TDFNet: An Efficient Audio-Visual Speech Separation Model with Top-down Fusion
2023 13th International Conference on Information Science and Technology (ICIST)(2024)
摘要
Audio-visual speech separation has gained significant traction in recent
years due to its potential applications in various fields such as speech
recognition, diarization, scene analysis and assistive technologies. Designing
a lightweight audio-visual speech separation network is important for
low-latency applications, but existing methods often require higher
computational costs and more parameters to achieve better separation
performance. In this paper, we present an audio-visual speech separation model
called Top-Down-Fusion Net (TDFNet), a state-of-the-art (SOTA) model for
audio-visual speech separation, which builds upon the architecture of TDANet,
an audio-only speech separation method. TDANet serves as the architectural
foundation for the auditory and visual networks within TDFNet, offering an
efficient model with fewer parameters. On the LRS2-2Mix dataset, TDFNet
achieves a performance increase of up to 10% across all performance metrics
compared with the previous SOTA method CTCNet. Remarkably, these results are
achieved using fewer parameters and only 28% of the multiply-accumulate
operations (MACs) of CTCNet. In essence, our method presents a highly effective
and efficient solution to the challenges of speech separation within the
audio-visual domain, making significant strides in harnessing visual
information optimally.
更多查看译文
关键词
Audio-Visual,Multi-Modal,Speech-Separation
AI 理解论文
溯源树
样例
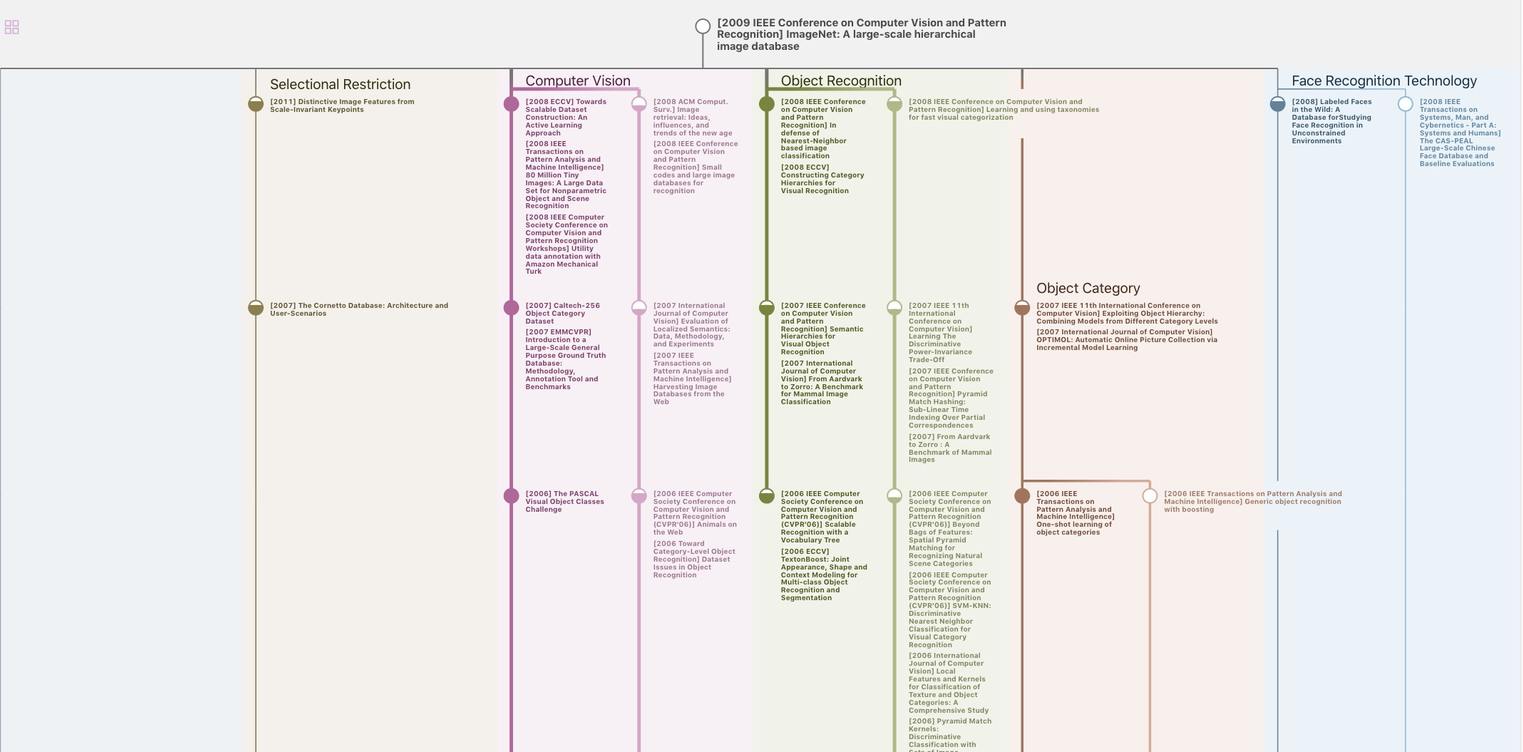
生成溯源树,研究论文发展脉络
Chat Paper
正在生成论文摘要