Camouflage Target Detection Based on Strong Semantic Information and Feature Fusion.
Journal of electronic imaging(2023)
摘要
Aiming at the detection difficulties in camouflage target detection, such as the high similarity between the target and its background, serious damage to the edge, and strong concealment of the target, a camouflage target detection algorithm YOLO of camouflage object detection based on strong semantic information and feature fusion is proposed. First, the attention mechanism convolutional block attention module (CBAM) is constructed to highlight the important channel features and target spatial locations to further aggregate rich semantic information from the high-level feature map. Then the atrous spatial pyramid pooling module is constructed to repeatedly sample the multiscale feature maps to expand the receptive field of the neural network, reduce feature sparsity in the process of convolution, and ensure dense features and multiscale contextual semantic information enter the feature fusion module. Finally, the attention skip-connections are constructed based on the CBAM module for fusing the original feature maps extracted by the backbone network to the corresponding detection outputs so as to eliminate the redundant features as well as enrich the target information of the network outputs. In order to fully verify the performance of the proposed algorithm, a camouflage target detection dataset named strong camouflage efficiency target dataset (SCETD) is constructed. Experimental results on SCETD show that the precision and recall of the proposed algorithm achieve 96.1% and 87.1%, respectively. The AP(0.5) and AP(0.5 : 0.95) achieve 92.3% and 54.4%, respectively. The experimental results prove the effectiveness of the proposed method in detecting camouflage targets.
更多查看译文
关键词
camouflage target,strong semantic information,feature fusion,attention mechanism,receptive field,convolutional neural network
AI 理解论文
溯源树
样例
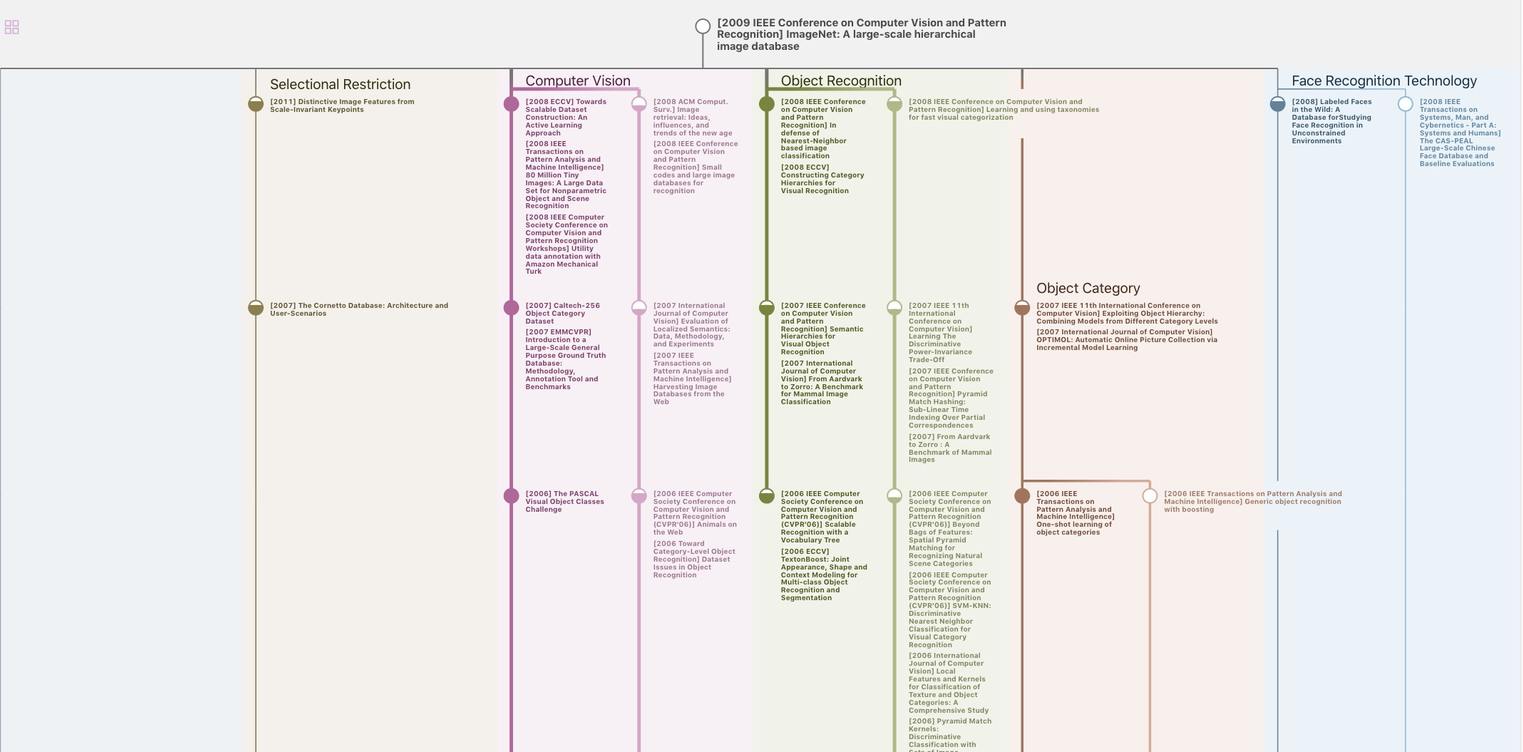
生成溯源树,研究论文发展脉络
Chat Paper
正在生成论文摘要