Self-Learning Takagi-Sugeno Fuzzy Control With Application to Semicar Active Suspension Model
IEEE TRANSACTIONS ON FUZZY SYSTEMS(2024)
摘要
In this article, we investigate the optimal control problem for semicar active suspension systems (SCASSs). First, we model the SCASSs by Newtonian dynamics as well as considering the uncertainties and nonlinear dynamics of the actuator. Second, in order to solve the complexity brought by uncertainties, we apply the Takagi-Sugeno (T-S) fuzzy approach to transform the SCASSs as multilinear systems, as well as solving the optimal control problem as a zero-sum problem to find the solution of Nash-equilibrium. Third, we construct a novel self-learning method based on the reinforcement learning framework, and propose two algorithms to solve the fuzzy game algebraic Riccati equation. Especially, in the second algorithm, without using any model information of the SCASSs, we only use the state and input information in control design by a self-learning manner removing the traditional dependence problem, which is more preferable for practical applications. Finally, we give a simulation result of the SCASSs to demonstrate the effectiveness and practicability for the designed self-learning algorithms.
更多查看译文
关键词
Fuzzy control,reinforcement learning (RL),self-learning,semicar active suspension,zero-sum game
AI 理解论文
溯源树
样例
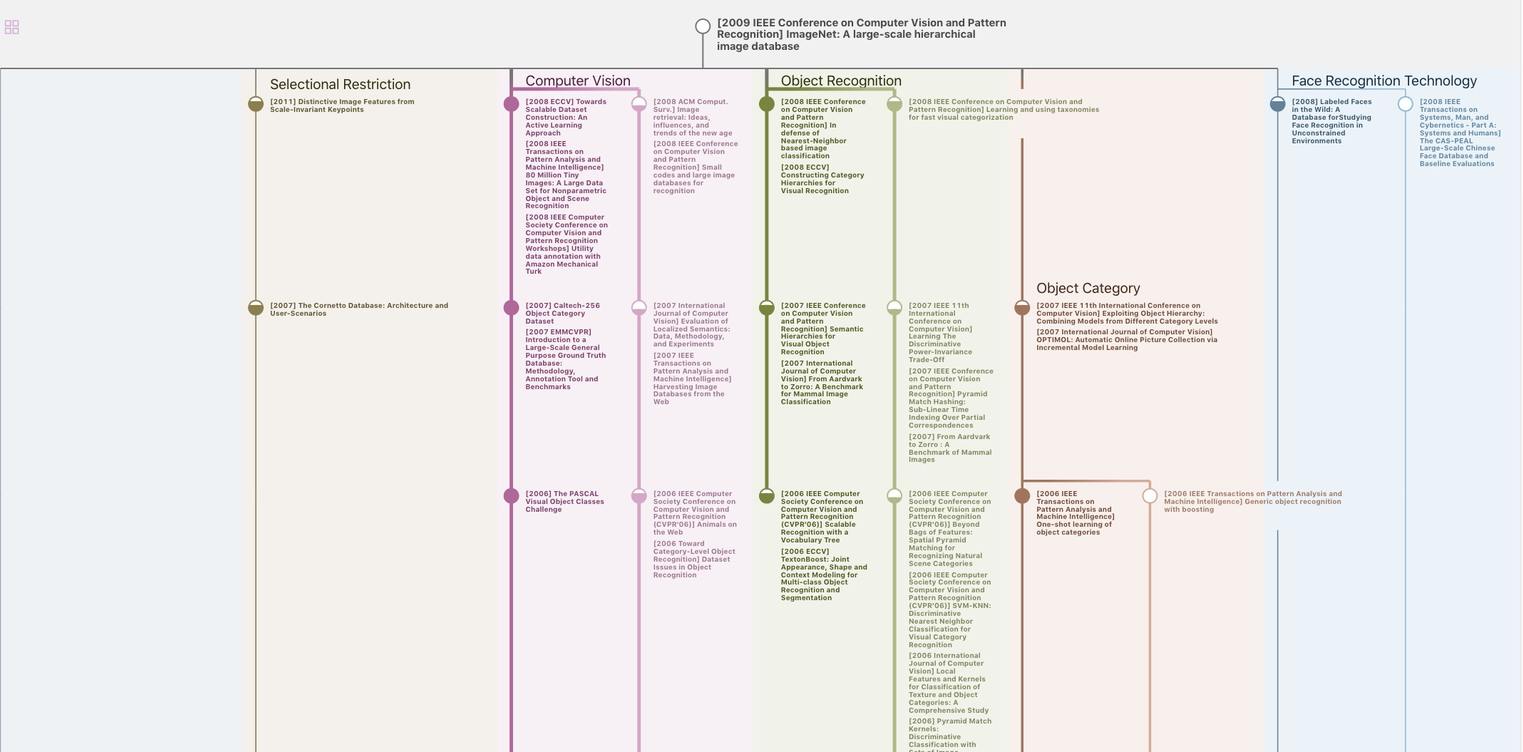
生成溯源树,研究论文发展脉络
Chat Paper
正在生成论文摘要