A robust autoregressive long-term spatiotemporal forecasting framework for surrogate-based turbulent combustion modeling via deep learning
ENERGY AND AI(2024)
摘要
This paper systematically develops a high-fidelity turbulent combustion surrogate model using deep learning. We construct a surrogate model to simulate the turbulent combustion process in real time, based on a state-of-the-art spatiotemporal forecasting neural network. To address the issue of shifted distribution in autoregressive long-term prediction, two training techniques are proposed: unrolled training and injecting noise training. These techniques significantly improve the stability and robustness of the model. Two datasets of turbulent combustion in a combustor with cavity and a vitiated co-flow burner (Cabra burner) have been generated for model validation. The effects of model architecture, unrolled time, noise amplitude, and training dataset size on the long-term predictive performance are explored. The well-trained model can be applicable to new cases by extrapolation and give spatially and temporally consistent results in long-term predictions for turbulent reacting flows that are highly unsteady.
更多查看译文
关键词
Turbulent combustion,Detailed reaction mechanism,Transient simulation,Deep neural network,Spatiotemporal series prediction,Long-term forecast stability
AI 理解论文
溯源树
样例
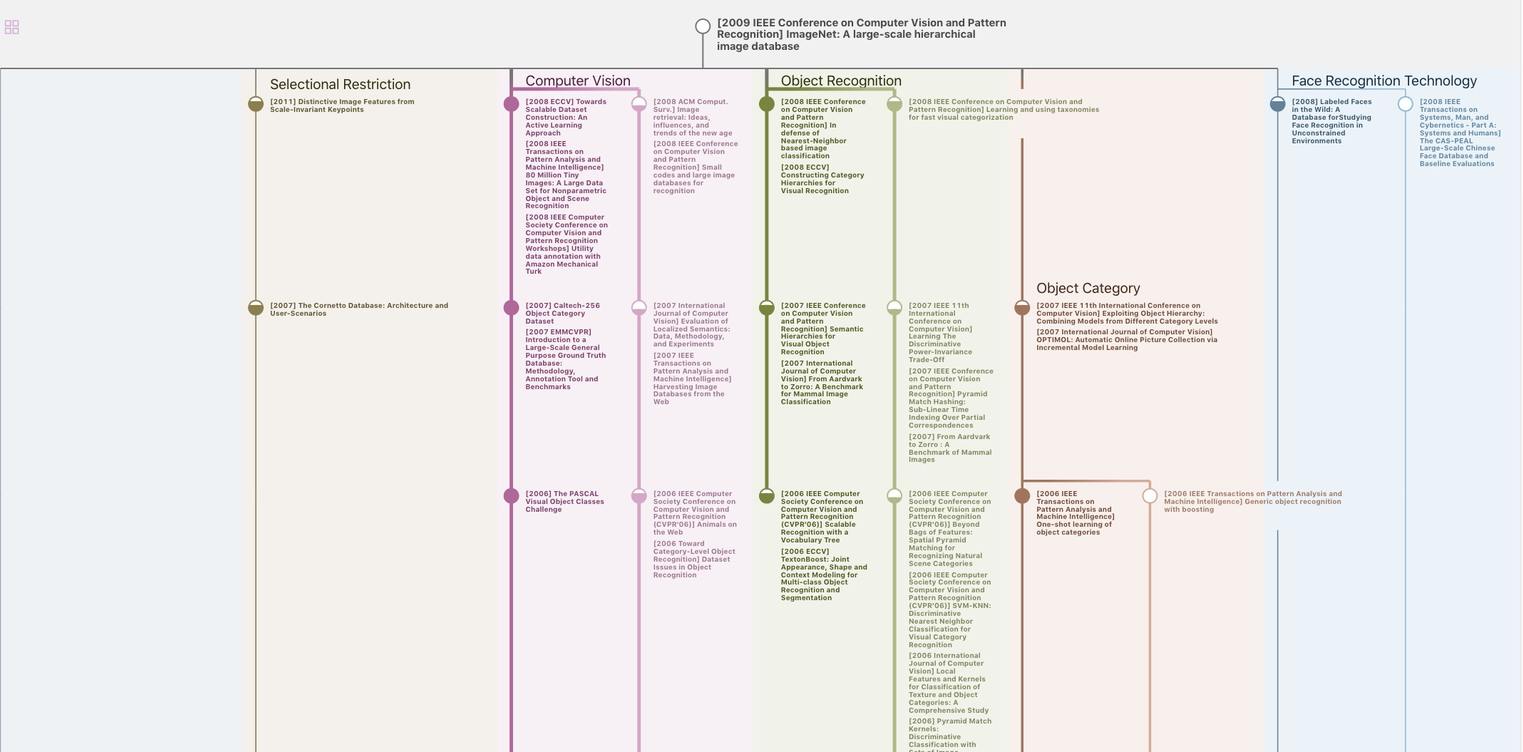
生成溯源树,研究论文发展脉络
Chat Paper
正在生成论文摘要