Advancing object recognition in visual blur conditions: a benchmark study
JOURNAL OF ELECTRONIC IMAGING(2023)
摘要
Recent studies have shown that deep neural networks are sensitive to visual blur. This vulnerability raises concerns about the robustness of learning-based methods in safety-critical applications, such as autonomous driving and industrial inspection. Although many methods have demonstrated the potential to enhance the robustness of vision tasks in blurry scenes, a standard benchmark for comparing and validating their effectiveness is still lacking. To address this, we propose a new benchmark for the comprehensive evaluation of visual recognition tasks in blurry scenes. The benchmark dataset includes a large number of scenes with different blur types, enabling multi-dimensional evaluation and comparison of existing methods. The existing methods are integrated into a unified evaluation framework for comparison, and the mechanism of robustness improvement is roughly categorized as reducing image-level shift and distribution-level shift. Through this unified framework, we identify the strengths and weaknesses of current methods in visual recognition tasks under blurry conditions. Furthermore, we propose a prior knowledge-based regularization module that can be easily incorporated into existing methods, which consistently boosts performance on visual recognition tasks in blurry scenes. (c) 2023 SPIE and IS&T
更多查看译文
关键词
object recognition,visual blur,unsupervised domain adaptation
AI 理解论文
溯源树
样例
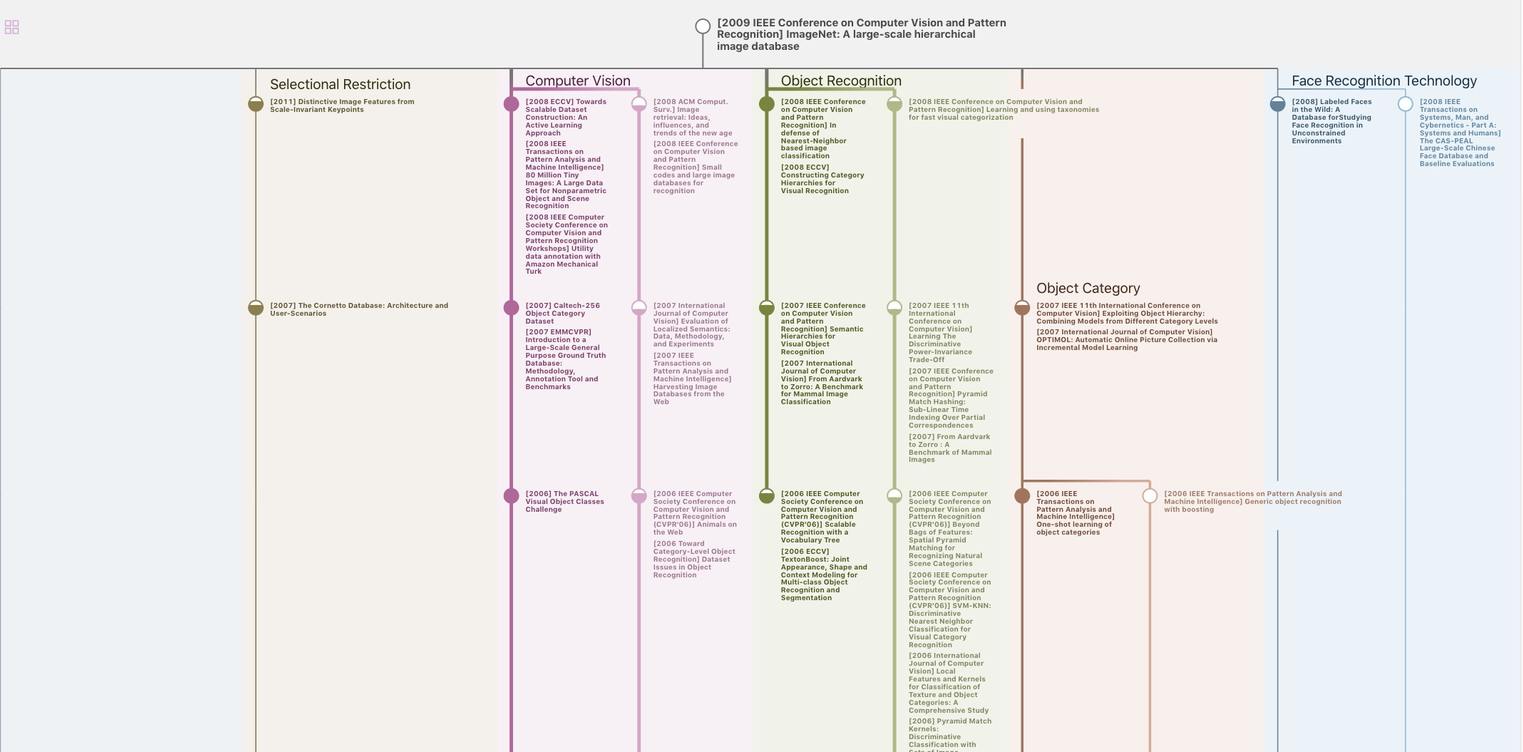
生成溯源树,研究论文发展脉络
Chat Paper
正在生成论文摘要