Digital Twinning in Precision Agriculture: Fabrication of a Close-Range Photogrammetry and Microclimate IoT-Enabled Data Acquisition System
2023 6th International Conference on Applied Computational Intelligence in Information Systems (ACIIS)(2023)
摘要
Volatility in the agriculture sector is increasing due to the market and especially the climate conditions. In precision agriculture, resource optimization is key for cost reduction, emission control, and efficiency. However, this relies on large data volumes. With insufficient data, mathematical and machine learning models can fail. This issue is particularly pronounced in developing countries like the Philippines, where agricultural data infrastructure is often lacking. This deficiency can lead to poor decision-making, inefficient resource allocation, and negative impacts on agricultural production and economy. With IoT, massive amounts of data can be aggregated to create Systems of Digital Twins, particularly for Precision Agriculture. There are multiple designs and approaches for Digital Twinning in Precision Agriculture. This paper presents the fabrication of a photogrammetric crop pseudo-volume data acquisition system in a hydroponic setup. Moreover, a realtime microclimate and nutrient availability data gathering system that showed current values and historical data was created. Results showed that the low-cost close-range photogrammetry image capture system’s execution, the microclimate gathering system’s performance, and the dashboard’s renditions were reliable. The photogrammetric pseudo-volumes were linearly related to the ground truth weight of the crops with an R
2
of 0.87. Additionally, the Pearson correlation between the actual weight and pseudo-volume resulted in a value of 0.93 which signifies a strong positive correlation. Therefore, the photogrammetric pseudo-volumes can be reliably and remotely used to predict the current fresh weight of the crops. To forward this research, more data from multiple cropping cycles is needed for robust machine learning applications.
更多查看译文
关键词
Photogrammetry,Internet of Things,Digital Twins,Optimization,Precision Agriculture
AI 理解论文
溯源树
样例
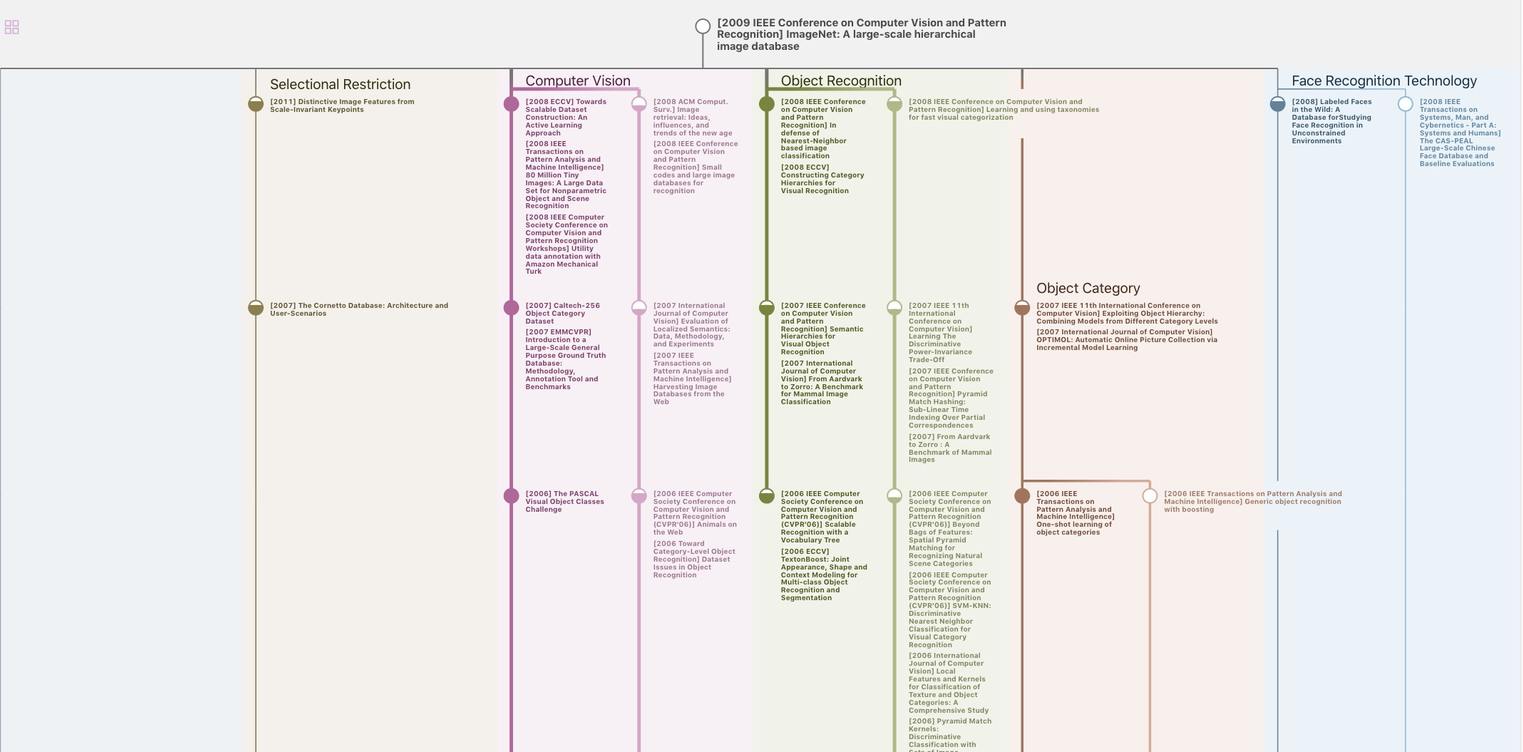
生成溯源树,研究论文发展脉络
Chat Paper
正在生成论文摘要