A2-CLM: Few-Shot Malware Detection Based on Adversarial Heterogeneous Graph Augmentation
IEEE TRANSACTIONS ON INFORMATION FORENSICS AND SECURITY(2024)
摘要
Malware attacks, especially "few-shot" malware, have profoundly harmed the cyber ecosystem. Recently, malware detection models based on graph neural networks have achieved remarkable success. However, these efforts over-rely on sufficient labeled data for model training and thus may be brittle in few-shot malware detection because of the label scarcity. To this end, we propose a self-supervised malware detection framework based on graph contrastive learning and adversarial augmentation, termed A2-CLM, to address the challenge of few-shot malware detection. Particularly, A2-CLM first depicts the malware execution context with a sensitivity heterogeneous graph by assessing the security semantic of each behavior. Afterwards, A2-CLM designs multiple adversarial attacks to generate more practical contrastive pairs, including the PGD attack, attribute masking attack, meta-graph-guide sampling attack, direct system calls attack, and obfuscation attack, which is beneficial to strengthening the model's effectiveness and robustness. To alleviate the training workload of contrastive learning, we introduce a momentum strategy to train the multiple graph encoders in A2-CLM. Especially on 1-shot detection tasks, A2-CLM achieves performance gains of up to 24.63% and 4.58% against supervised and self-supervised detection methods, respectively.
更多查看译文
关键词
Malware,Behavioral sciences,Task analysis,Sensitivity,Semantics,Feature extraction,Training,Few-shot malware detection,security semantic,graph contrastive learning,adversarial augmentation
AI 理解论文
溯源树
样例
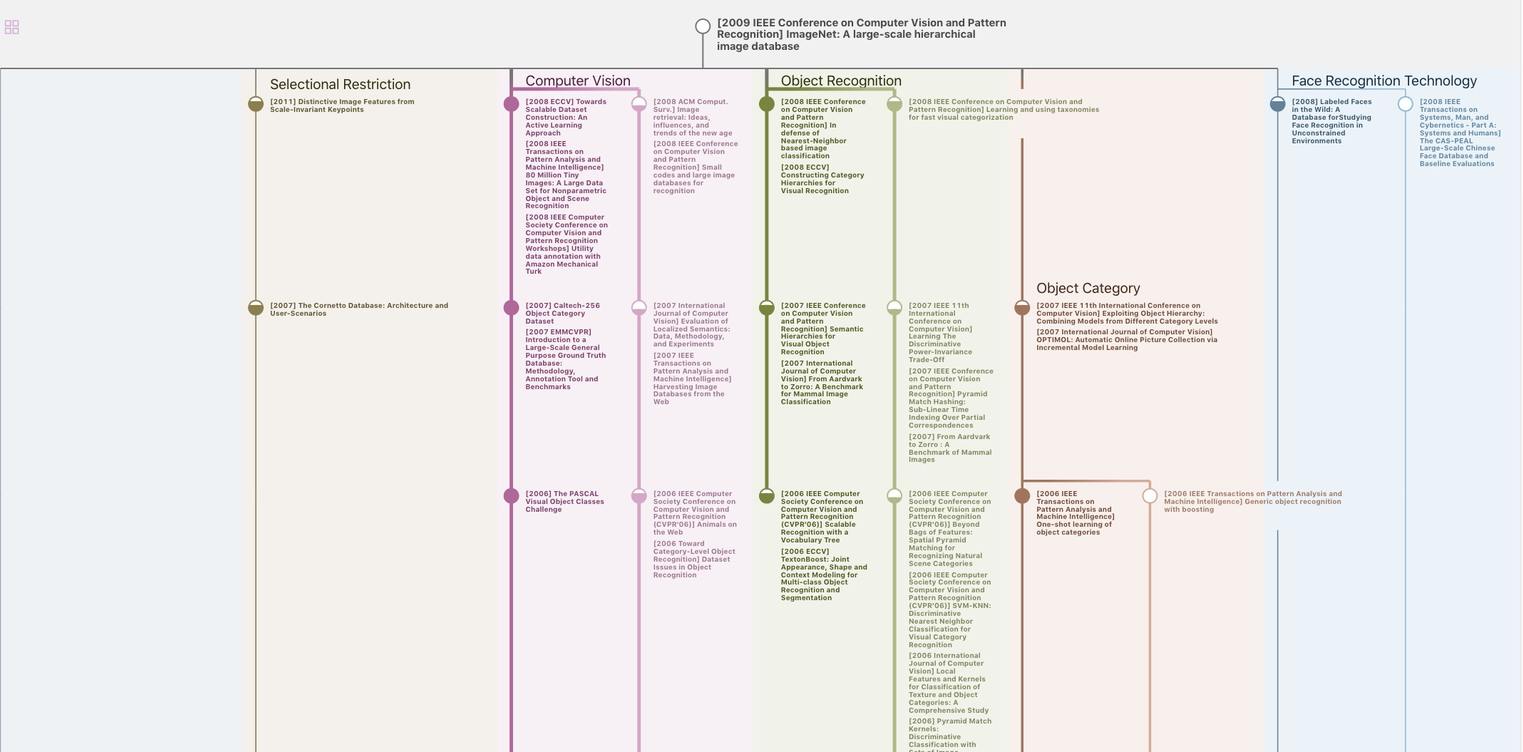
生成溯源树,研究论文发展脉络
Chat Paper
正在生成论文摘要