Rethinking Pan-Sharpening via Spectral-Band Modulation
IEEE TRANSACTIONS ON GEOSCIENCE AND REMOTE SENSING(2024)
摘要
Pan-sharpening aims to super-resolve the low-resolution (LR) multispectral (MS) image under the guidance of a high-resolution (HR) panchromatic (PAN) image. Existing deep learning (DL)-based pan-sharpening methods usually adhere to a common philosophy of learning complementary information between MS and PAN images. Despite remarkable advances, few studies consider the band-private characteristics which differ greatly from band to band. An ideal MS image, however, is jointly determined by its diverse spectral bands, thus the accurate restoration of every band will benefit the pan-sharpening performance. In this work, we propose a novel yet effective solution to reconstruct the HRMS image by explicitly modulating every spectral band under the conditions of the PAN image. As a result, we design a spatially-adaptive spectral modulation network, dubbed SSMNet, which consists of three core designs: source-aware spectral modulator (SSM), cross-band information aggregation (CBIA) module, and cross-stage feature integration (CSFI) module. The first predicts a series of spatially-adaptive kernels to capture the local information of every spectral band. Followed by, the second is responsible for facilitating the information communication among various bands to guarantee continuous spectral representations. Furthermore, the third attends to integrate the cross-stage output features to produce the pan-sharpened result. In addition, we also introduce the histogram loss to constrain the band-wise distribution of the final fused products. Extensive experiments demonstrate that our SSMNet achieves favorable performance against other state-of-the-art (SOTA) methods on multiple satellite datasets. The code is available at https://github.com/ez4lionky/SSMNet/.
更多查看译文
关键词
Band-private characteristics,deep learning (DL),histogram loss,pan-sharpening,spectral modulation
AI 理解论文
溯源树
样例
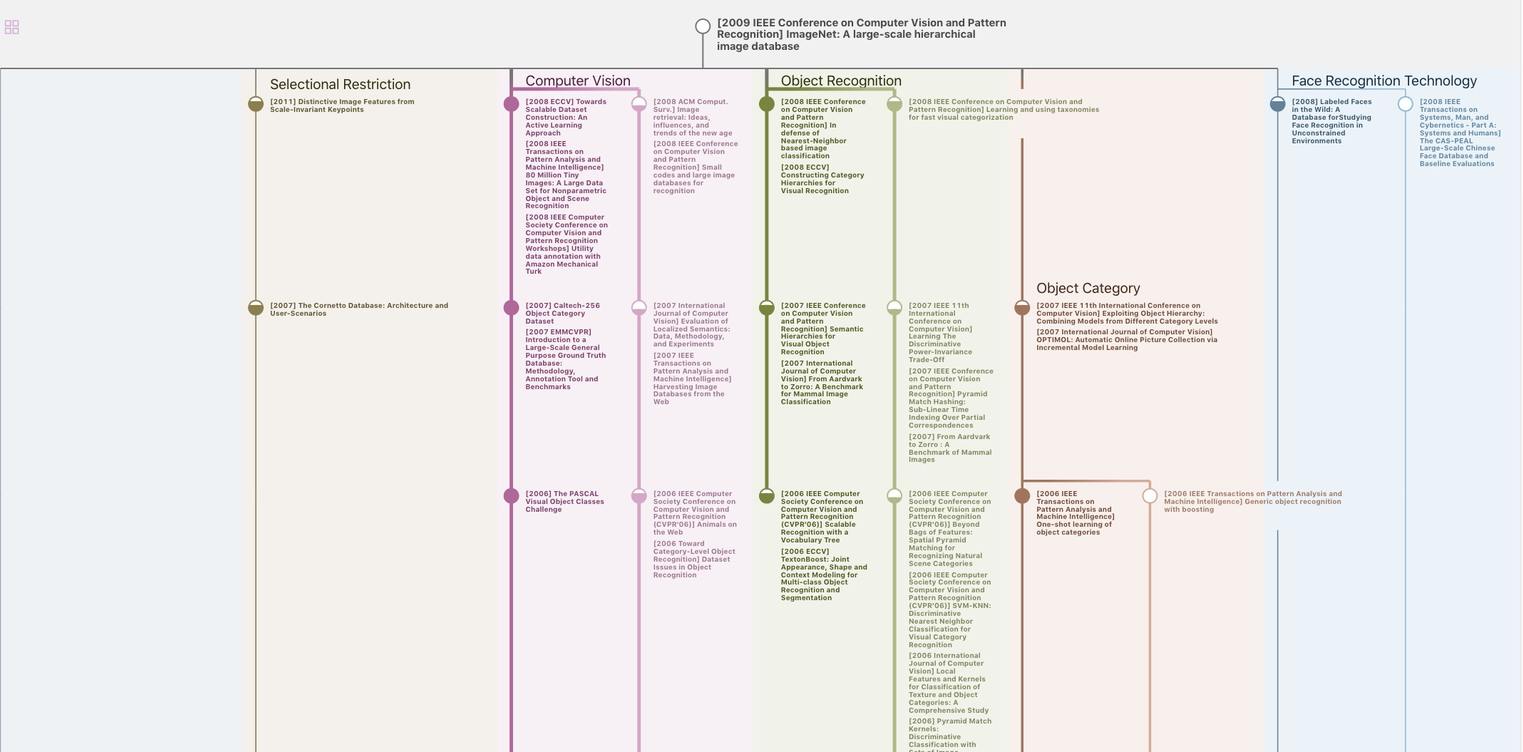
生成溯源树,研究论文发展脉络
Chat Paper
正在生成论文摘要