Research on Underground 3-D Displacement Measurement Based on Convolutional Neural Networks and Dual Mutual Inductance Voltages
IEEE SENSORS JOURNAL(2024)
摘要
Underground 3-D displacement monitoring plays an important role in landslide disaster early warning. Compared with surface displacement and underground 2-D displacement monitoring, it can provide more accurate early warning information. Previously, the measurement device of underground 3-D displacement based on dual-mutual inductance voltages was developed, which can collect millimeter-level displacement data. However, how to establish the relationship between mutual inductance voltages and underground 3-D displacement still faces huge challenges. In this work, the dual-mutual inductance voltages at each interaxis angle are treated as data maps. Then, they can serve as input to the convolutional neural network (CNN)-based model (ConvRnet), which possess powerful image data mining capabilities. The experiment results show that the ConvRnet model significantly improves the prediction accuracy compared with the interpolation model and other machine learning models. The maximum errors of horizontal displacement and vertical displacement are 0.42 and 0.32 mm, respectively. The ConvRnet model combined with the data maps of dual mutual inductance voltages provides an effective tool for underground 3-D displacement measurement.
更多查看译文
关键词
3-D measurement,attention mechanism,convolutional neural networks,underground displacement monitoring of landslide
AI 理解论文
溯源树
样例
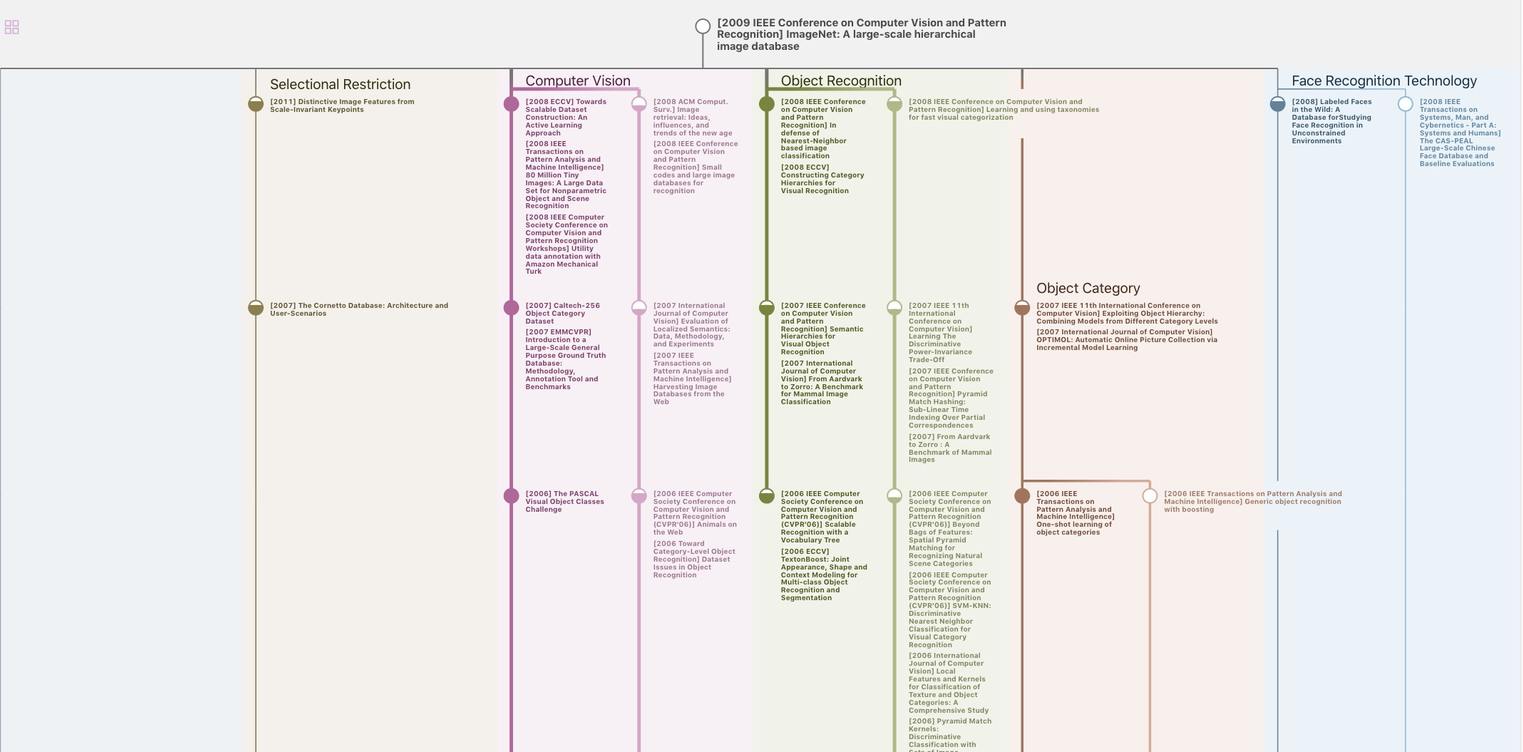
生成溯源树,研究论文发展脉络
Chat Paper
正在生成论文摘要