End-to-End Trainable Deep Neural Network for Radar Interference Detection and Mitigation
2023 IEEE International Radar Conference (RADAR)(2023)
摘要
The automotive radar sensor is an essential sensor for intelligent transportation systems. However, the increasing number of such systems leads to mutual interference between sensors. Radar interference can be a severe disturbance, where targets within the victim radar are not perceived anymore if no countermeasures are taken. To prevent fatal injuries caused by this disturbance, several classical and deep-learning based methods to detect and mitigate radar interference have been proposed. We present an end-to-end trainable deep neural network for radar interference detection and mitigation. We evaluate different radar interference detection and mitigation methods on a large scale urban driving dataset using simulated radar interference. To our knowledge, this is the first end-to-end trainable architecture for interference detection and mitigation in the time domain. We show that our method achieves state-of-the-art results in interference detection and mitigation. Code for our method, reference methods, simulation, and the evaluation is available at https://github.com/KIT-MRT/ridam.
更多查看译文
关键词
radar interference mitigation,deep learning,radar signal processing,automotive radar
AI 理解论文
溯源树
样例
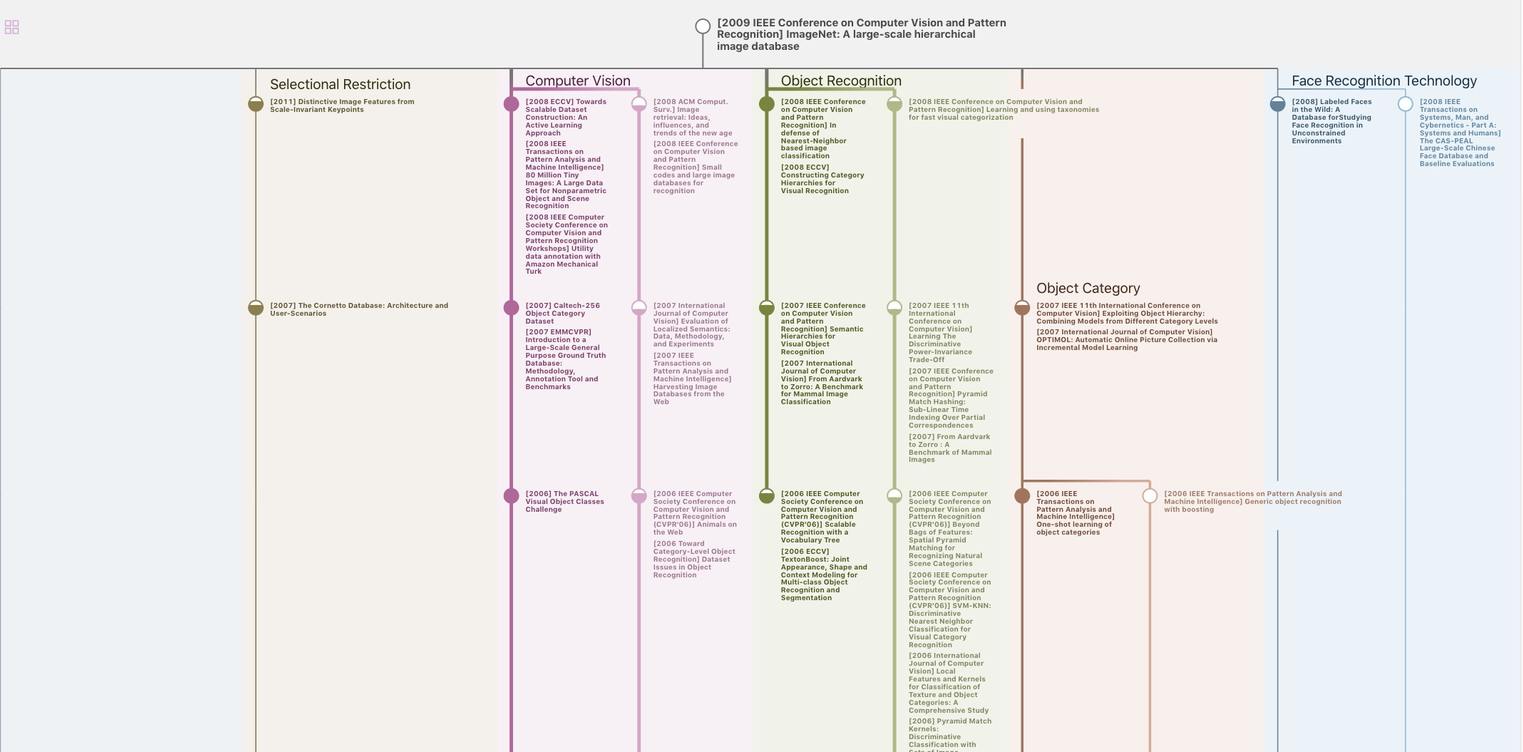
生成溯源树,研究论文发展脉络
Chat Paper
正在生成论文摘要