Machine learning-aided cooling profile prediction in plastic injection molding
The International Journal of Advanced Manufacturing Technology(2024)
摘要
This article discusses a new approach to develop a machine learning model for the cooling profile prediction of plastic parts produced through injection molding to estimate the cooling time. Design parameters related to the cooling stage of injection molding, such as distance between the cooling channel and plastic part, pitch distance, channel diameter, and part thickness, as well as material properties including density, mass, thermal conductivity, and specific heat are considered. A wide range of scenarios is created by considering the design parameters, ensuring a comprehensive analysis. To manage this extensive collection of cases, scripts are used to automate the design and simulation process. The scripts first generate the required cooling channels for each scenario, then simulate the cooling stage of the injection molding process and collect the relevant results. The obtained results are thoroughly analyzed to evaluate the impact of each design parameter on the cooling profile. Then, the physics of the cooling is discussed to estimate the time-dependent temperature of the parts in the plastic injection process. A long short-term memory machine learning model is used to forecast the simulation results, and a regression model is used to predict the cooling profile of the parts. Validation of the regression model is done by calculating the cooling time of an industrial part, produced by plastic injection, and the cooling time of the part is calculated with a 2.67% error.
更多查看译文
关键词
Conformal cooling channels,Plastic injection,Cooling time prediction,Newton's law of cooling,Machine learning
AI 理解论文
溯源树
样例
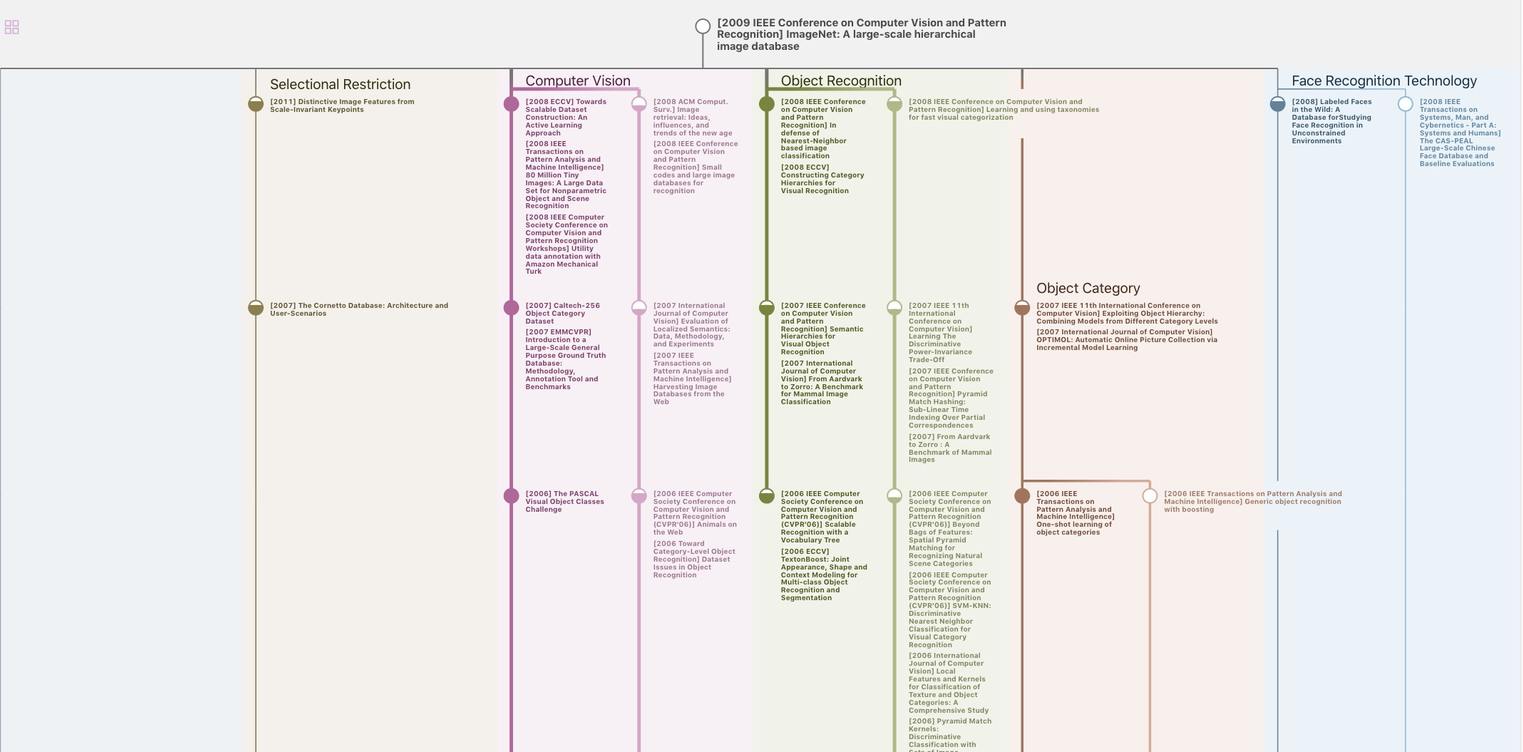
生成溯源树,研究论文发展脉络
Chat Paper
正在生成论文摘要