Depth map super-resolution via learned nonlocal model and enhanced local regularization
SIGNAL PROCESSING(2024)
摘要
The appearance of consumer depth cameras makes it easy to obtain depth maps of a scene. However, the quality of depth maps is low and cannot satisfy the practical application. This paper proposes a novel depth map superresolution method, which combines the advantages of the learning-based method and the reconstruction-based method, and incorporates both the learned nonlocal self-similarity prior and enhanced local geometry prior. On one hand, the nonlocal self-similarity prior is learned from the external examples by the patch group-based Gaussian mixture model (PG-GMM) and then used to explore the nonlocal self-similarity of depth maps. On the other hand, the local geometry prior is explored in both the gradient and spatial domain. For the gradient domain, the hyper-Laplacian prior is introduced to model the gradient distribution. For the spatial domain, a structure similarity-based auto-regressive model is proposed to acquire local correlation in a small area of a depth map. Finally, the learned nonlocal and enhanced local prior are cast into a unified optimization framework to acquire an estimation of the high-resolution image. Compared with state-of-the-art methods, experimental results indicate that our method can achieve better reconstruction performance in terms of both quantitative and qualitative evaluations.
更多查看译文
关键词
Depth map super-resolution,Learned nonlocal self-similarity,Enhanced local prior,Structure similarity,Hyper-Laplacian
AI 理解论文
溯源树
样例
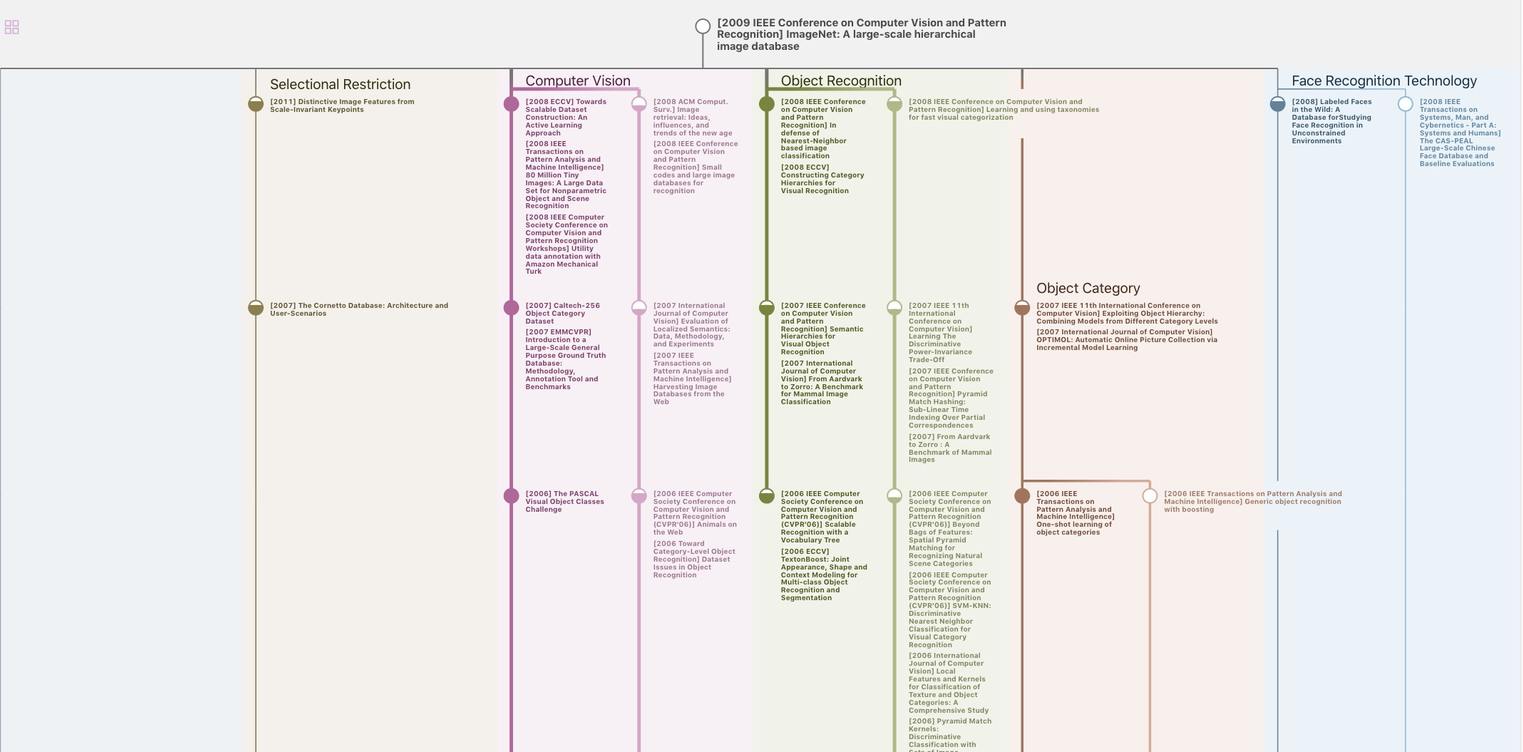
生成溯源树,研究论文发展脉络
Chat Paper
正在生成论文摘要