TEM1Dformer: A Novel 1-D Time Series Deep Denoising Network for TEM Signals
IEEE SENSORS JOURNAL(2024)
摘要
The transient electromagnetic method (TEM) is widely used in geophysical exploration tasks of minerals, goaves, and groundwater, but the received secondary induced electromotive force is susceptible to external noise interference and attenuation distortion. Deep learning has been used for transient electromagnetic signal denoising tasks and outperforms traditional algorithms. However, the existing convolutional neural network (CNN) has limitations in modeling the global time series relationships of the data. Transformer is vulnerable to the interference of redundant information in the time series data, and the computing power requirements are difficult to meet the needs of engineering deployment. To solve the above problems, this article proposes a new 1-D time series denoising framework. This framework adopts 1-D convolution and vision transformer (ViT) encoder architecture, which reduces the computational requirements of the Transformer, retains the local perception characteristics of the convolution network and the global perception characteristics of the transformer, and combines multitask loss function and dense connection residual structure to optimize the denoising performance of the model. The TEM signal dataset is constructed by selecting multiple types of noise and power model parameters. The simulation signal test shows that compared with other typical algorithms, this work achieves the best performance in the 1-D sequential denoising task for transient electromagnetic. The model denoising research is carried out with transient electromagnetic field signals collected from iron ore mine in western Shanxi Province, China. The results show that the accuracy of data interpretation is effectively improved, and the validity of the proposed model is verified.
更多查看译文
关键词
Noise reduction,Feature extraction,Transformers,Convolutional neural networks,Transient analysis,Task analysis,Time series analysis,1-D time series denoising,convolutional neural network (CNN),deep learning,transient electromagnetic,vision transformer (ViT)
AI 理解论文
溯源树
样例
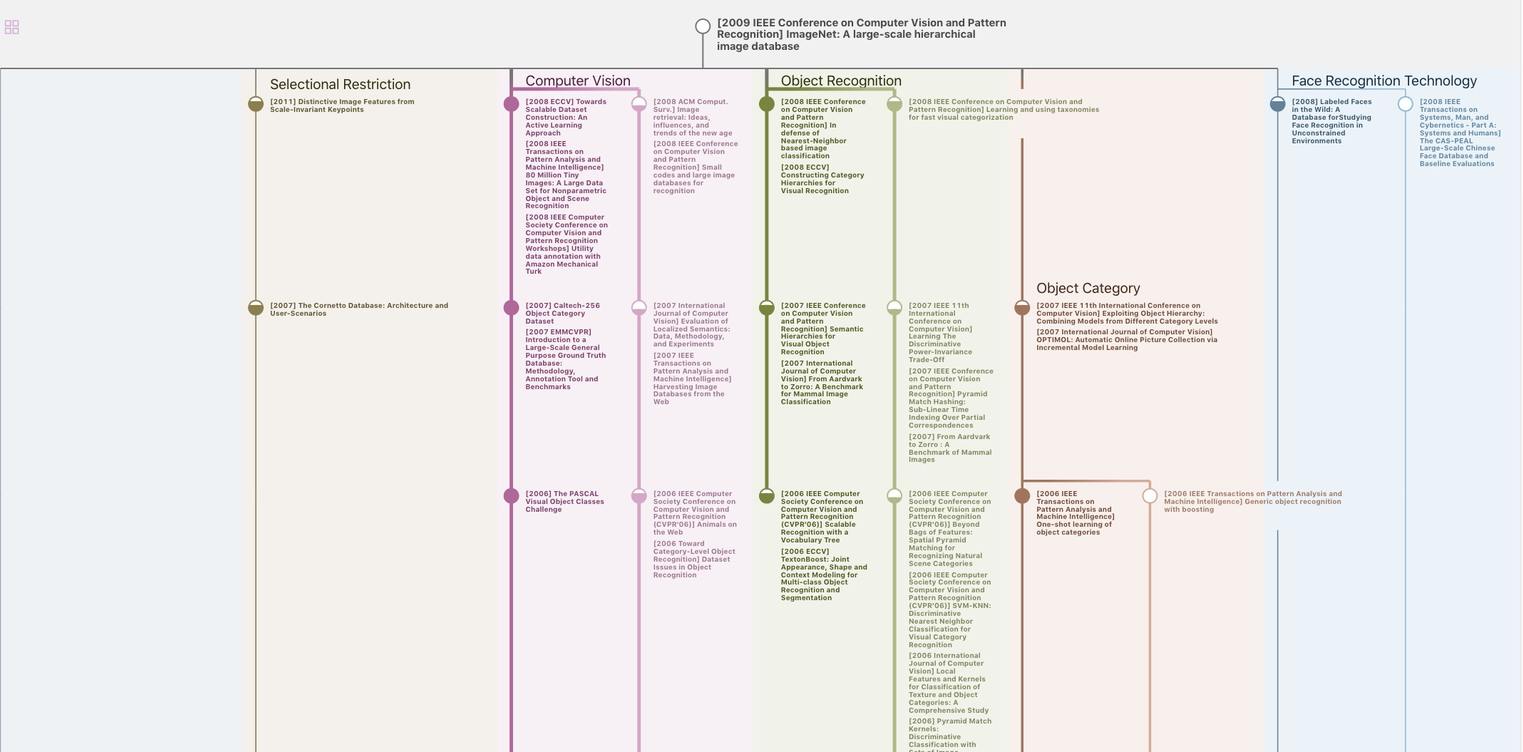
生成溯源树,研究论文发展脉络
Chat Paper
正在生成论文摘要