Learning Scalable Structural Representations for Link Prediction with Bloom Signatures
arXiv (Cornell University)(2023)
摘要
Graph neural networks (GNNs) have shown great potential in learning ongraphs, but they are known to perform sub-optimally on link prediction tasks.Existing GNNs are primarily designed to learn node-wise representations andusually fail to capture pairwise relations between target nodes, which provesto be crucial for link prediction. Recent works resort to learning moreexpressive edge-wise representations by enhancing vanilla GNNs with structuralfeatures such as labeling tricks and link prediction heuristics, but theysuffer from high computational overhead and limited scalability. To tackle thisissue, we propose to learn structural link representations by augmenting themessage-passing framework of GNNs with Bloom signatures. Bloom signatures arehashing-based compact encodings of node neighborhoods, which can be efficientlymerged to recover various types of edge-wise structural features. We furthershow that any type of neighborhood overlap-based heuristic can be estimated bya neural network that takes Bloom signatures as input. GNNs with Bloomsignatures are provably more expressive than vanilla GNNs and also morescalable than existing edge-wise models. Experimental results on five standardlink prediction benchmarks show that our proposed model achieves comparable orbetter performance than existing edge-wise GNN models while being 3-200× faster and more memory-efficient for online inference.
更多查看译文
关键词
Signal Processing on Graphs,Representation Learning,Knowledge Graph Embedding,Network Embedding,Graph Neural Networks
AI 理解论文
溯源树
样例
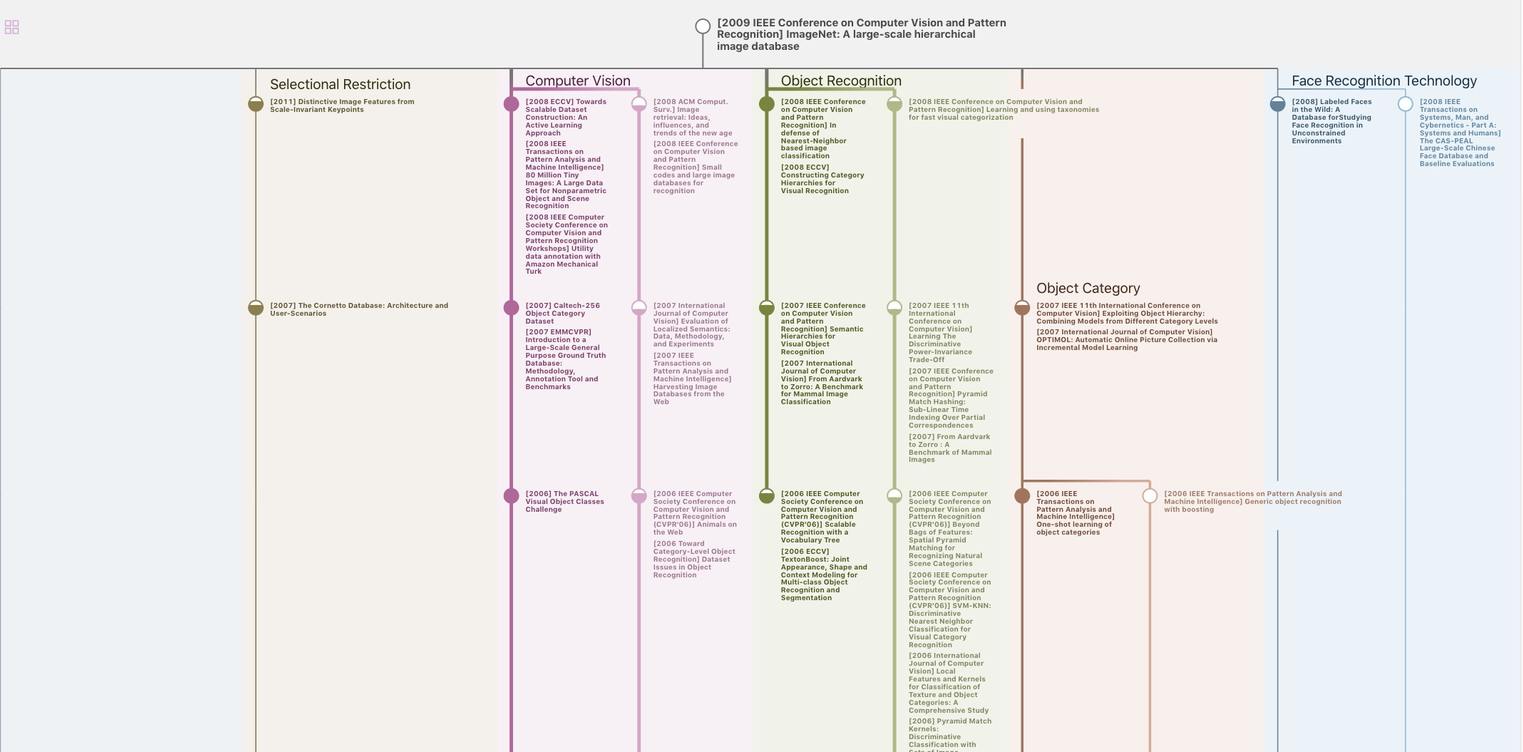
生成溯源树,研究论文发展脉络
Chat Paper
正在生成论文摘要