JIST: Joint Image and Sequence Training for Sequential Visual Place Recognition
IEEE ROBOTICS AND AUTOMATION LETTERS(2024)
摘要
Visual Place Recognition aims at recognizing previously visited places by relying on visual clues, and it is used in robotics applications for SLAM and localization. Since typically a mobile robot has access to a continuous stream of frames, this task is naturally cast as a sequence-to-sequence localization problem. Nevertheless, obtaining sequences of labelled data is much more expensive than collecting isolated images, which can be done in an automated way with little supervision. As a mitigation to this problem, we propose a novel Joint Image and Sequence Training (JIST) protocol that leverages large uncurated sets of images through a multi-task learning framework. With JIST we also introduce SeqGeM, an aggregation layer that revisits the popular GeM pooling to produce a single robust and compact embedding from a sequence of single-frame embeddings. We show that our model is able to outperform previous state of the art while being faster, using eight times smaller descriptors, having a lighter architecture and allowing to process sequences of various lengths.
更多查看译文
关键词
Training,Task analysis,Visualization,Databases,Multitasking,Streaming media,Location awareness,Representation learning,simultaneous localization and mapping,visual information retrieval
AI 理解论文
溯源树
样例
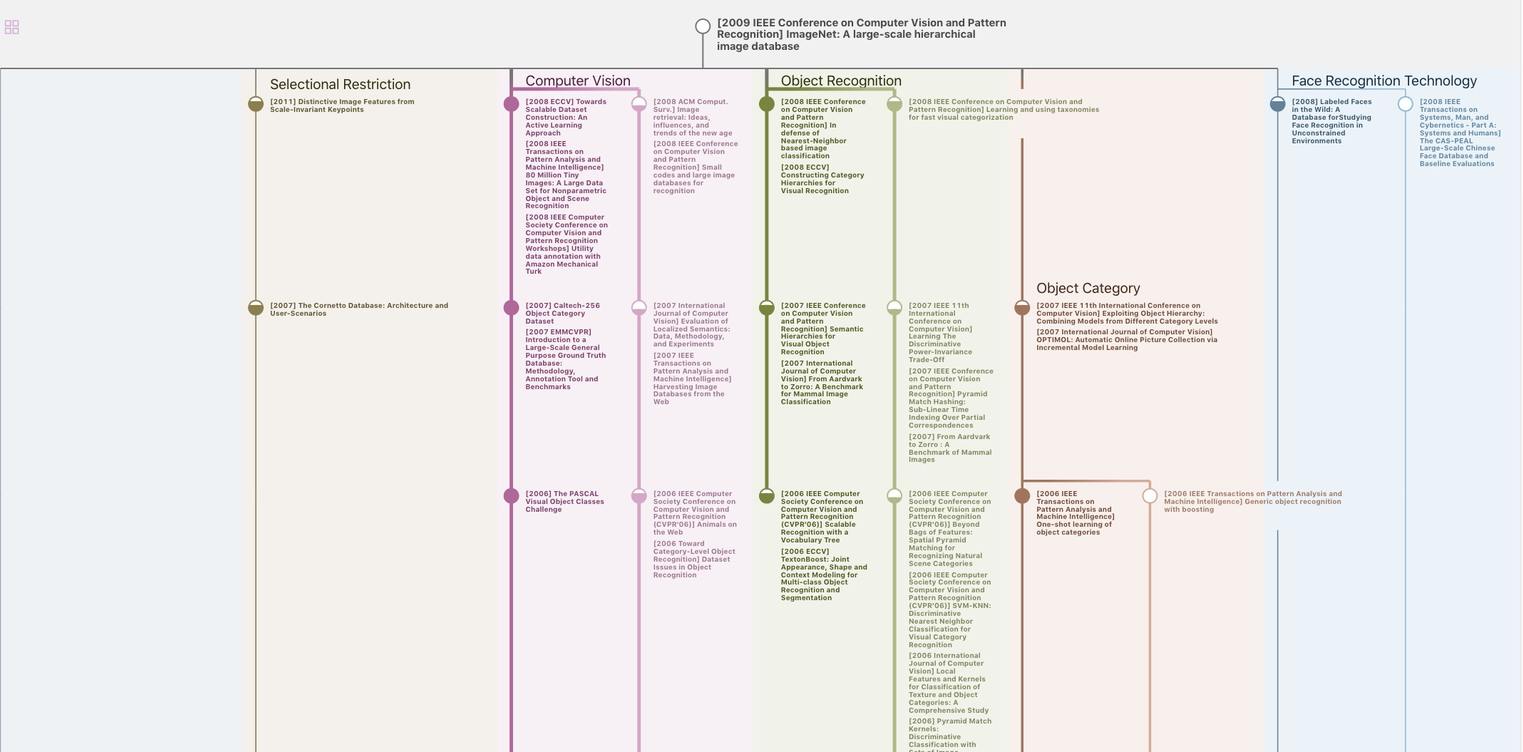
生成溯源树,研究论文发展脉络
Chat Paper
正在生成论文摘要