Learning and forgetting in systems neuroscience: A control perspective
ANNUAL REVIEWS IN CONTROL(2023)
摘要
A longstanding open problem of systems neuroscience is to understand how the brain calibrates thousands of reflexes to achieve near instantaneous disturbance rejection. While reflexes typically act locally at the site of sensory measurements, the adaptation of reflex gains is the result of an ingenious architecture in which knowledge of disturbances is transferred from the cerebellum to the deep cerebellar nuclei or the brainstem. This paper investigates the use of control theory as the mathematical foundation to explain the mechanisms by which such forms of learning, as well as forgetting, manifest themselves in systems neuroscience. Particularly, we use adaptive control and averaging theory to model the computations performed in learning appropriate reflex gains. While forgetting is perceived as counter-productive to learning, we show that if incorporated correctly, it can endow the much needed robustness to train thousands of reflexes without interfering with their adaptation. This is accomplished using the ??????-modification which achieves robustness of adaptive schemes through the estimation of exciting subspaces. Our techniques are combined in a comprehensive model, with simulations illustrating their effectiveness.
更多查看译文
关键词
Adaptive internal models,Averaging analysis,Cerebellum,Output regulation,Reflexes,Robust adaptive control
AI 理解论文
溯源树
样例
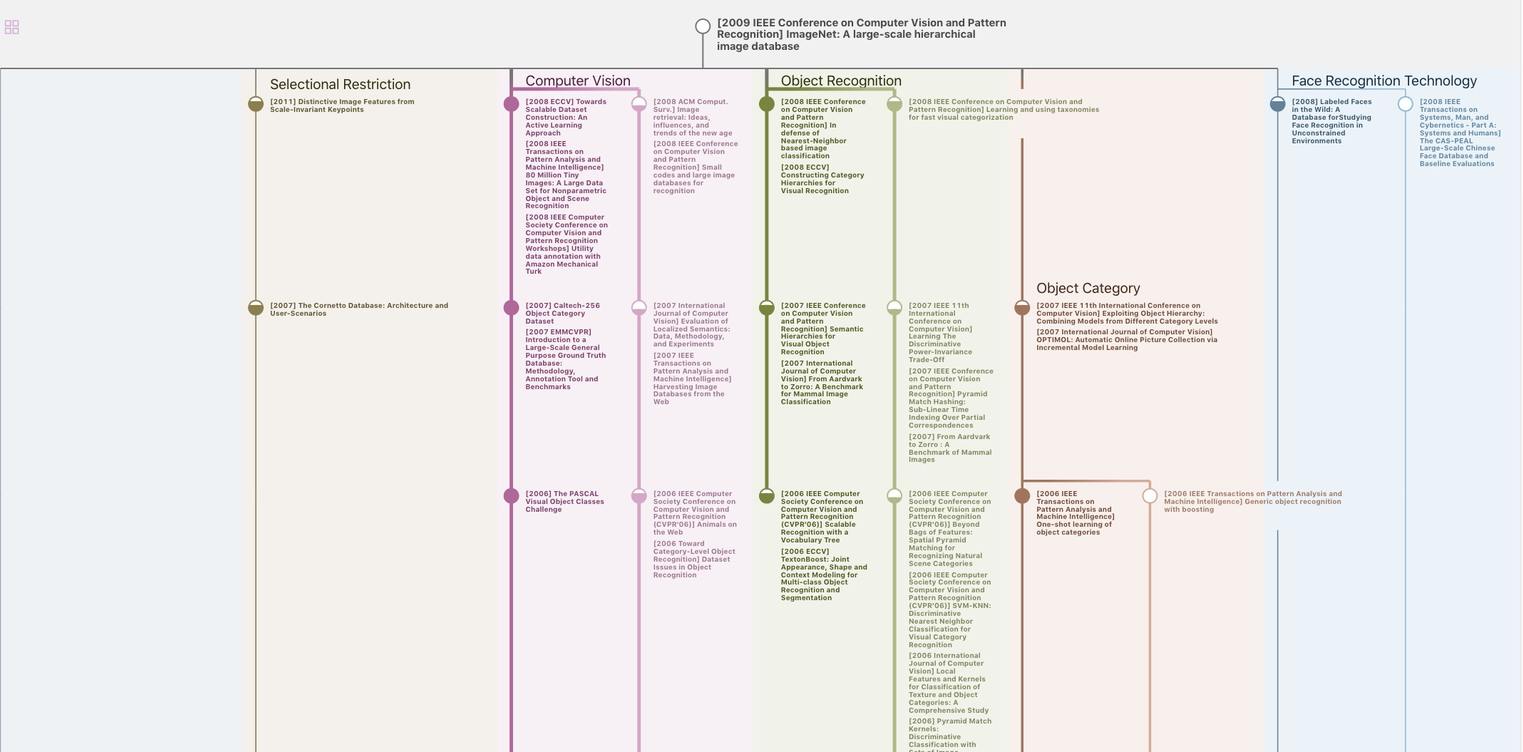
生成溯源树,研究论文发展脉络
Chat Paper
正在生成论文摘要