edge Computing
AI 理解论文
溯源树
样例
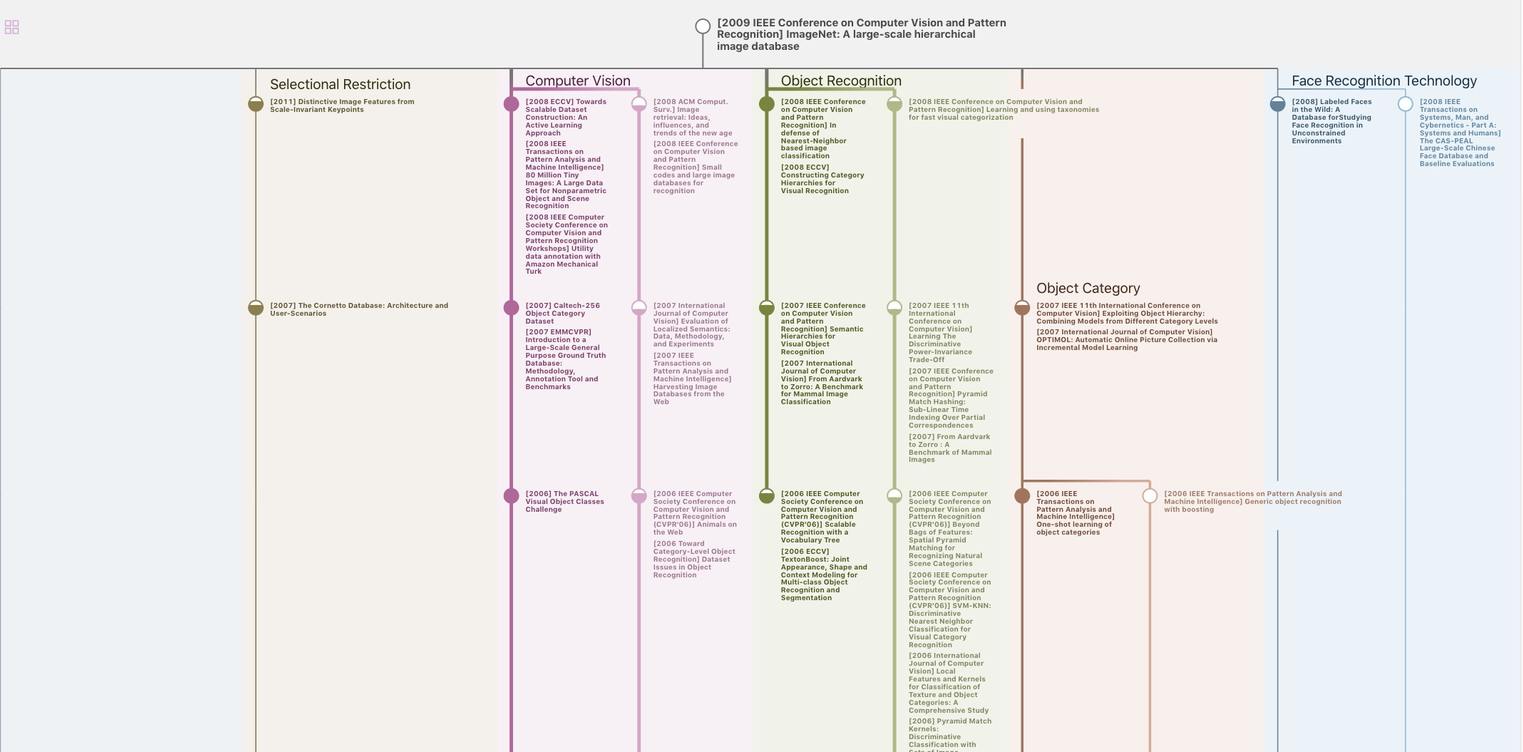
生成溯源树,研究论文发展脉络
Chat Paper
正在生成论文摘要