CANet: cross attention network for food image segmentation
MULTIMEDIA TOOLS AND APPLICATIONS(2023)
摘要
Food image segmentation which aims to distinguish various ingredients is crucial for food safety, as estimating calories and other nutrients is important for human health and sustainable development. However, the performances of current image segmentation methods are inferior on food image datasets due to the significant diversity of appearances and distinctive conditions between ingredients and daily props, while these methods have insufficient capabilities for feature extraction of food images. In addition, utilizing attention mechanisms to obtain contextual detail information and long-range dependencies leads to a quadratic computational complexity. In this paper, we propose a Cross Spatial Attention (CSA) module to extract richer spatial features from food images, with lower time and space complexity. Specifically, the CSA module aggregates the contextual information by cross-calculation of horizontal and vertical dimensions. And experiments demonstrate that, by taking a two-step cross-calculation, each pixel could eventually capture global long-range dependencies. Furthermore, our method integrates a Channel Attention (CA) module to selectively highlight interdependent channel information by integrating relevant features across all feature maps. Then the outputs of these two attention modules are aggregated to enhance the representation of the image feature. Convincing performance improvement is achieved on the FoodSeg103, UECFoodPix and ADE20K. Moreover, the proposed network achieved better trade-off between accuracy and efficiency.
更多查看译文
关键词
Food image segmentation,Cross attention network,Cross spatial attention,Channel attention,Food safety
AI 理解论文
溯源树
样例
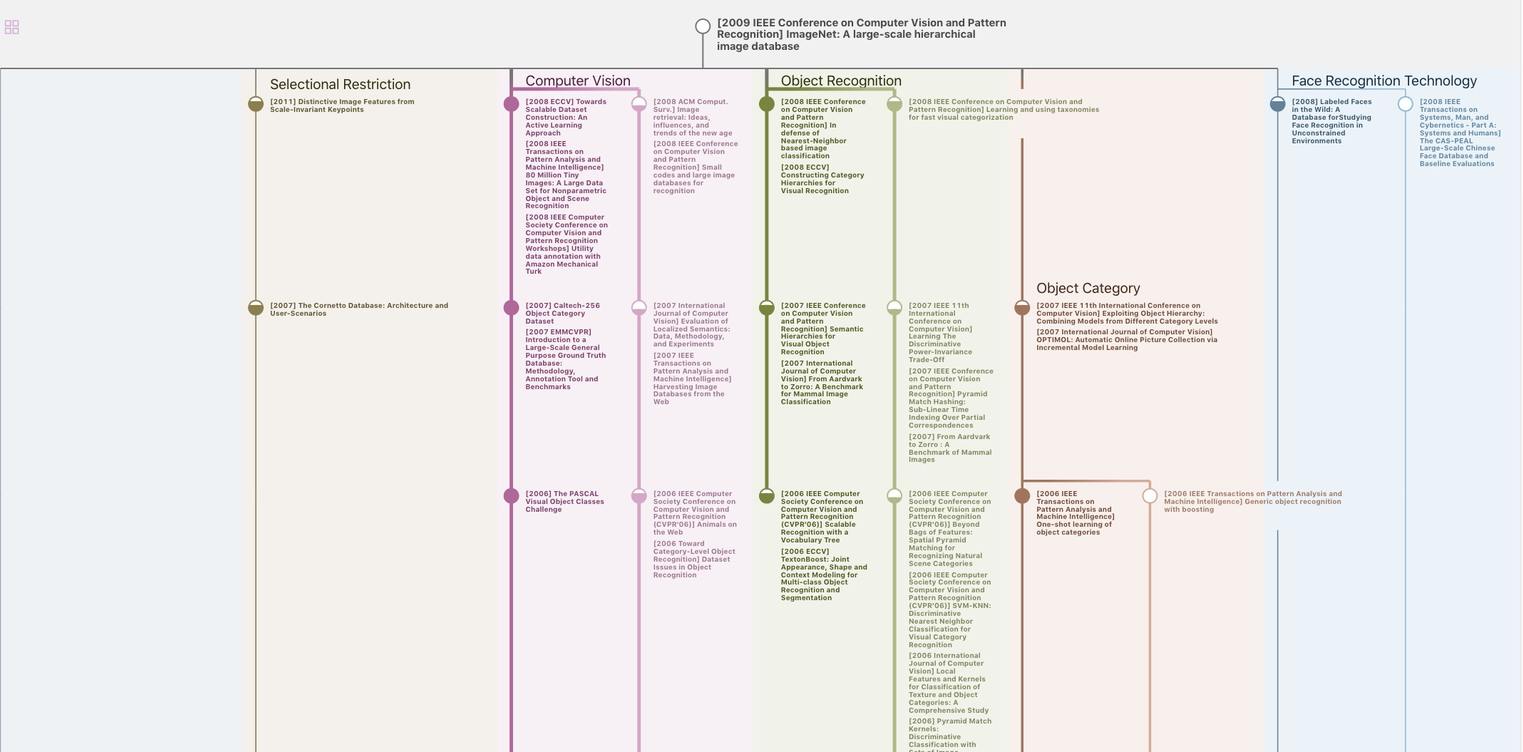
生成溯源树,研究论文发展脉络
Chat Paper
正在生成论文摘要