Computational Approaches for Green Computing of Energy Consumption Forecasting on Non-working Periods in an Office Building
ENERGY INFORMATICS, EI.A 2023, PT II(2024)
摘要
The energy management of electrical buildings takes an active role in the energy market. Researchers tasked with the primary goal of reducing the energy costs take advantage of machine learning algorithms to predict how much energy should be bought and sold in the market ahead of time. Some researchers take account green computing approaches to reduce the energy cost spent in forecasting roles and ensure the environmental sustainability of computing devices. In this paper two forecasting algorithms known as k-nearest neighbors and artificial neural networks train an annual historic with energy consumptions and sensors devices data to predict several energy consumption values of a target week for non-working periods of either five minutes or hour schedules. The green computing area is highlighted in this paper by studying the influence that decisions intended on decreasing the energy of the CPU processing unit on forecasting activities may have in the forecasting accuracy. Such decisions include changing the training and forecasting schedule of five minutes to hour periods and excluding the retraining using updated data from the test set. The conclusions of this paper clarify that scheduling forecasts for non-working hours with the support of k-nearest neighbors algorithm contextualized for periods of five minutes results in lower errors than artificial neural networks. However scheduling forecasts for periods of five minutes instead of hour periods also results in higher energy and time dedicated for cleaning, training, and forecasting tasks.
更多查看译文
关键词
environmental sustainability,forecasting algorithms,green computing
AI 理解论文
溯源树
样例
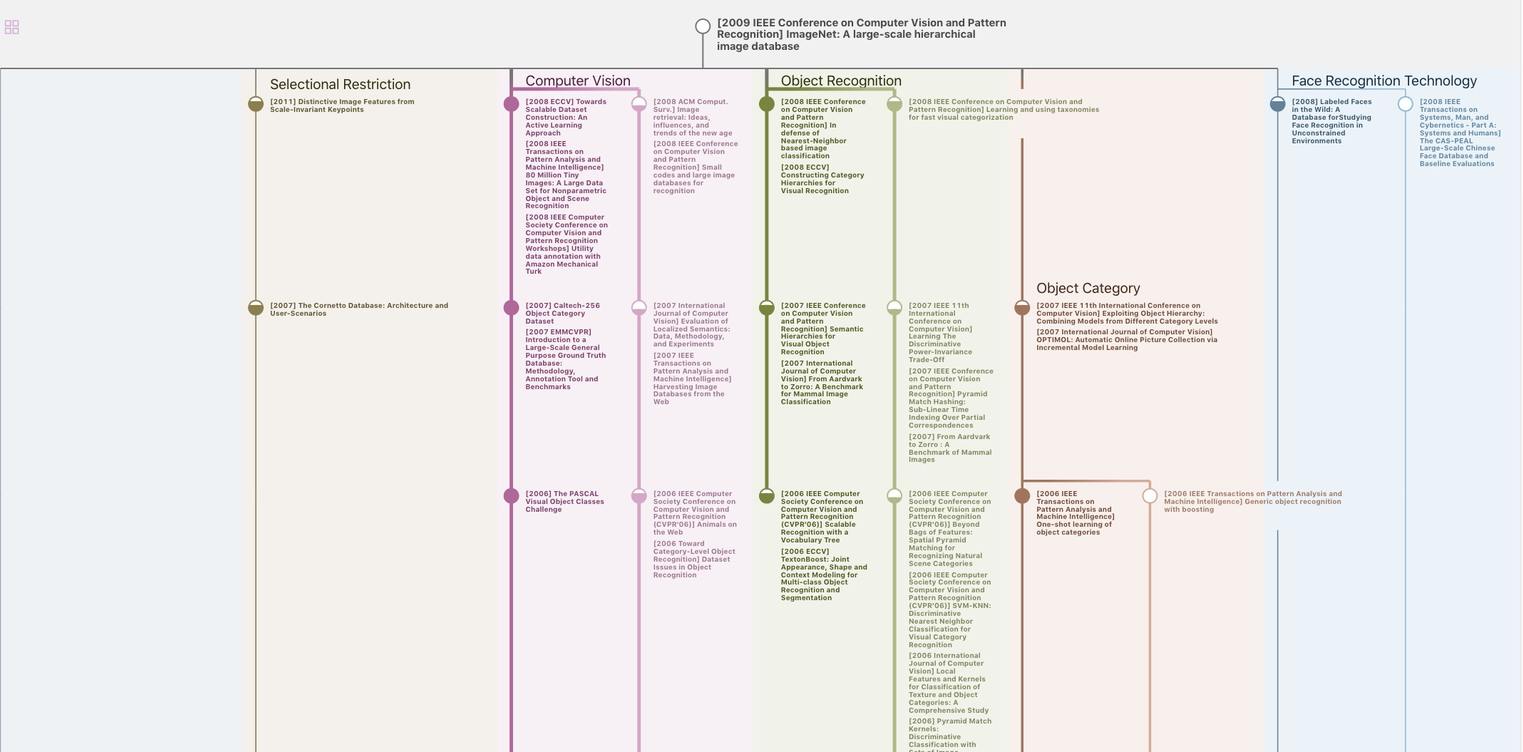
生成溯源树,研究论文发展脉络
Chat Paper
正在生成论文摘要