Source term inversion of short-lived nuclides in complex nuclear accidents based on machine learning using off-site gamma dose rate
JOURNAL OF HAZARDOUS MATERIALS(2024)
摘要
During nuclear accidents, large amounts of short-lived radionuclides are released into the environment, causing acute health hazards to local populations. Therefore, it is particularly important to obtain source-term information to assist nuclear emergency decision makers in determining emergency protective measures. However, it is extremely difficult to obtain reliable contaminant monitoring instrument readings to estimate the source term based on core conditions, release routes, and release conditions. Currently, a wide variety of source-term inversion methods are attracting increasing attention. In this study, the release rates of four typical short-lived nuclides (Kr-88, Sr-91, Te-132, I-131) in two complex nuclear accident scenarios were estimated using a machine-learning method. The results show that the best estimation performance is obtained with the long shortterm memory network, and the mean absolute percentage errors for the release rates of the four nuclides at 10 h under the two nuclear accidents are 9.87% and 11.08%, 17.49% and 16.51%, 7.16% and 8.35%, and 38.83% and 41.87%, respectively. Meanwhile, the mean absolute percentage errors for Te-132 (7.16% and 8.35%) were the lowest among all the estimated nuclides. In addition, stability analysis showed that the gamma dose rate was the key parameter affecting the estimation accuracy.
更多查看译文
关键词
Complex nuclear accident,Source -term inversion,Machine learning,Gamma dose rate
AI 理解论文
溯源树
样例
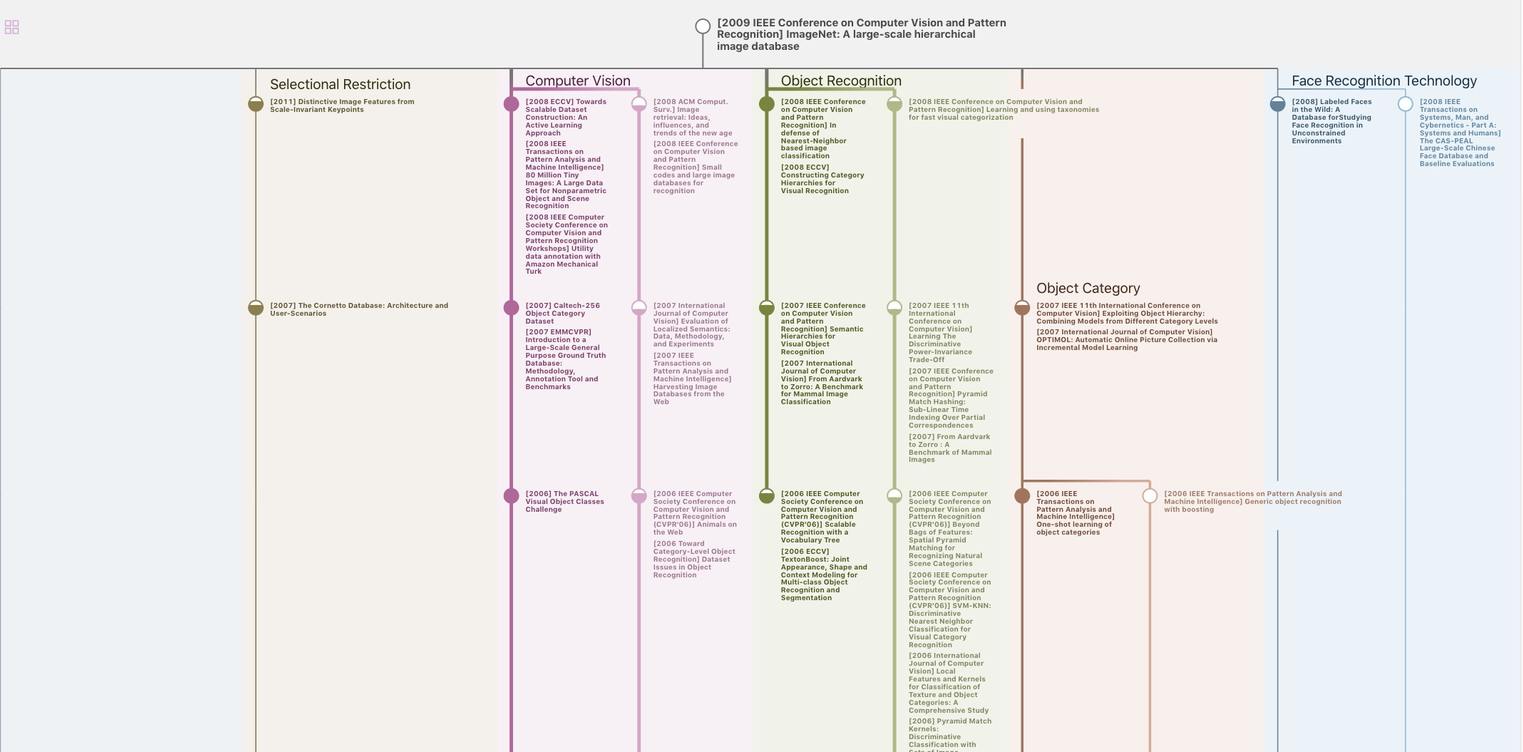
生成溯源树,研究论文发展脉络
Chat Paper
正在生成论文摘要