Early prediction of mortality at sepsis diagnosis time in critically ill patients by using interpretable machine learning
Journal of Clinical Monitoring and Computing(2023)
摘要
This study applied machine learning for the early prediction of 30-day mortality at sepsis diagnosis time in critically ill patients. Retrospective study using data collected from the Medical Information Mart for Intensive Care IV database. The data of the patient cohort was divided on the basis of the year of hospitalization, into training (2008–2013), validation (2014–2016), and testing (2017–2019) datasets. 24,377 patients with the sepsis diagnosis time < 24 h after intensive care unit (ICU) admission were included. A gradient boosting tree-based algorithm (XGBoost) was used for training the machine learning model to predict 30-day mortality at sepsis diagnosis time in critically ill patients. Model performance was measured in both discrimination and calibration aspects. The model was interpreted using the SHapley Additive exPlanations (SHAP) module. The 30-day mortality rate of the testing dataset was 17.9
更多查看译文
关键词
Intensive care,Sepsis,Mortality,Prediction,Machine learning,Shock
AI 理解论文
溯源树
样例
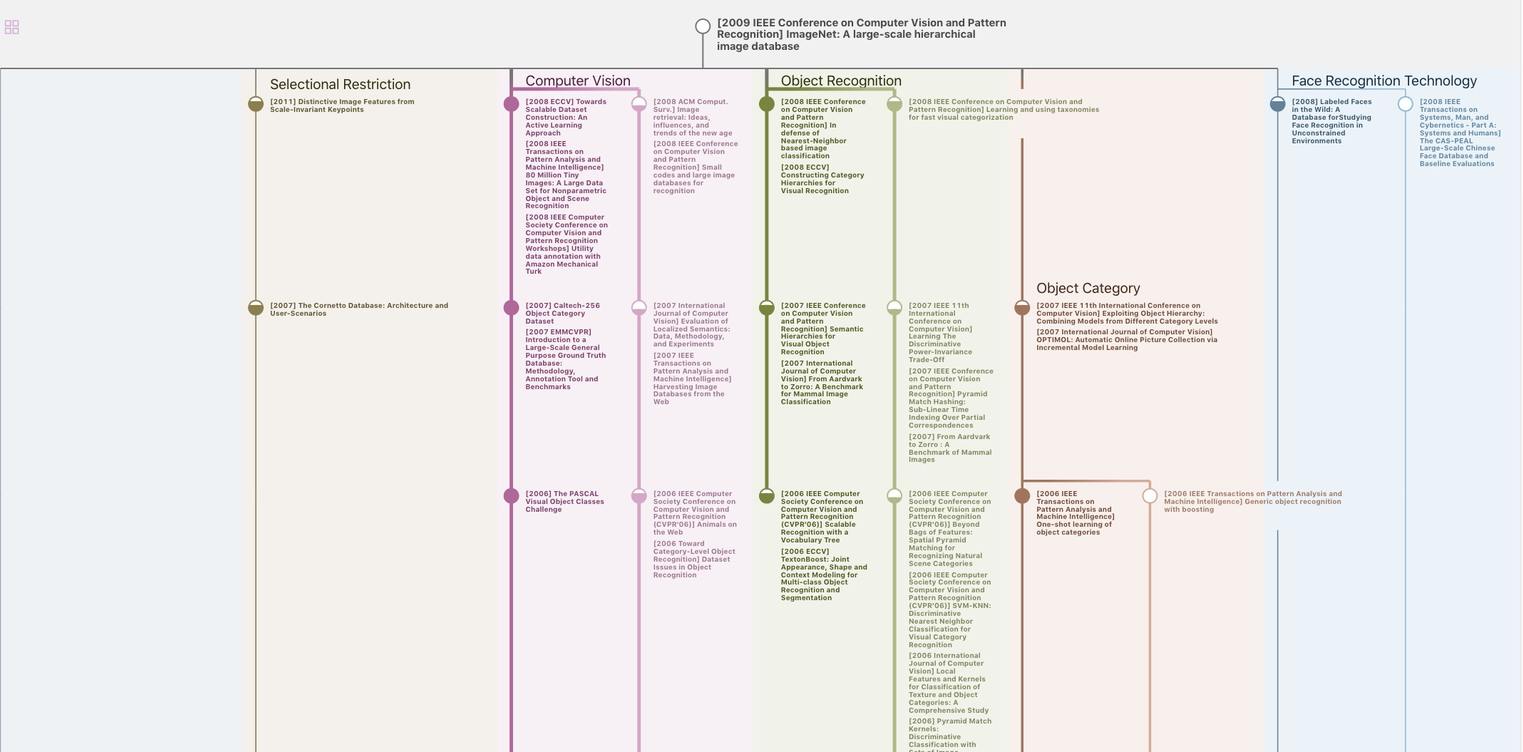
生成溯源树,研究论文发展脉络
Chat Paper
正在生成论文摘要