Predicting adolescent suicidal behavior following inpatient discharge using structured and unstructured data
JOURNAL OF AFFECTIVE DISORDERS(2024)
摘要
Background: The objective was to develop and assess performance of an algorithm predicting suicide -related ICD codes within three months of psychiatric discharge. Methods: This prognostic study used a retrospective cohort of EHR data from 2789 youth (12 to 20 years old) hospitalized in a safety net institution in the Northeastern United States. The dataset combined structured data with unstructured data obtained through natural language processing of clinical notes. Machine learning approaches compared gradient boosting to random forest analyses. Results: Area under the ROC and precision -recall curve were 0.88 and 0.17, respectively, for the final Gradient Boosting model. The cutoff point of the model -generated predicted probabilities of suicide that optimally classified the individual as high risk or not was 0.009. When applying the chosen cutoff (0.009) to the hold -out testing set, the model correctly identified 8 positive cases out of 10, and 418 negative cases out 548. The corresponding performance metrics showed 80 % sensitivity, 76 % specificity, 6 % PPV, 99 % NPV, F-1 score of 0.11, and an accuracy of 76 %. Limitations: The data in this study comes from a single health system, possibly introducing bias in the model's algorithm. Thus, the model may have underestimated the incidence of suicidal behavior in the study population. Further research should include multiple system EHRs. Conclusions: These performance metrics suggest a benefit to including both unstructured and structured data in design of predictive algorithms for suicidal behavior, which can be integrated into psychiatric services to help assess risk.
更多查看译文
关键词
Suicide,Adolescence,Risk,Patient discharge,Machine learning,Electronic health records
AI 理解论文
溯源树
样例
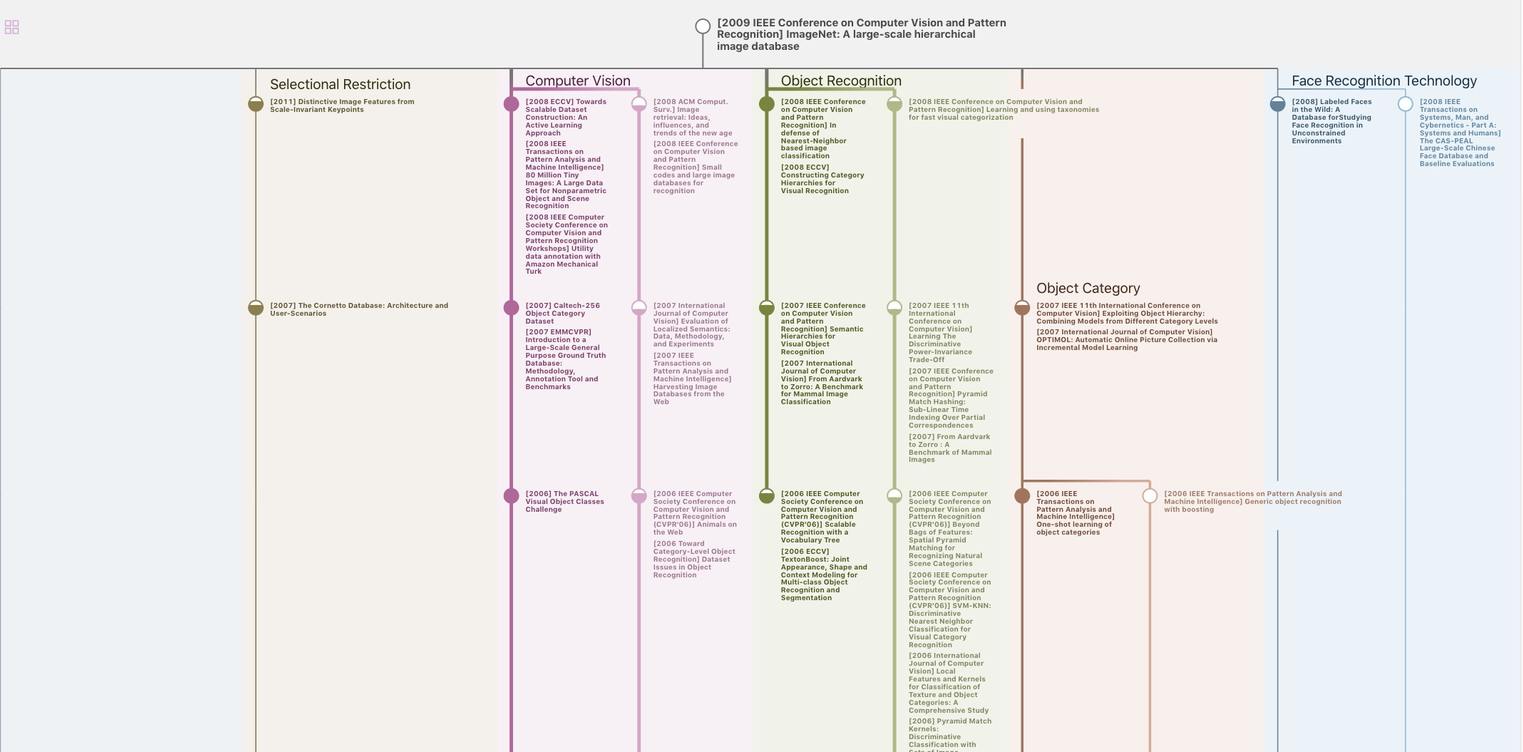
生成溯源树,研究论文发展脉络
Chat Paper
正在生成论文摘要