Data-driven coordinated attention deep learning for high-fidelity brain imaging denoising and inpainting
JOURNAL OF BIOPHOTONICS(2024)
摘要
Deep learning offers promise in enhancing low-quality images by addressing weak fluorescence signals, especially in deep in vivo mouse brain imaging. However, current methods struggle with photon scarcity and noise within in vivo deep mouse brains, and often neglecting tissue preservation. In this study, we propose an innovative in vivo cortical fluorescence image restoration approach, combining signal enhancement, denoising, and inpainting. We curated a deep brain cortical image dataset and developed a novel deep brain coordinate attention restoration network (DeepCAR), integrating coordinate attention with optimized residual networks. Our method swiftly and accurately restores deep cortex images exceeding 800 mu m, preserving small-scale tissue structures. It boosts the peak signal-to-noise ratio (PSNR) by 6.94 dB for weak signals and 11.22 dB for large noisy images. Crucially, we validate the effectiveness on external datasets with diverse noise distributions, structural features compared to those in our training data, showcasing real-time high-performance image restoration capabilities. This paper introduces an image restoration method proficient in denoising intense noise and amplifying weak photon-limited signals within in vivo mouse brain imaging. Meanwhile, it addresses imaging defects from fluorescent dyes in vascular imaging, preserving tissue structural integrity.image
更多查看译文
关键词
deep brain imaging,deep learning,image restoration,multiphoton microscopy
AI 理解论文
溯源树
样例
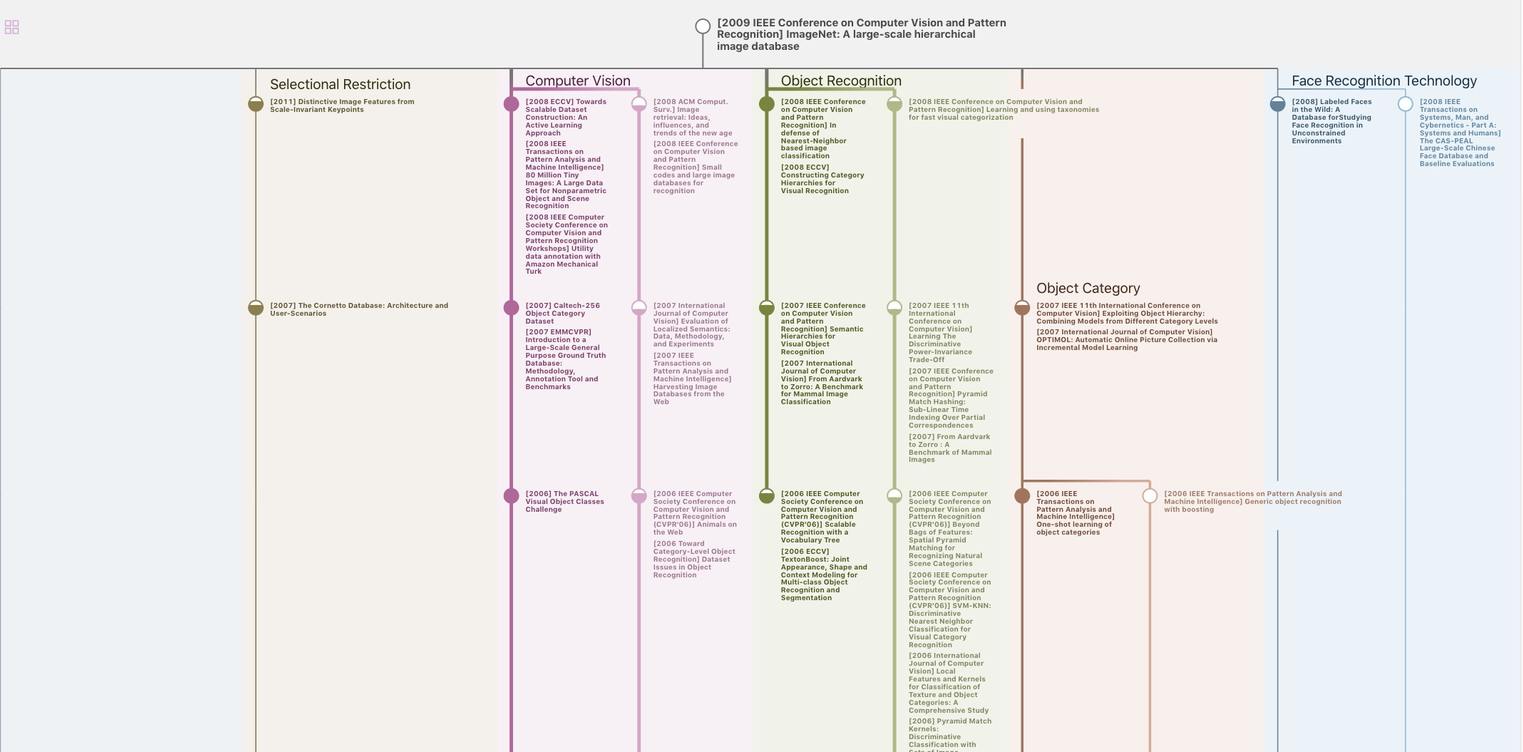
生成溯源树,研究论文发展脉络
Chat Paper
正在生成论文摘要