A Lightweight Integer-STBP On-Chip Learning Method of Spiking Neural Networks For Edge Processors.
2023 IEEE International Conference on Integrated Circuits, Technologies and Applications (ICTA)(2023)
摘要
Spiking Neural Networks (SNNs) with energy-efficient on neuromorphic hardware are suitable for edge processors with limited resources. The software-hardware co-design plays a crucial role in achieving optimal performance in such processors. Current research focuses on equipping edge processors with on-chip learning capabilities, and a high accuracy low hardware requirements on-chip learning algorithm is needed. Spatio-Temporal BackPropagation (STBP) algorithm enables deep-layer SNNs and high-accuracy training, shows great potential as an on-chip learning algorithm. However, the high computational complexity and storage requirements of STBP making edge processors design extremely challenging. Despite the efforts and significant progress made by many studies, the complex calculations required for surrogate gradient and the high storage requirements for membrane potentials still limit deployment. This work proposes a lightweight integer-STBP on-chip training method of SNNs for edge processors with lightweight surrogate gradients and low-storage computation flow, significantly reducing computational complexity and storage requirements. Experimental shows that, the proposed method achieve the same accuracy as state-of-the-art method while reducing the operations without MAC to 66% and storage requirements to 22%.
更多查看译文
关键词
spiking neural networks,on-chip learning,edge processors,software-hardware co-design
AI 理解论文
溯源树
样例
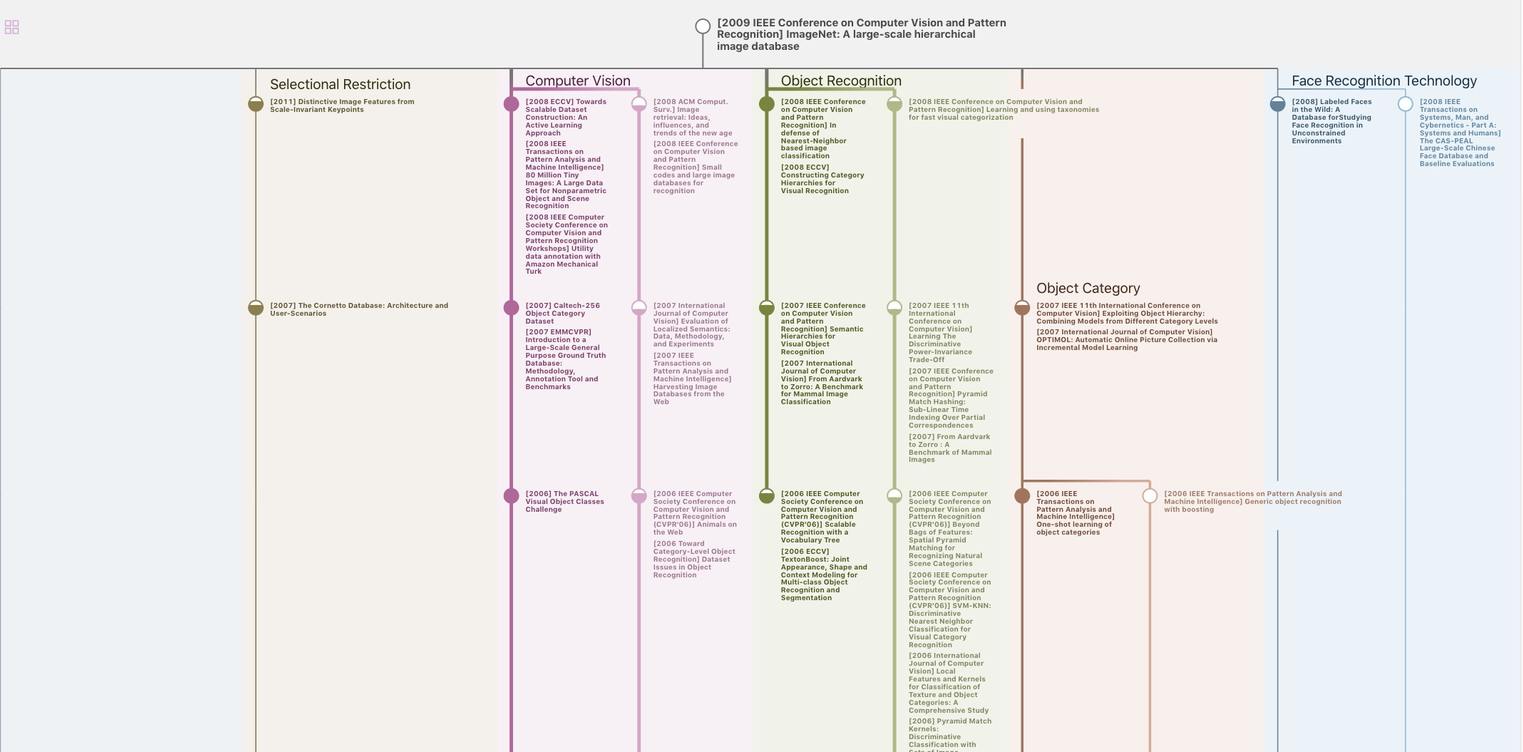
生成溯源树,研究论文发展脉络
Chat Paper
正在生成论文摘要