Parameter-Free and Scalable Incomplete Multiview Clustering With Prototype Graph
IEEE TRANSACTIONS ON NEURAL NETWORKS AND LEARNING SYSTEMS(2024)
摘要
Multiview clustering (MVC) seamlessly combines homogeneous information and allocates data samples into different communities, which has shown significant effectiveness for unsupervised tasks in recent years. However, some views of samples may be incomplete due to unfinished data collection or storage failure in reality, which refers to the so-called incomplete multiview clustering (IMVC). Despite many IMVC pioneer frameworks have been introduced, the majority of their approaches are limited by the cubic time complexity and quadratic space complexity which heavily prevent them from being employed in large-scale IMVC tasks. Moreover, the massively introduced hyper-parameters in existing methods are not practical in real applications. Inspired by recent unsupervised multiview prototype progress, we propose a novel parameter-free and scalable incomplete multiview clustering framework with the prototype graph termed PSIMVC-PG to solve the aforementioned issues. Different from existing full pair-wise graph studying, we construct an incomplete prototype graph to flexibly capture the relations between existing instances and discriminate prototypes. Moreover, PSIMVC-PG can directly obtain the prototype graph without pre-process of searching hyper-parameters. We conduct massive experiments on various incomplete multiview tasks, and the performances show clear advantages over existing methods. The code of PSIMVC-PG can be publicly downloaded at https://github.com/wangsiwei2010/PSIMVC-PG.
更多查看译文
关键词
Prototypes,Task analysis,Complexity theory,Microwave integrated circuits,Kernel,Clustering algorithms,Indexes,Incomplete multiview learning,multiple graph clustering,prototype graph clustering
AI 理解论文
溯源树
样例
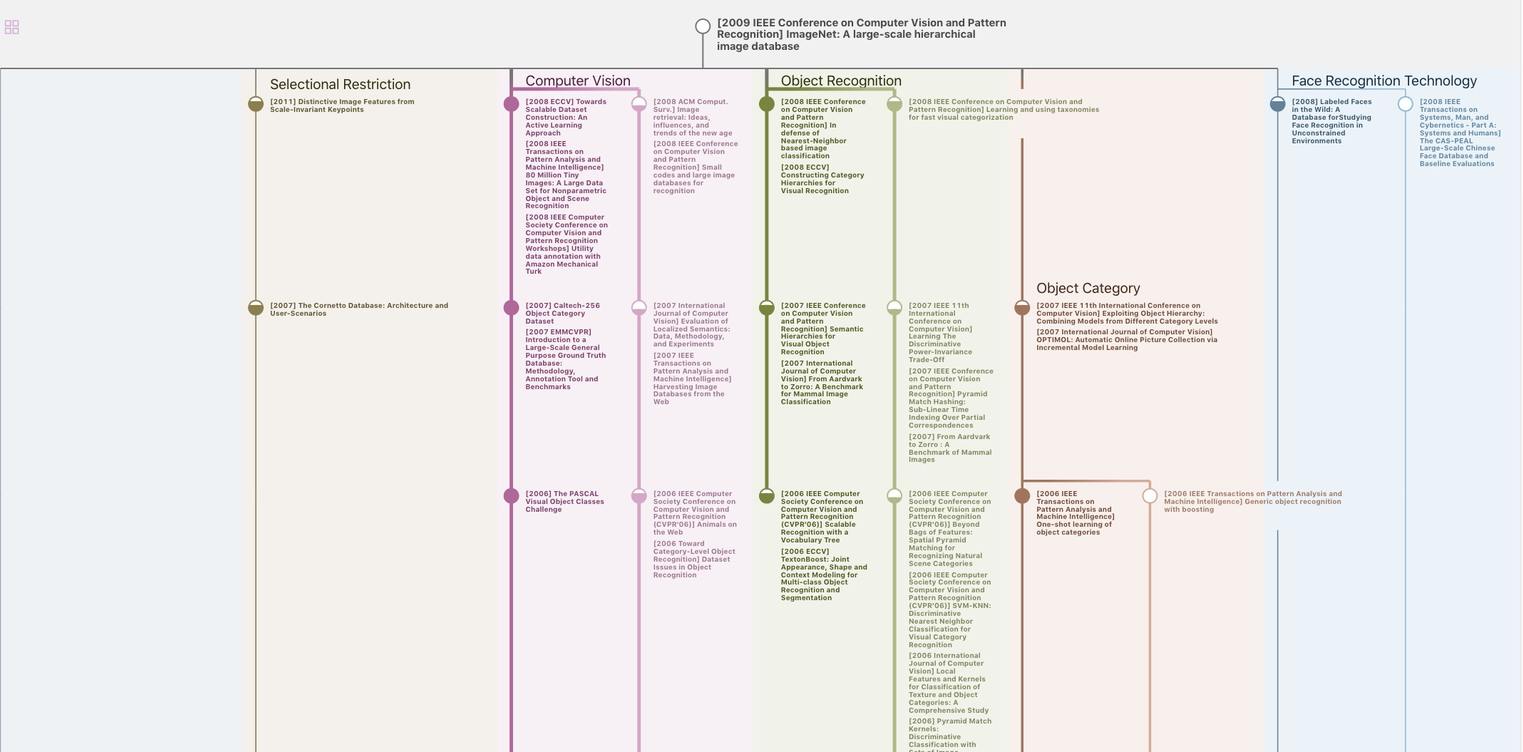
生成溯源树,研究论文发展脉络
Chat Paper
正在生成论文摘要