Effluent quality prediction of the sewage treatment based on a hybrid neural network model: Comparison and application
JOURNAL OF ENVIRONMENTAL MANAGEMENT(2024)
摘要
The accurate prediction and assessment of effluent quality in wastewater treatment plants (WWTPs) are paramount for the efficacy of sewage treatment processes. Neural network models have exhibited promise in enhancing prediction accuracy by simulating and analyzing diverse influent parameters. In this study, a back propagation neural network hybrid model based on a tent chaotic map and sparrow search algorithm (Tent_BP_SSA) was developed to predict the effluent quality of sewage treatment processes. The prediction performance of the propose hybrid model was compared with traditional neural network models using five performance indicators (MAE, RMSE, SSE, MAPE and R2). Specifically, in comparison with the prior Tent_BP_SSA, Tent_BP_SSA2 demonstrated notable enhancements, with the R2 increasing from 0.9512 to 0.9672, while MAE, RMSE, SSE, and MAPE decreased by 9.62%, 18.84%, 24.80%, and 47.10%, respectively. These indicators collectively affirm that the utilization of higher-order input parameters ensures improved accuracy of the Tent_BP_SSA2 hybrid model in predicting effluent quality. Moreover, the Tent_BP_SSA2 model exhibited robust prediction ability (R2 of 0.9246) when applied to assess the effluent quality of an actual sewage treatment plant. The incorporation of integrated models comprising the sparrow search optimizing algorithm, tent chaotic mapping, and higher-order magnitude decomposition of input parameters has demonstrated the capacity to enhance the accuracy of effluent quality prediction. This study illuminates novel perspectives on the prediction of effluent quality and the assessment of effluent warnings in WWTPs.
更多查看译文
关键词
Artificial neural networks,Effluent quality prediction,Machine learning,Wastewater treatment plants
AI 理解论文
溯源树
样例
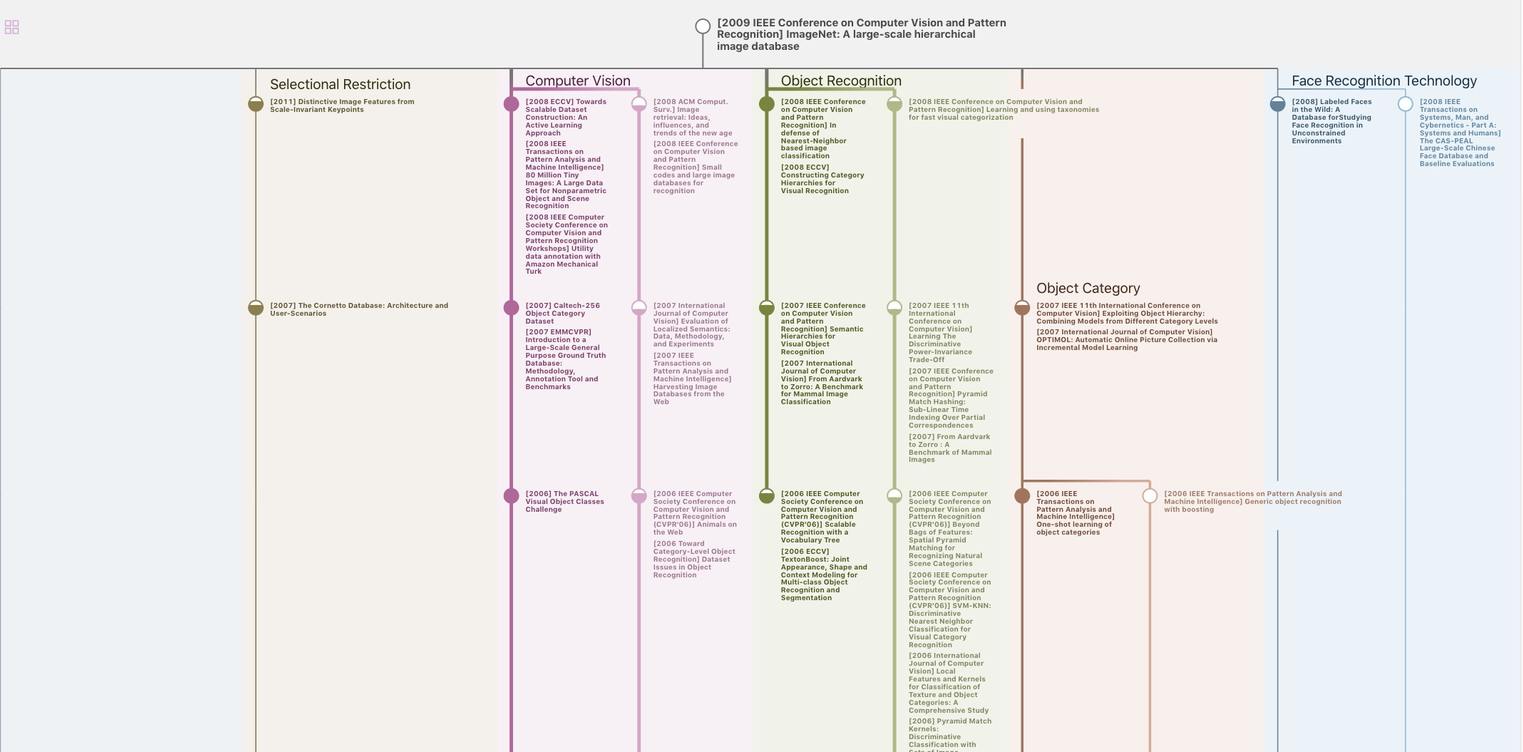
生成溯源树,研究论文发展脉络
Chat Paper
正在生成论文摘要