WRA-SS: A High-Performance Accelerator Integrating Winograd With Structured Sparsity for Convolutional Neural Networks
IEEE TRANSACTIONS ON VERY LARGE SCALE INTEGRATION (VLSI) SYSTEMS(2024)
摘要
Sparsification for convolutional neural networks (CNNs) and convolution acceleration algorithms such as the Winograd algorithm are two efficient ways to reduce the intensive computations of existing CNNs. To better combine the sparsification and Winograd algorithm, a close integration method is proposed to dynamically reduce the invalid parameters following the Winograd transformation. To address the limitation of data bandwidth, a hierarchical two-level storage structure and corresponding data scheduling scheme are proposed, which can realize a conflict-free scheduling process. In addition, an algorithm hardware codesign method is proposed to efficiently and flexibly reduce the invalid computations led by the previous filter decomposition method. The accelerator is evaluated on Xilinx XCVU9P FPGA, reaching 412-MHz clock frequency. Compared to state-of-the-art designs, WRA-SS can achieve 1.54-5.33x and 1.17-7.39x performance improvement for VGG-16 under 80% weight sparsity and 0% weight sparsity, respectively.
更多查看译文
关键词
Convolutional neural network,data storage and schedule,sparse neural network,Winograd algorithm
AI 理解论文
溯源树
样例
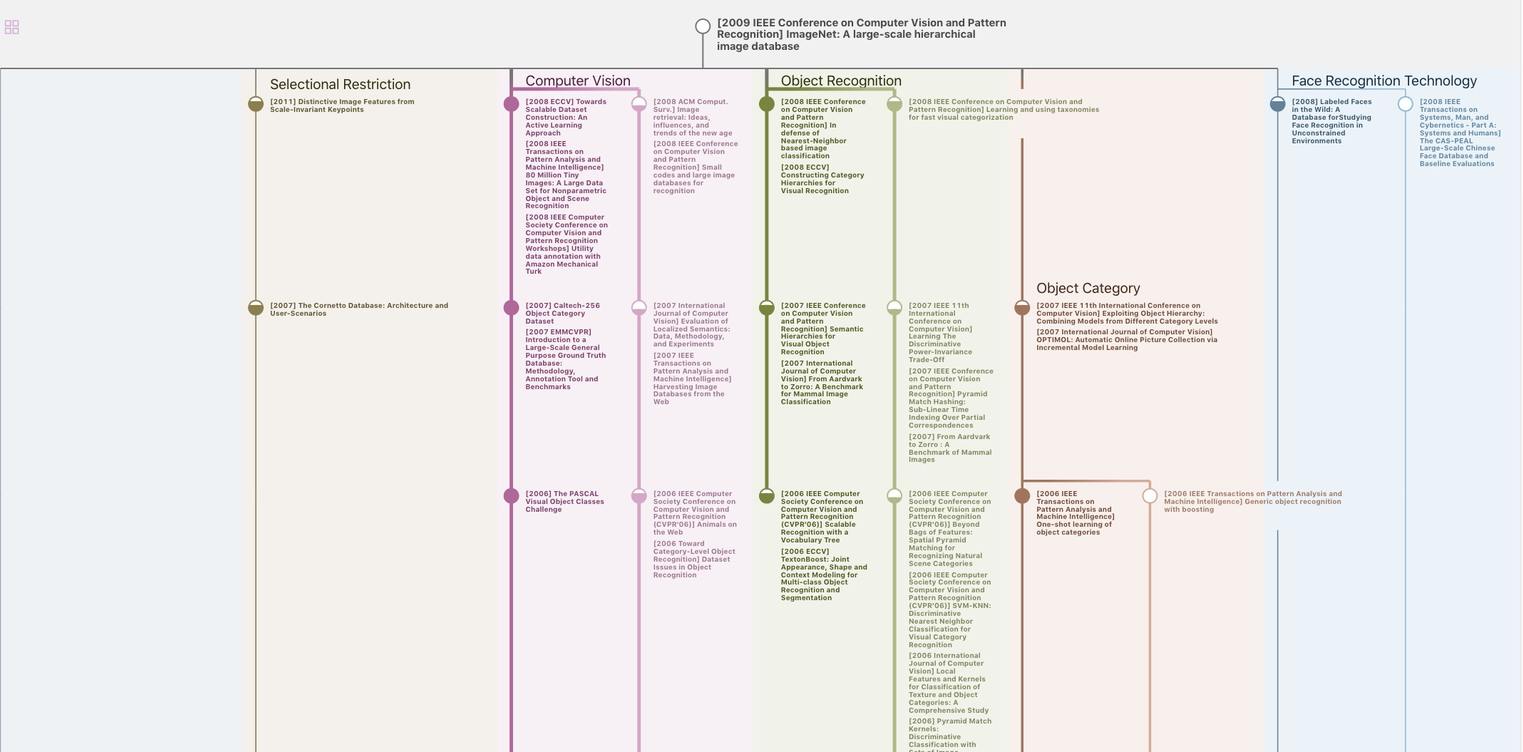
生成溯源树,研究论文发展脉络
Chat Paper
正在生成论文摘要