ProbMCL: Simple Probabilistic Contrastive Learning for Multi-label Visual Classification
arXiv (Cornell University)(2024)
摘要
Multi-label image classification presents a challenging task in many domains,including computer vision and medical imaging. Recent advancements haveintroduced graph-based and transformer-based methods to improve performance andcapture label dependencies. However, these methods often include complexmodules that entail heavy computation and lack interpretability. In this paper,we propose Probabilistic Multi-label Contrastive Learning (ProbMCL), a novelframework to address these challenges in multi-label image classificationtasks. Our simple yet effective approach employs supervised contrastivelearning, in which samples that share enough labels with an anchor image basedon a decision threshold are introduced as a positive set. This structurecaptures label dependencies by pulling positive pair embeddings together andpushing away negative samples that fall below the threshold. We enhancerepresentation learning by incorporating a mixture density network intocontrastive learning and generating Gaussian mixture distributions to explorethe epistemic uncertainty of the feature encoder. We validate the effectivenessof our framework through experimentation with datasets from the computer visionand medical imaging domains. Our method outperforms the existingstate-of-the-art methods while achieving a low computational footprint on bothdatasets. Visualization analyses also demonstrate that ProbMCL-learnedclassifiers maintain a meaningful semantic topology.
更多查看译文
关键词
Multi-label Visual Classification,Supervised Contrastive Learning,Gaussian Mixture Models
AI 理解论文
溯源树
样例
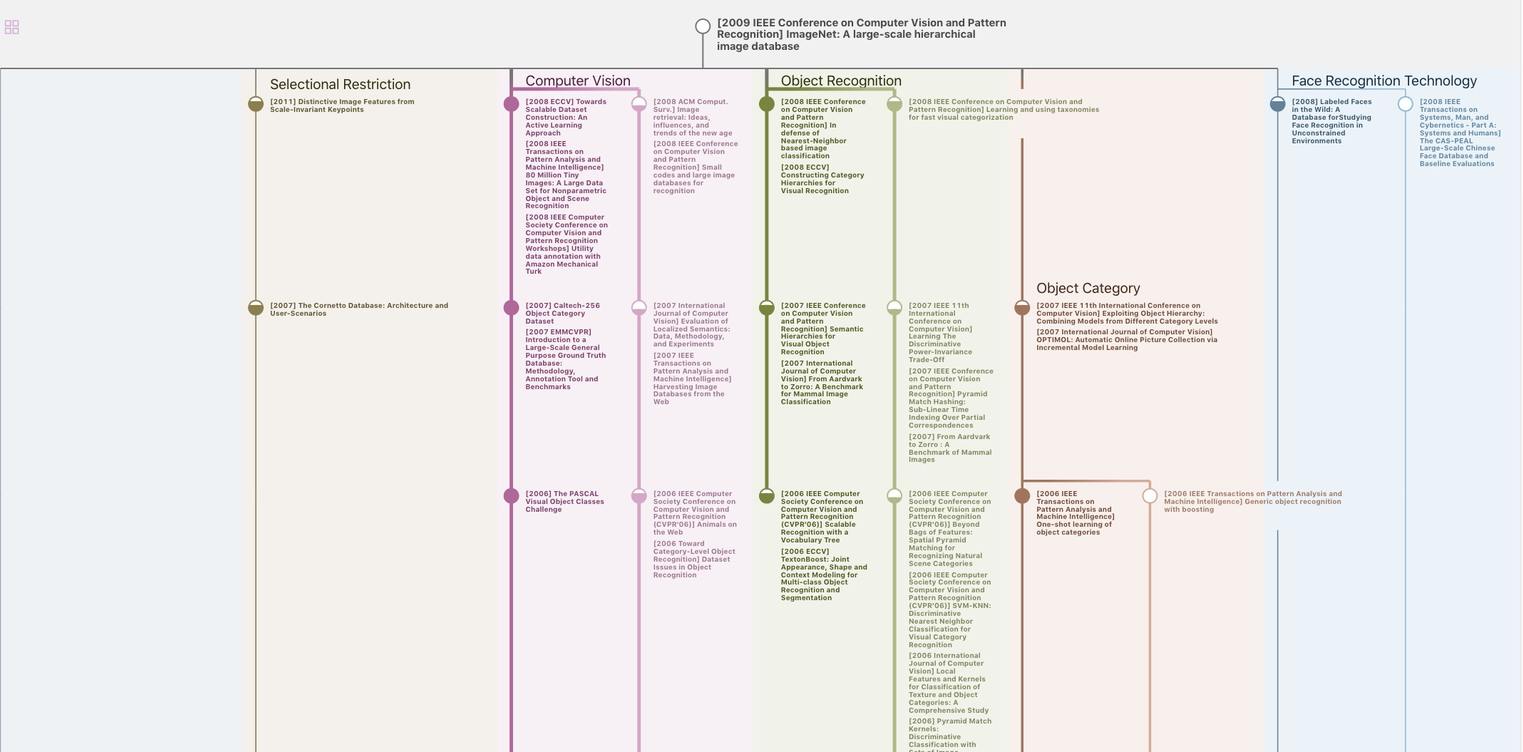
生成溯源树,研究论文发展脉络
Chat Paper
正在生成论文摘要