The Geospatial Generalization Problem: When Mobility Isn't Mobile
31ST ACM SIGSPATIAL INTERNATIONAL CONFERENCE ON ADVANCES IN GEOGRAPHIC INFORMATION SYSTEMS, ACM SIGSPATIAL GIS 2023(2023)
摘要
Human mobility research has significantly benefited from recent advances in machine learning, as have numerous other industries. Aided by the ever-increasing availability of geospatial and mobility data, machine learning models have enabled large-scale systems for simulating city-wide macro and micro mobility behaviors, urban planning, transportation management, and disaster relief optimization. However, while many fields have invested significant effort in solving the model transferability and generalization problem, the inability of machine learning-based human mobility models to generalize to new locations has come to be implicitly accepted in most geospatial research. In this vision paper, we focus on this geospatial generalization problem, its root causes, and how it is restricting the applications of otherwise-promising research. Most importantly, we argue for several data- and modeling-driven innovations which could help remedy this problem, spanning mega-scale simulations, large foundation models, and multi-task, transfer, and meta-learning. We also spotlight a handful of promising ideas which have recently emerged from the community. We hope that these proposals take root and help develop more capable, flexible, and generalizable models in research and industry.
更多查看译文
关键词
mobility,geospatial data,generalization,transfer learning,domain adaptation
AI 理解论文
溯源树
样例
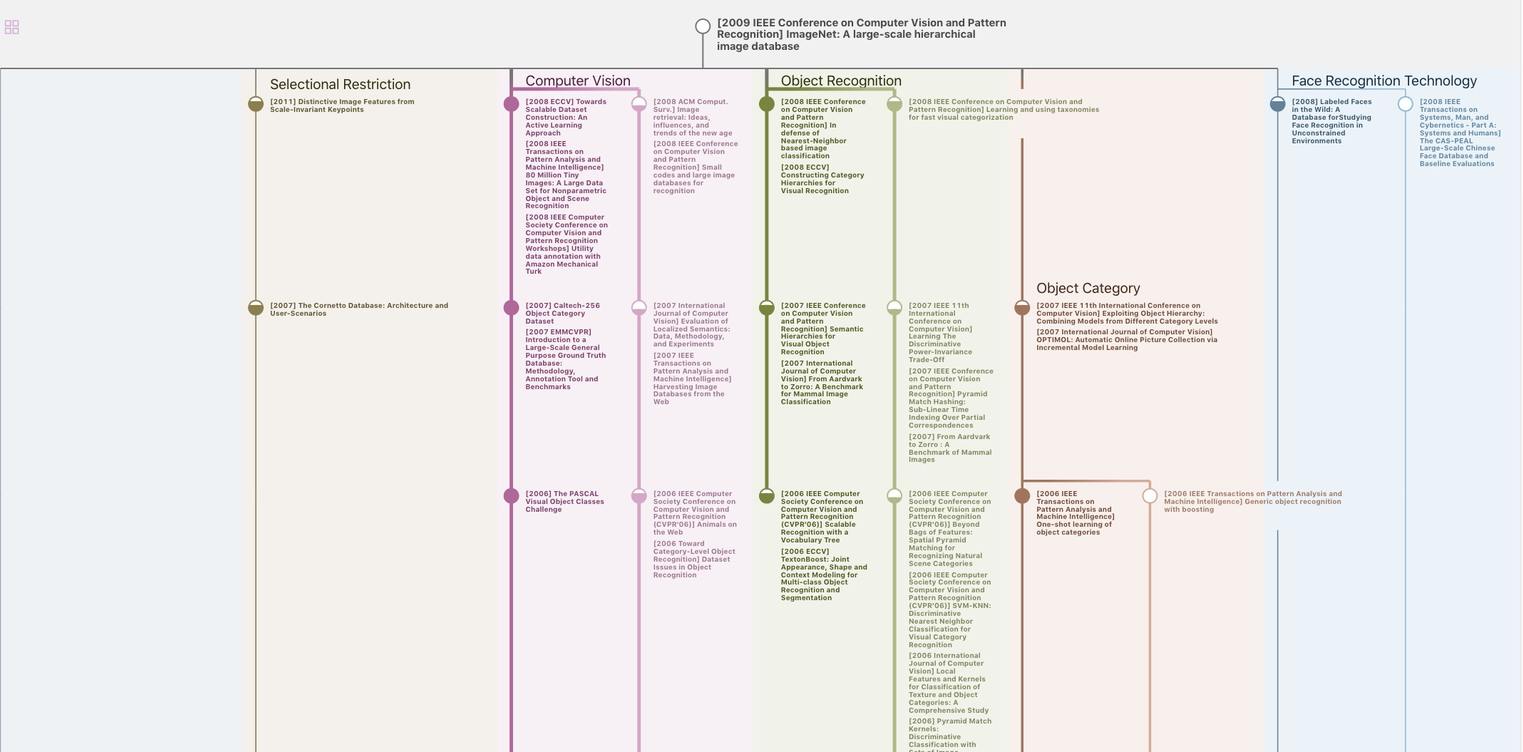
生成溯源树,研究论文发展脉络
Chat Paper
正在生成论文摘要