Are Central Eating Disorder Network Symptoms Sensitive to Item Selection and Sample? Implications for Conceptualization of Eating Disorder Psychopathology From a Network Perspective
JOURNAL OF PSYCHOPATHOLOGY AND CLINICAL SCIENCE(2024)
摘要
Item selection is a critical decision in modeling psychological networks. The current preregistered two-study research used random selections of 1,000 symptom networks to examine which eating disorder (ED) and co-occurring symptoms are most central in longitudinal networks among individuals with EDs (N= 71, total observations = 6,060) and tested whether centrality changed based on which items were included in the network. Participants completed 2 weeks of ecological momentary assessment (five surveys/day). In Study 1, we obtained initial strength centrality values by estimating an a priori network using eight items with the highest means. We then estimated 1,000 networks and their centrality from a random selection of unique eight-item symptom combinations. We compared the strength centrality from the a priori network to the distribution of strength centrality estimates from the random-item networks. In Study 2, we repeated this procedure in an independent longitudinal dataset (N= 41, total observations = 4,575) to determine if our results generalized across samples. Shame, guilt, worry, and fear of losing control were consistently central across networks, regardless of items included in the network or sample. Results suggest that these symptoms may be important to the structure of ED psychopathology and have implications for how we understand the structure of ED psychopathology. Existing methods for item inclusion in psychological networks may distort the structure of ED symptom networks by either under-or overestimating strength centrality, or by omitting consistently central symptoms that are nontraditional ED symptoms. Future research should consider including these symptoms in models of ED psychopathology.
更多查看译文
关键词
eating disorders,network analysis,network theory,ecological momentary assessment,intensive longitudinal data
AI 理解论文
溯源树
样例
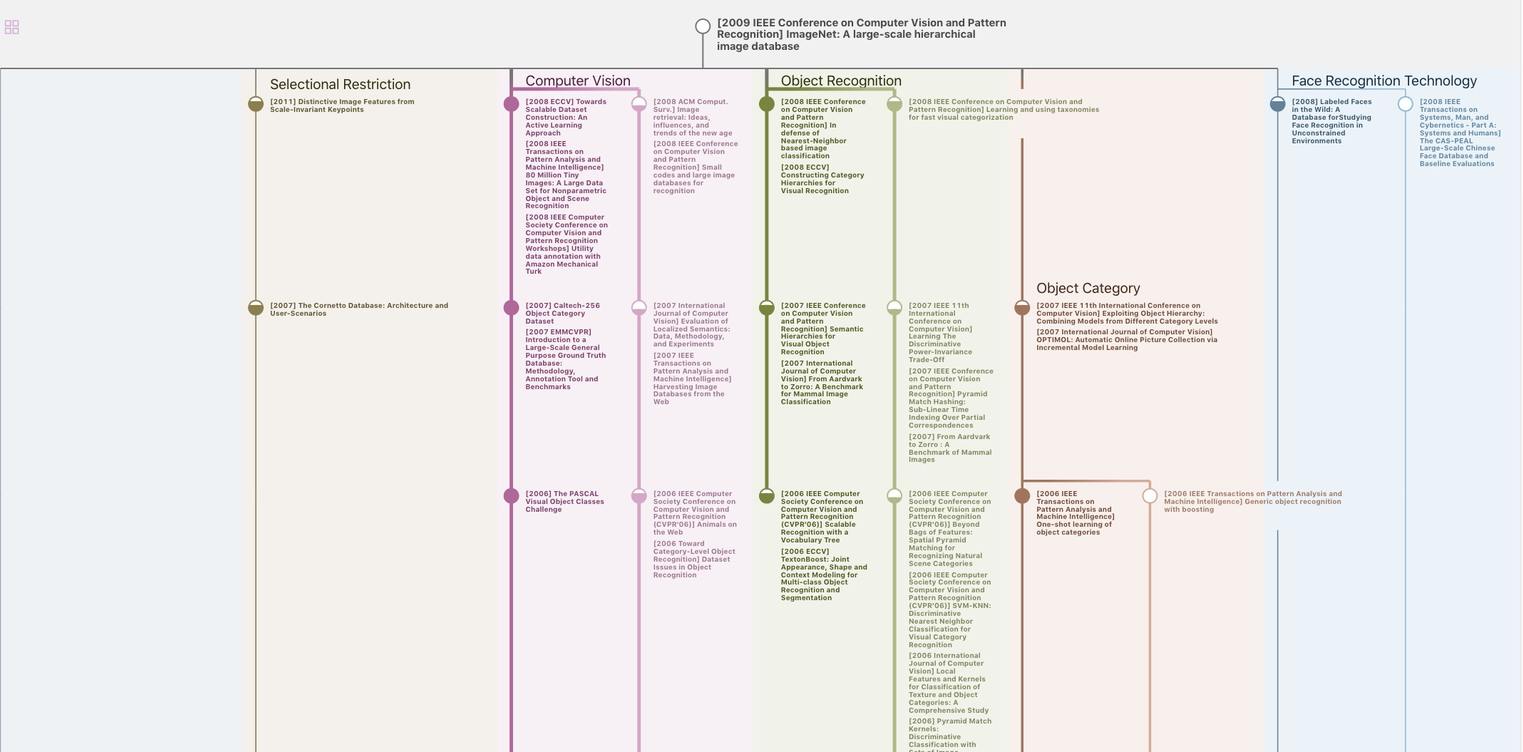
生成溯源树,研究论文发展脉络
Chat Paper
正在生成论文摘要