BRITD: behavior rhythm insider threat detection with time awareness and user adaptation
Cybersecurity(2024)
摘要
AbstractResearchers usually detect insider threats by analyzing user behavior. The time information of user behavior is an important concern in internal threat detection. Existing works on insider threat detection fail to make full use of the time information, which leads to their poor detection performance. In this paper, we propose a novel behavioral feature extraction scheme: we implicitly encode absolute time information in the behavioral feature sequences and use a feature sequence construction method taking covariance into account to make our scheme adaptive to users. We select Stacked Bidirectional LSTM and Feedforward Neural Network to build a deep learning-based insider threat detection model: Behavior Rhythm Insider Threat Detection (BRITD). BRITD is universally applicable to various insider threat scenarios, and it has good insider threat detection performance: it achieves an AUC of 0.9730 and a precision of 0.8072 with the CMU CERT dataset, which exceeds all baselines. Graphical Abstract
更多查看译文
关键词
Insider threat detection,Behavior pattern mining,Time information,User adaptive,Deep learning
AI 理解论文
溯源树
样例
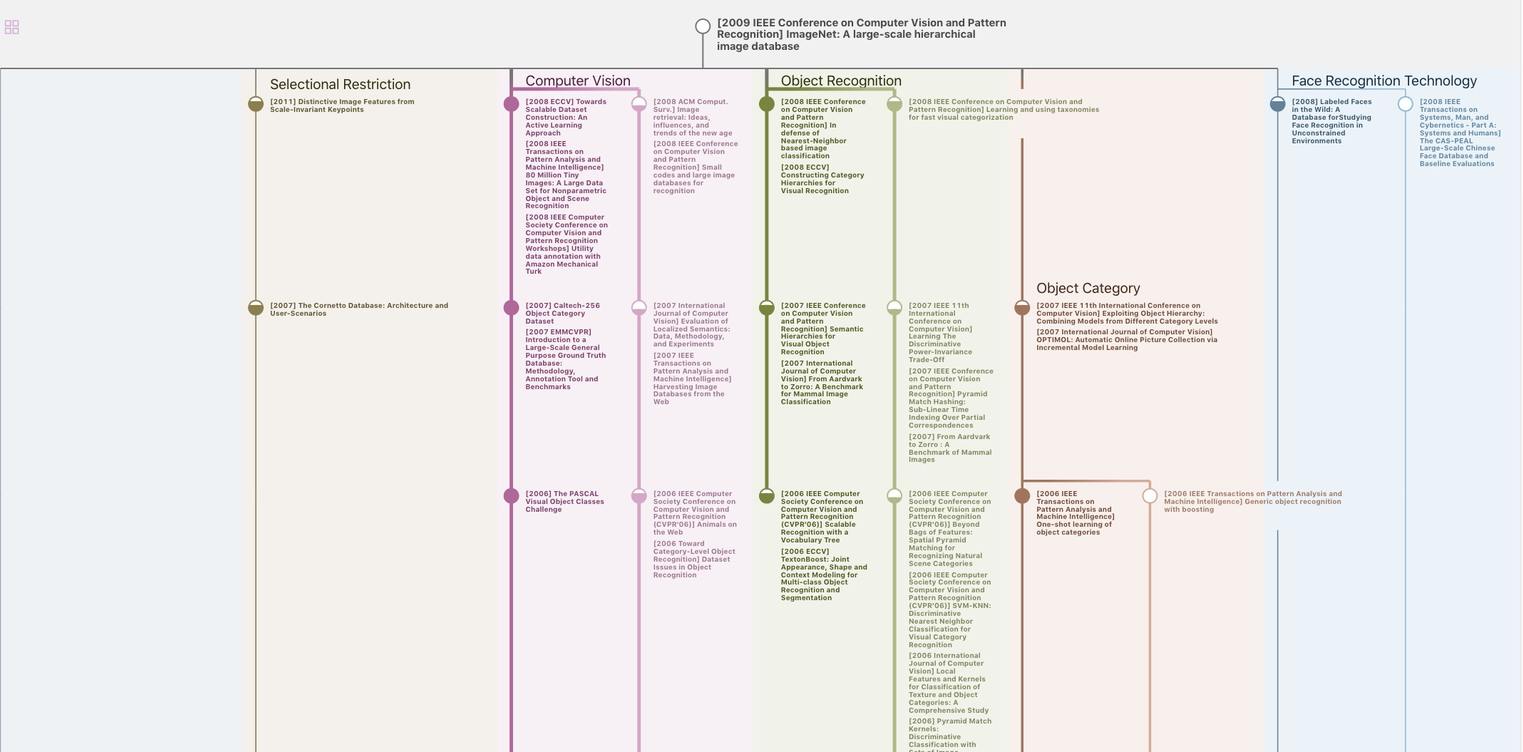
生成溯源树,研究论文发展脉络
Chat Paper
正在生成论文摘要