Dynamic Weight-Guided Smooth-Sparse Decomposition for Small Target Detection Against Strong Vignetting Background
IEEE TRANSACTIONS ON INSTRUMENTATION AND MEASUREMENT(2024)
摘要
Small target detection against a strong vignetting background poses two major challenges: the submergence of the target in a high-brightness background and the difficult separability between dense noise and a weak target. These challenges have not been adequately addressed by existing methods, which heavily rely on the low-rank prior background. In this article, our focus shifts to the smoothness of the background and proposes a dynamic weight-guided smooth-sparse decomposition (DW-SSD) model. First, we adopt a surface-fitting perspective to reconstruct the smooth vignetting, where the B-spline is introduced as the basis, and total variation (TV) is utilized to regularize the coefficients of the B-spline basis. This term provides a flexible description for different background local regions with low computation cost, effectively suppressing the high brightness vignetting and facilitating the emergence of the target. Second, a dynamic weight based on spatial-temporal prior characteristic is added to the target component, where the differences in spatial contrast and temporal profile are jointly considered to guide a precise separation of the target from dense noise. Finally, a customized solution is designed based on the alternating direction method of multipliers (ADMM) to separate small targets from strong vignetting rapidly and precisely. Comprehensive experiments on five real sequences demonstrate that the DW-SSD method effectively overcomes the challenges posed by strong vignetting and performs well in real-time small target detection. The code is publicly available at https://github.com/D-IceIce/DW-SSD.
更多查看译文
关键词
B-spline,dynamic weight,small target detection,smooth-sparse decomposition,strong vignetting
AI 理解论文
溯源树
样例
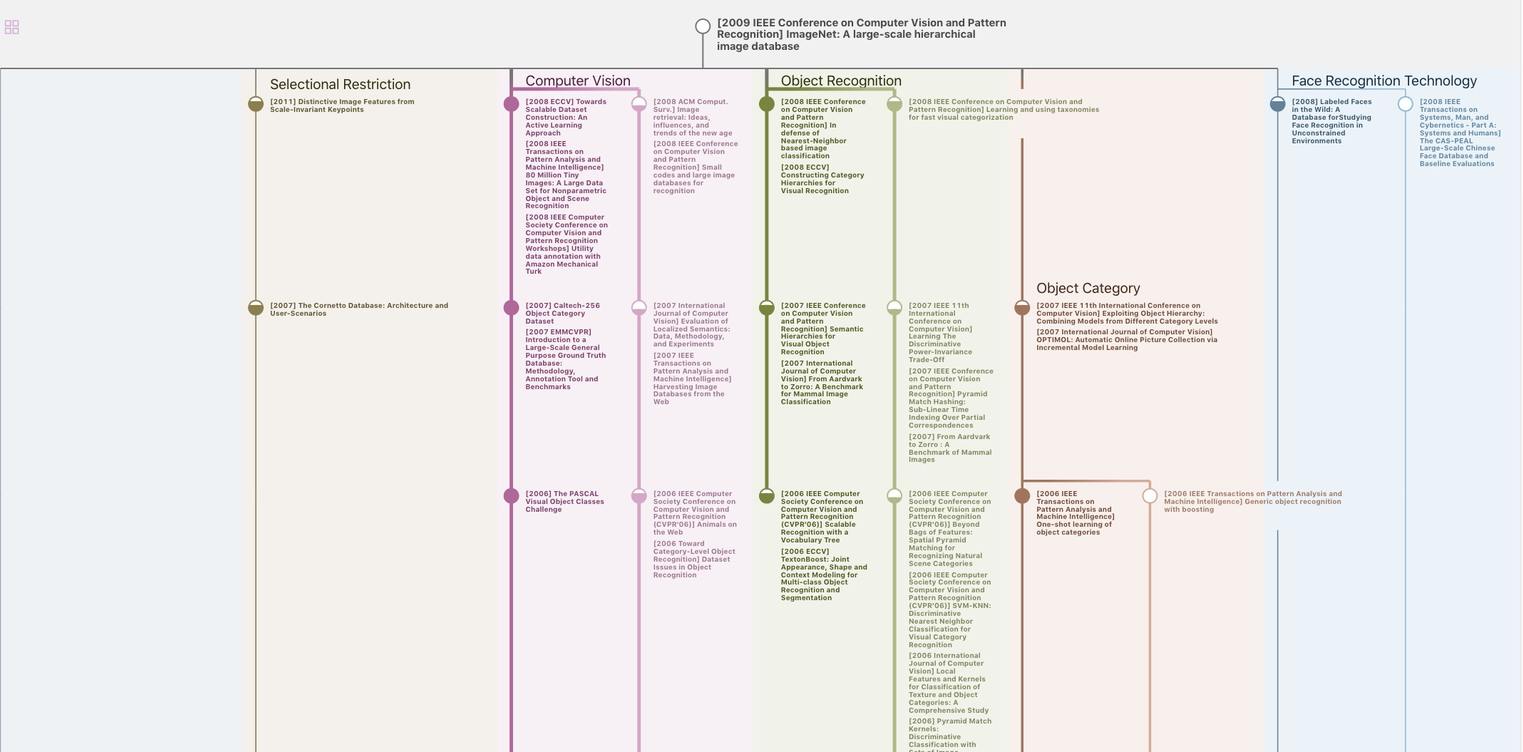
生成溯源树,研究论文发展脉络
Chat Paper
正在生成论文摘要