Empowering Spatial Knowledge Graph for Mobile Traffic Prediction
31ST ACM SIGSPATIAL INTERNATIONAL CONFERENCE ON ADVANCES IN GEOGRAPHIC INFORMATION SYSTEMS, ACM SIGSPATIAL GIS 2023(2023)
摘要
Accurately predicting base station traffic volumes and understanding mobile traffic patterns is essential for smart city development, enabling efficient resource allocation and ensuring high-quality communication services. However, existing works have limitations in capturing spatial information, though the surrounding environment plays a critical role in mobile traffic prediction. In this paper, we utilize a spatial knowledge graph to represent spatial information and add important urban components to augment it making it a more effective tool for capturing environmental information. we further propose a multi-relational knowledge graph convolutional network model for mobile traffic prediction, which consists of three parts. The environmental context modelling captures spatial information from the augmented spatial knowledge graph using tucker decomposition and relational graph convolutional network. The semantic relationship modelling extracts semantic relationships between base stations and employs transformer and causal convolution to capture temporal features. The inter-attentional fusion modelling utilizes the self-attention mechanism to further capture base station relationships and predict future traffic volumes. Extensive experiments demonstrate that our proposed model significantly outperforms the state-of-the-art models by over 10% in mobile traffic prediction. The code is available at https://github.com/tsinghua-fib-lab/Mobile-Traffic-Prediction-sigspatial23
更多查看译文
关键词
knowledge graph,mobile traffic prediction,graph neural networks
AI 理解论文
溯源树
样例
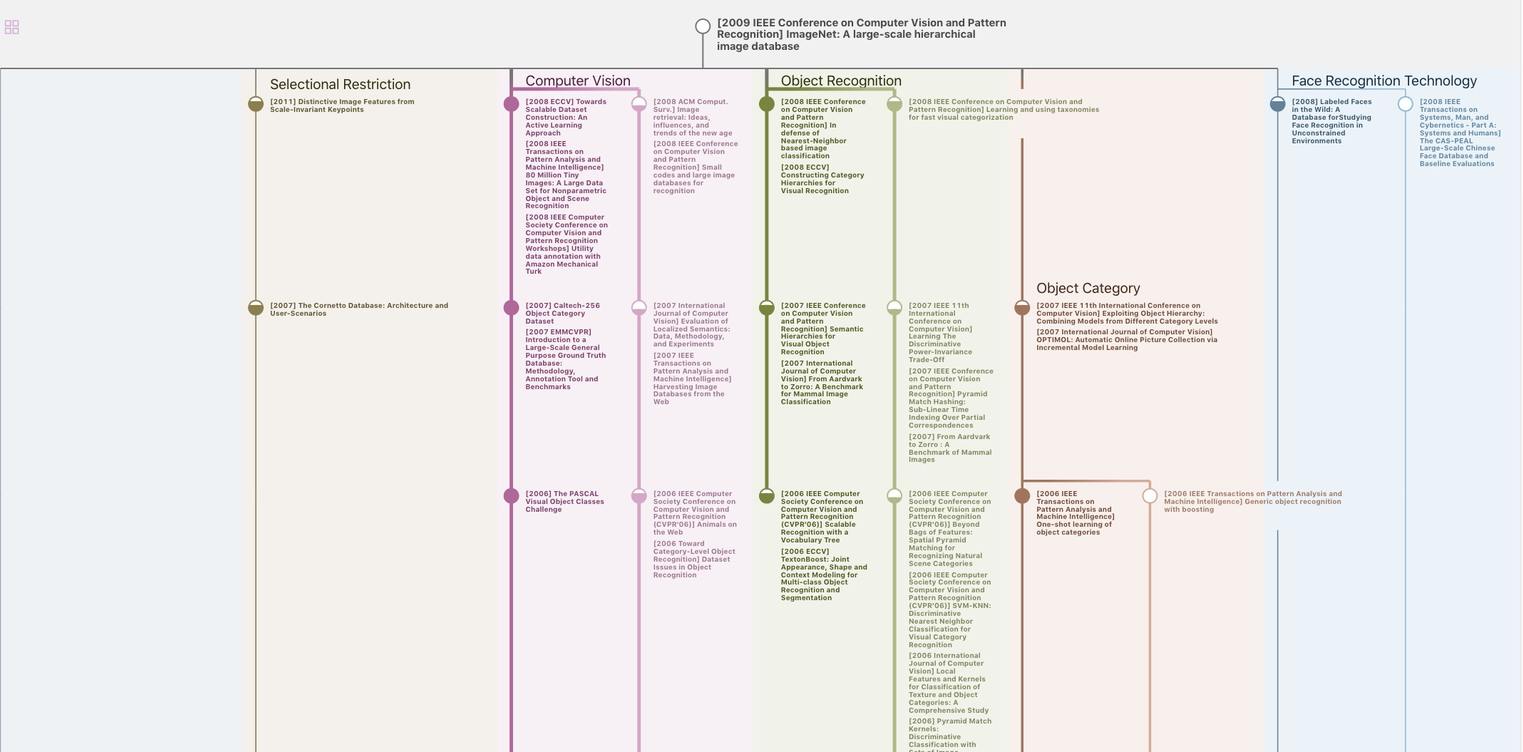
生成溯源树,研究论文发展脉络
Chat Paper
正在生成论文摘要