Online Identification of Payload Inertial Parameters Using Ensemble Learning for Collaborative Robots
IEEE ROBOTICS AND AUTOMATION LETTERS(2024)
摘要
Collaborative robots (Cobots) are essential in flexible automation solutions, enabling fast and easy reconfiguration to adapt to varying task requirements in dynamic environments. This requires the ability to safely handle different payloads with varying inertial parameters, which may not be known in advance. Hence, online identification of the payload's inertial parameters becomes essential for safe interactions, accurate path following, and stable grasping. Most existing methods require additional sensors, calibration procedures, or custom filtering, which increases the complexity and estimation time. In this letter, we propose a novel online identification method that employs a bagging ensemble machine learning approach to identify the payload inertial parameters without external sensors or additional filtering and calibration steps. The method uses available joint position, velocity, and torque measurements from the Cobot to train neural networks and decision trees as weak learners. The method is tested in simulation and validated using the Franka Emika Panda Cobot. The results showed that our method outperforms the state-of-the-art recursive least square methods reducing prediction errors by 75%-78% for mass and 49.5%-60% for the center of mass, while estimating accurate payload parameters within the first time step.
更多查看译文
关键词
In-Hand manipulation,online identification,payload dynamics
AI 理解论文
溯源树
样例
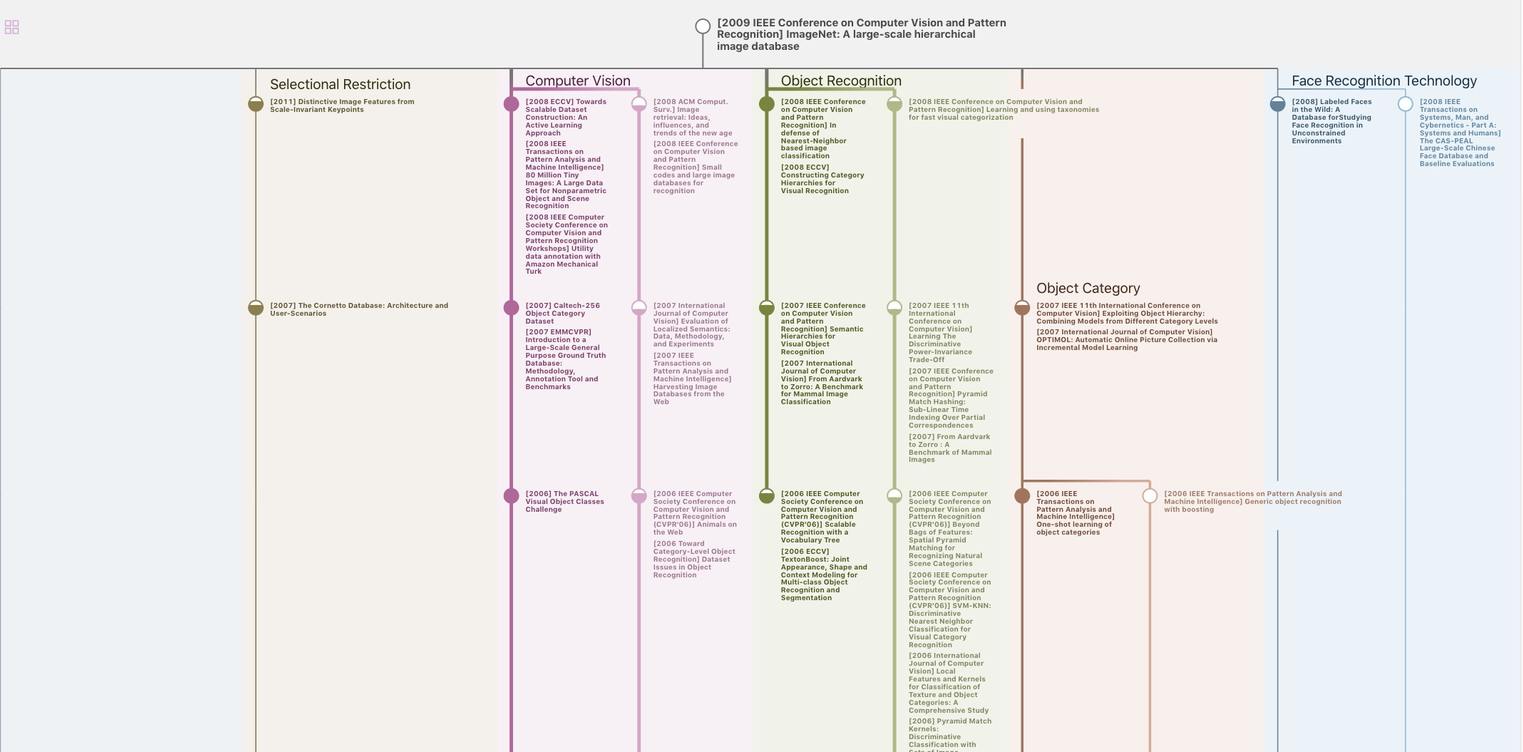
生成溯源树,研究论文发展脉络
Chat Paper
正在生成论文摘要