Fully Automated Explainable Abdominal CT Contrast Media Phase Classification Using Organ Segmentation and Machine Learning
medrxiv(2023)
摘要
Purpose To detect contrast media injection phase from CT images by means of organ segmentation and deep learning.
Materials and Methods A total number of 2509 CT images split into four subsets of non-contrast (class #0), arterial (class #1), venous (class #2), and delayed (class #3) after contrast media injection were collected from two CT scanners. Seven organs including the liver, spleen, heart, kidneys, lungs, urinary bladder, and aorta along with body contour masks were generated by pre-trained deep learning algorithms. Subsequently, five first-order statistical features including average, standard deviation, 10, 50, and 90 percentiles extracted from the above-mentioned masks were fed to machine learning models after feature selection and reduction to classify the CT images in one of four above mentioned classes. A ten-fold data split strategy was followed. The performance of our methodology was evaluated in terms of classification accuracy metrics.
Results The best performance was achieved by Boruta feature selection and RF model with average area under the curve of more than 0.999 and accuracy of 0.9936 averaged over four classes and ten folds. Boruta feature selection selected all predictor features. The lowest classification was observed for class #2 (0.9888), which is already an excellent result. In the ten-fold strategy, only 33 cases from 2509 cases (∼1.4%) were misclassified.
Conclusion We developed a fast, accurate, reliable, and explainable methodology to classify contrast media phases which may be useful in data curation and annotation in big online datasets or local datasets with non-standard or no series description.
Key points
1. The lack of standard series description and information about contrast media phase limits the usability of medical CT data.
2. We developed a twostep deep learning/machine learning solution with excellent performance.
3. This fast, automated, reliable and explainable purposed pipeline can tag every CT images with using only image matrices.
### Competing Interest Statement
The authors have declared no competing interest.
### Funding Statement
This work was supported by the Euratom research and training programme 2019-2020 Sinfonia project under grant agreement No 945196 and by the Distinguished Professor Program of Obuda University, Budapest Hungary.
### Author Declarations
I confirm all relevant ethical guidelines have been followed, and any necessary IRB and/or ethics committee approvals have been obtained.
Yes
The details of the IRB/oversight body that provided approval or exemption for the research described are given below:
The study was approved by the institutional ethics committee of HUG (CCER ID: 2017-00922) which allows us to process these images retrospectively.
I confirm that all necessary patient/participant consent has been obtained and the appropriate institutional forms have been archived, and that any patient/participant/sample identifiers included were not known to anyone (e.g., hospital staff, patients or participants themselves) outside the research group so cannot be used to identify individuals.
Yes
I understand that all clinical trials and any other prospective interventional studies must be registered with an ICMJE-approved registry, such as ClinicalTrials.gov. I confirm that any such study reported in the manuscript has been registered and the trial registration ID is provided (note: if posting a prospective study registered retrospectively, please provide a statement in the trial ID field explaining why the study was not registered in advance).
Yes
I have followed all appropriate research reporting guidelines, such as any relevant EQUATOR Network research reporting checklist(s) and other pertinent material, if applicable.
Yes
The dataset is not publicly available.
更多查看译文
AI 理解论文
溯源树
样例
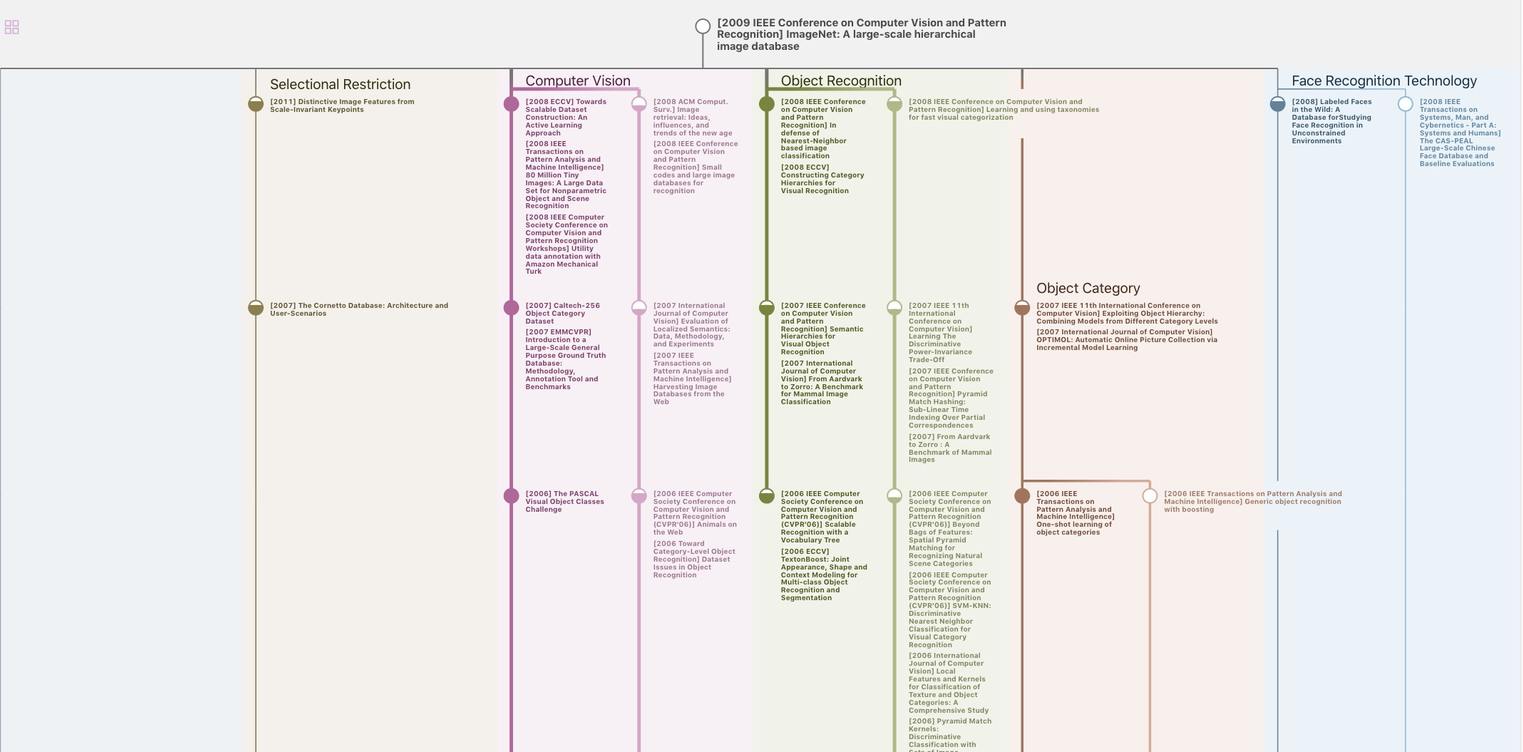
生成溯源树,研究论文发展脉络
Chat Paper
正在生成论文摘要