EPA: Neural Collapse Inspired Robust Out-of-Distribution Detector
CoRR(2024)
摘要
Out-of-distribution (OOD) detection plays a crucial role in ensuring the
security of neural networks. Existing works have leveraged the fact that
In-distribution (ID) samples form a subspace in the feature space, achieving
state-of-the-art (SOTA) performance. However, the comprehensive characteristics
of the ID subspace still leave under-explored. Recently, the discovery of
Neural Collapse (𝒩𝒞) sheds light on novel properties of the ID
subspace. Leveraging insight from 𝒩𝒞, we observe that the Principal
Angle between the features and the ID feature subspace forms a superior
representation for measuring the likelihood of OOD. Building upon this
observation, we propose a novel 𝒩𝒞-inspired OOD scoring function,
named Entropy-enhanced Principal Angle (EPA), which integrates both the global
characteristic of the ID subspace and its inner property. We experimentally
compare EPA with various SOTA approaches, validating its superior performance
and robustness across different network architectures and OOD datasets.
更多查看译文
关键词
Out-of-distribution detection,subspace principal angle,neural collapse
AI 理解论文
溯源树
样例
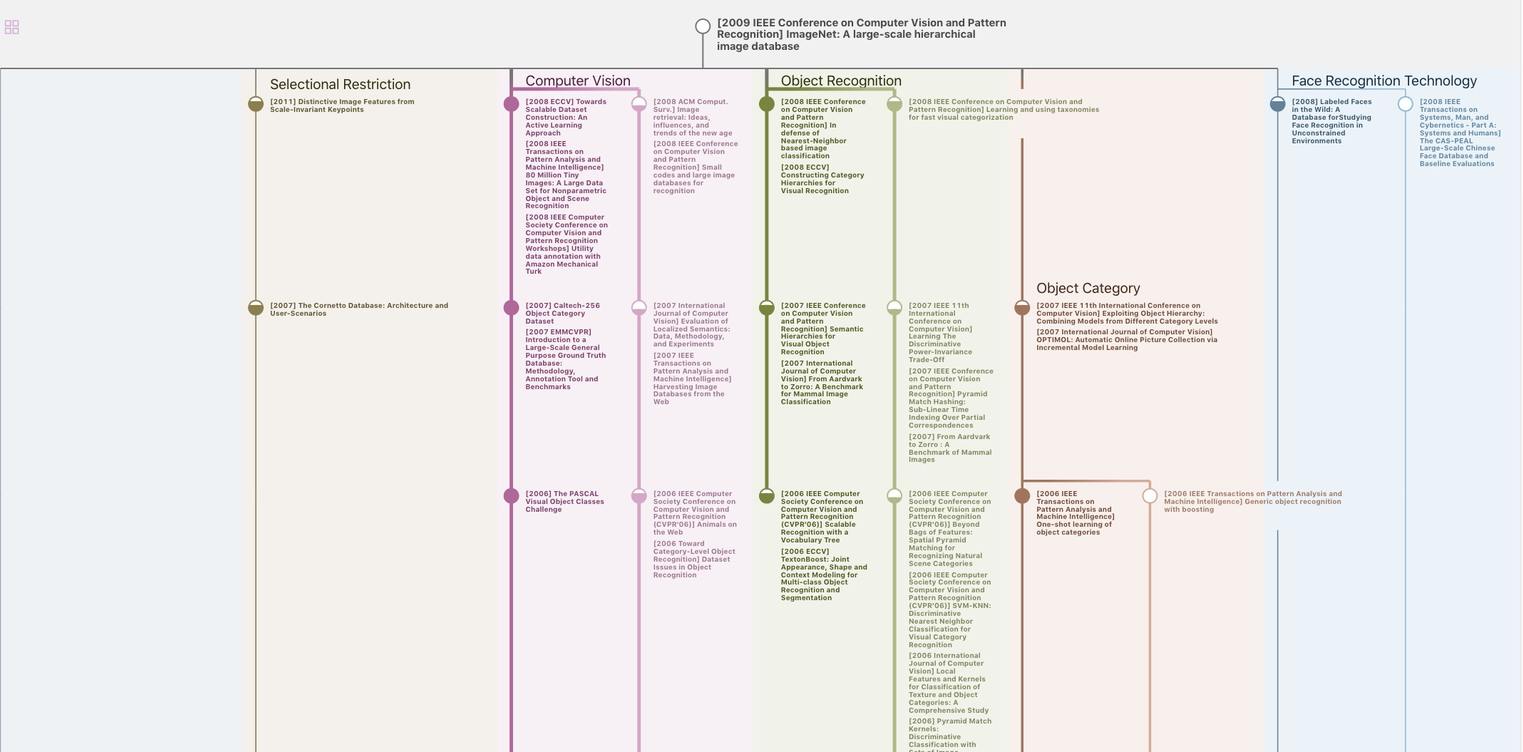
生成溯源树,研究论文发展脉络
Chat Paper
正在生成论文摘要