Pseudotime-based Deep Learning of Gene Regulatory Relationships from Single-Cell Expression Data.
ICBET(2023)
摘要
In this paper, we propose a pseudotime-based time-course deep learning method that combines trajectory inference and deep learning methods to infer gene regulatory relationships from single-cell RNA-seq data. We have applied our method to the inference of the regulatory relationships during cell differentiation of mouse embryonic stem cells. We have shown that during the process of cell differentiation, cells have a wide variety of cellular states, even at the same timepoint. This suggests that the deep learning of the regulatory relationships using pseudotime-based time-course expression data is more effective rather than that using real timepoint ones. Our pseudotime-based method has achieved better AUROC performance compared to the original time-course deep learning (TDL) with real timepoints. Also, we have shown that the prediction performance of the regulatory relationships can be greatly improved by focusing only on the cells of a representative cell lineage and by using deep conv-LSTM as a network architecture of the learning model.
更多查看译文
AI 理解论文
溯源树
样例
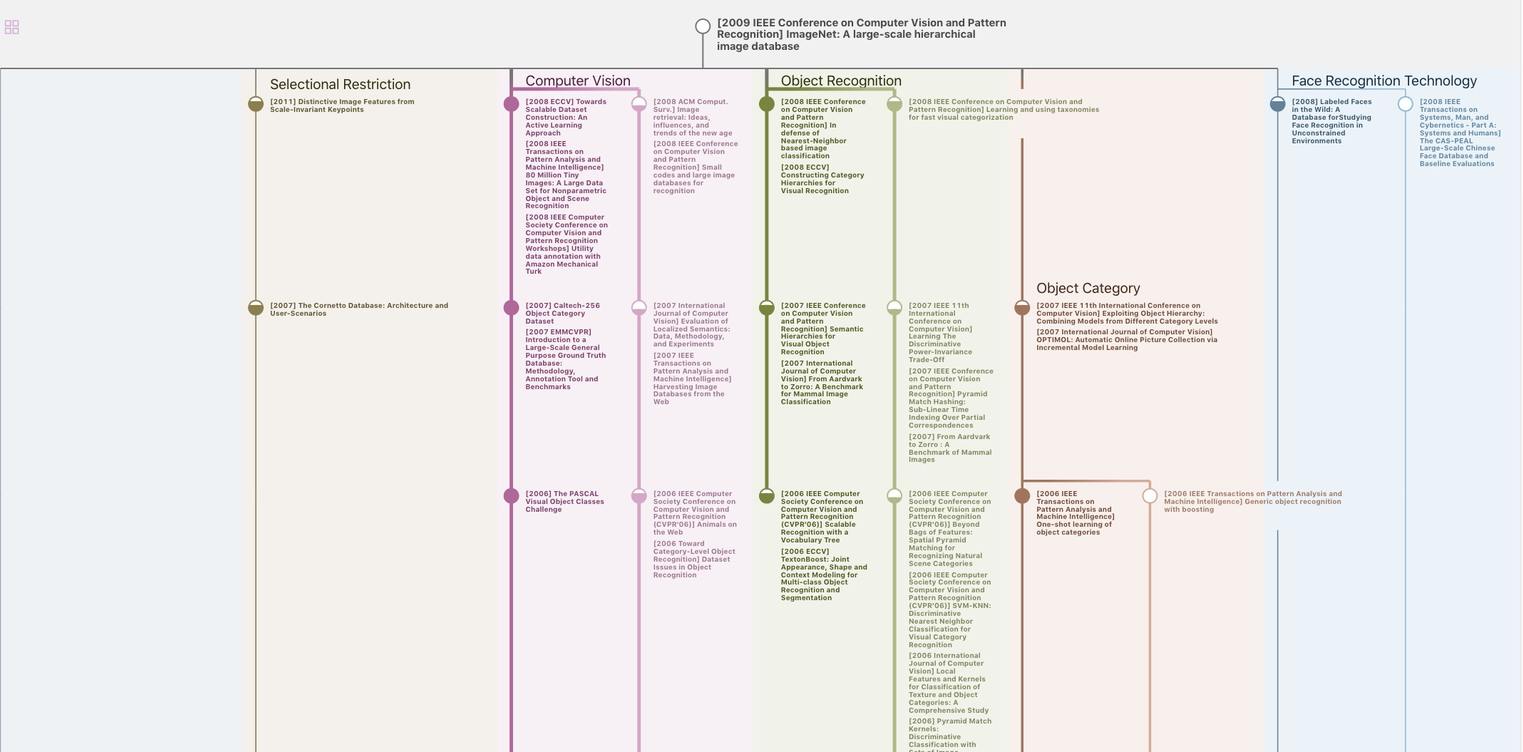
生成溯源树,研究论文发展脉络
Chat Paper
正在生成论文摘要