Multiassistant Knowledge Distillation for Lightweight Bearing Fault Diagnosis Based on Decreasing Threshold Channel Pruning
IEEE SENSORS JOURNAL(2024)
摘要
Bearing fault detection and classification under a diagnostics model with fewer parameters has been a challenging problem. A common solution is knowledge distillation (KD) using teacher-student models. Through the distillation process, the student model can acquire knowledge from the teacher model to enhance performance without introducing extra parameters. However, when using a powerful teacher model, distillation performance is not always ideal. This is because a more powerful teacher model can generate more specific classification strategies, which may result in poorer distillation performance. To this end, the multiassistant KD (MAKD) method is proposed, which bridges the gap between the teacher-student models by incorporating several intermediate-sized assistant models (AMs). Moreover, these AMs have the same architecture, which creates a better knowledge transfer condition at the logit layer. To further optimize the network structure to improve the distillation performance, decreasing threshold channel pruning (DTCP) is proposed to generate better AMs. DTCP leverages the scatter value of the decreasing function to prune the channels of the teacher model, which retains more channels that are beneficial to distillation. Finally, four-class and ten-class classification experiments are conducted on two bearing datasets. The experimental results demonstrate that the proposed DTCP-MAKD method improves distillation performance and outperforms other state-of-the-art KD methods.
更多查看译文
关键词
Channel pruning,intelligent fault diagnosis,knowledge distillation (KD),wavelet transform
AI 理解论文
溯源树
样例
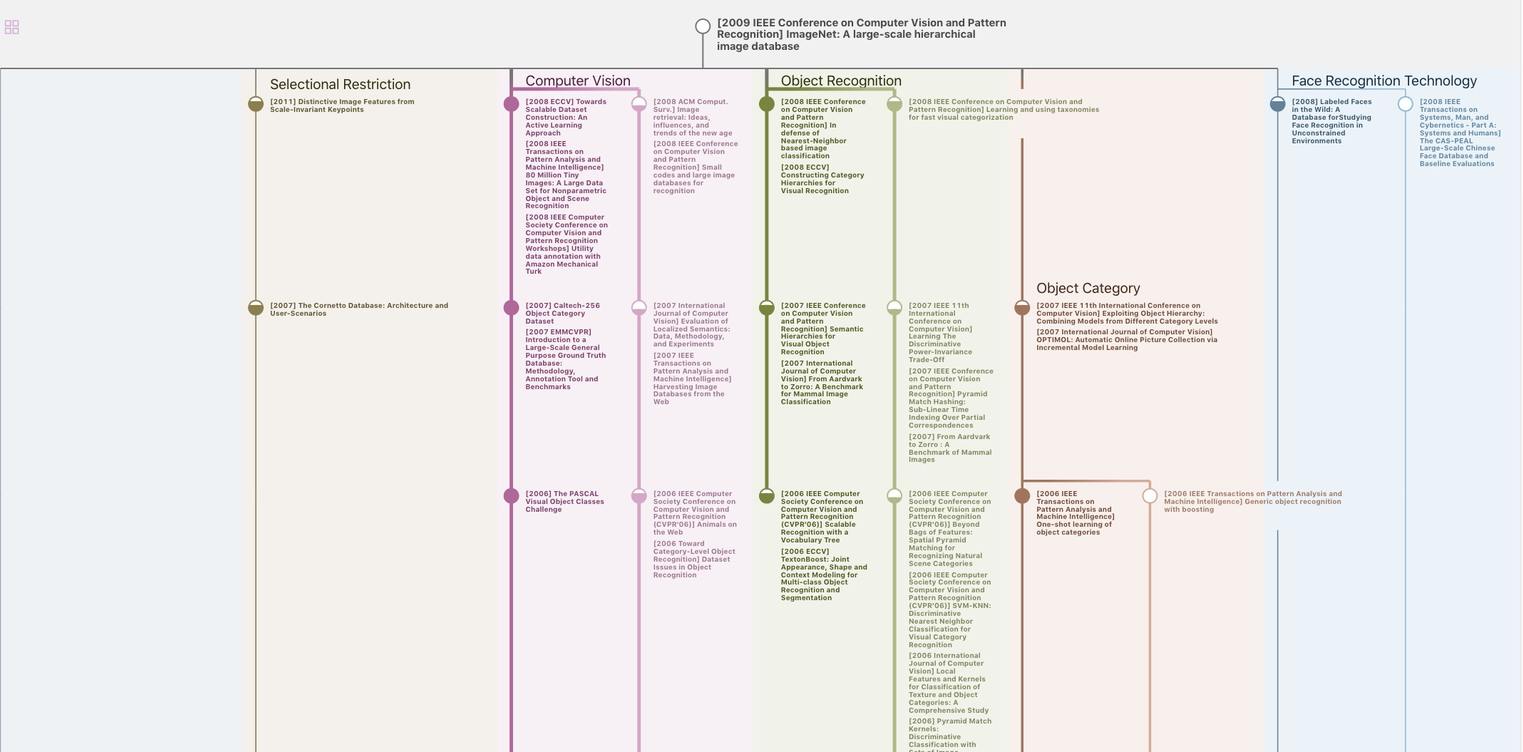
生成溯源树,研究论文发展脉络
Chat Paper
正在生成论文摘要