Using Artificial Intelligence to Gauge Competency on a Novel Laparoscopic Training System
JOURNAL OF SURGICAL EDUCATION(2024)
摘要
OBJECTIVE: Laparoscopic surgical skill assessment and machine learning are often inaccessible to low -and -middle -income countries (LMIC). Our team developed a low-cost laparoscopic training system to teach and assess psychomotor skills required in laparoscopic salpingostomy in LMICs. We performed video review using AI to assess global surgical techniques. The objective of this study was to assess the validity of artificial intelligence (AI) generated scoring measures of laparoscopic simulation videos by comparing the accuracy of AI results to human -generated scores. DESIGN: Seventy-four surgical simulation videos were collected and graded by human participants using a modified OSATS (Objective Structured Assessment of Technical Skills). The videos were then analyzed via AI using 3 different time and distance -based calculations of the laparoscopic instruments including path length, dimensionless jerk, and standard deviation of tool position. Predicted scores were generated using 5 -fold cross validation and K -Nearest -Neighbors to train classifiers. SETTING: Surgical novices and experts from a variety of hospitals in Ethiopia, Cameroon, Kenya, and the United States contributed 74 laparoscopic salpingostomy simulation videos. RESULTS: Complete accuracy of AI compared to human assessment ranged from 65-77%. There were no statistical differences in rank mean scores for 3 domains, Flow of Operation, Respect for Tissue, and Economy of Motion, while there were significant differences in ratings for Instrument Handling, Overall Performance, and the total summed score of all 5 domains (Summed). Estimated effect sizes were all less than 0.11, indicating very small practical effect. Estimated intraclass correlation coefficient (ICC) of Summed was 0.72 indicating moderate correlation between AI and Human scores. CONCLUSIONS: Video review using AI technology of global characteristics was similar to that of human review in our laparoscopic training system. Machine learning may help fill an educational gap in LMICs where direct apprenticeship may not be feasible. ( J Surg Ed 81:267-274. (c) 2023 Association of Program Directors in Surgery. Published by Elsevier Inc. All rights reserved.) ABBREVIATIONS: ALL -SAFE, African Laparoscopic Learners -Safe Advancement For Ectopic pregnancy AI, artificial intelligence CBME, competency -based medical education HIC, high -income country ICC, intraclass correlation coefficient KNN, K -Nearest Neighbors LOOCV, leave -one -out cross validation LMIC, low -and -middleincome country OSATS, Objective Structured Assessment of Technical Skills PAACS Pan-African Academy of Christian Surgeons
更多查看译文
关键词
global surgery,laparoscopic salpingos- tomy,surgical education,artificial intelligence,machine learning
AI 理解论文
溯源树
样例
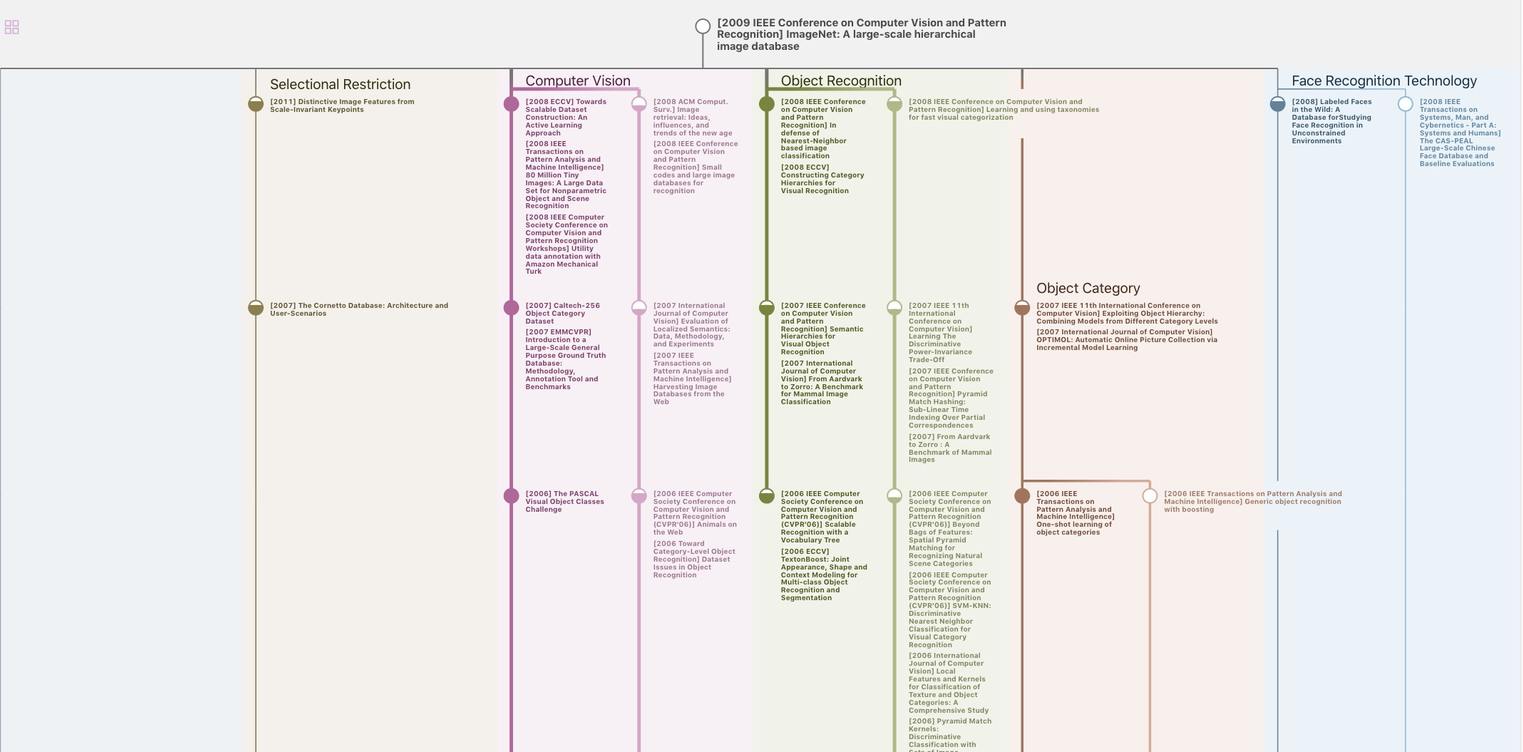
生成溯源树,研究论文发展脉络
Chat Paper
正在生成论文摘要