Spatiotemporal prediction of carbon emissions using a hybrid deep learning model considering temporal and spatial correlations
ENVIRONMENTAL MODELLING & SOFTWARE(2024)
摘要
Accurate prediction of carbon emissions plays a crucial role in enabling government decision-makers to formulate appropriate policies and plan necessary response measures in a timely manner. This study explored the spatiotemporal prediction methods for carbon emissions from temporal and spatial correlation perspectives. Specifically, a deep learning-based hybrid prediction framework for carbon emissions was developed. It includes three sequentially linked modules: gated recurrent units for modelling temporal correlation features, graph convolutional networks for modelling spatial correlation features and spatiotemporal prediction. The proposed model enables one-and multi-step spatiotemporal prediction of carbon emissions. The monthly Open-source Data Inventory for Anthropogenic CO2 data for three major urban agglomerations in China were utilised to assess the performance of our model. Results indicate that our model outperforms the baseline models in terms of predictive accuracy for single-and multi-step spatiotemporal predictions. Additionally, our model demonstrates good generalisation through further application experiments.
更多查看译文
关键词
Carbon emission,Time series,Temporal correlation,Spatial correlation,Spatiotemporal prediction
AI 理解论文
溯源树
样例
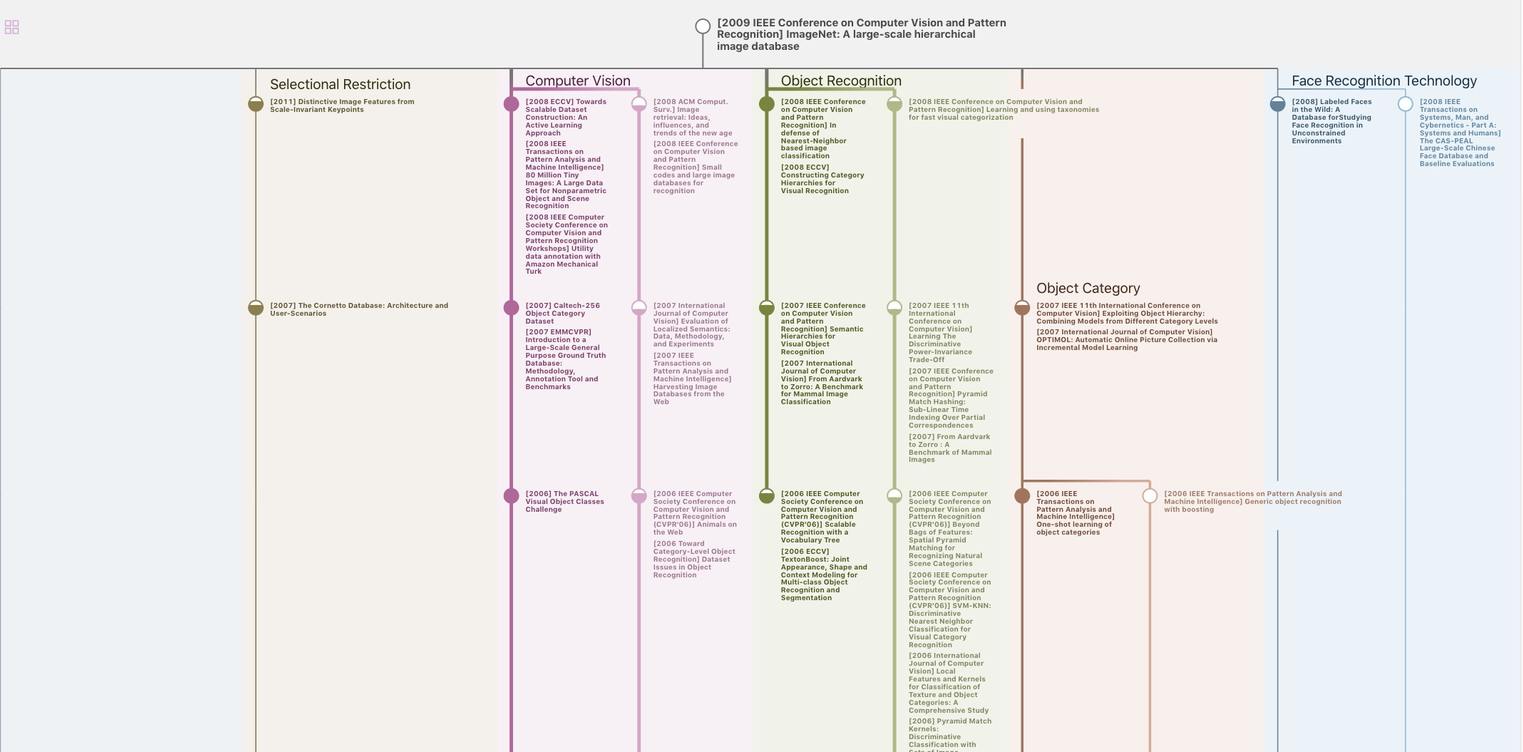
生成溯源树,研究论文发展脉络
Chat Paper
正在生成论文摘要